Parameter Space CNN for Cortical Surface Segmentation.
Bildverarbeitung für die Medizin(2020)
摘要
Spherical coordinate systems have become a standard for analyzing human cortical neuroimaging data. Surface-based signals, such as curvature, folding patterns, functional activations, or estimates of myelination define relevant cortical regions. Surface-based deep learning approaches, however, such as spherical CNNs primarily focus on classification and cannot yet achieve satisfactory accuracy in segmentation tasks. To perform surface-based segmentation of the human cortex, we introduce and evaluate a 2D parameter space approach with view aggregation (pCNN). We evaluate this network with respect to accuracy and show that it outperforms the spherical CNN by a margin, increasing the average Dice similarity score for cortical segmentation to above 0.9.
更多查看译文
AI 理解论文
溯源树
样例
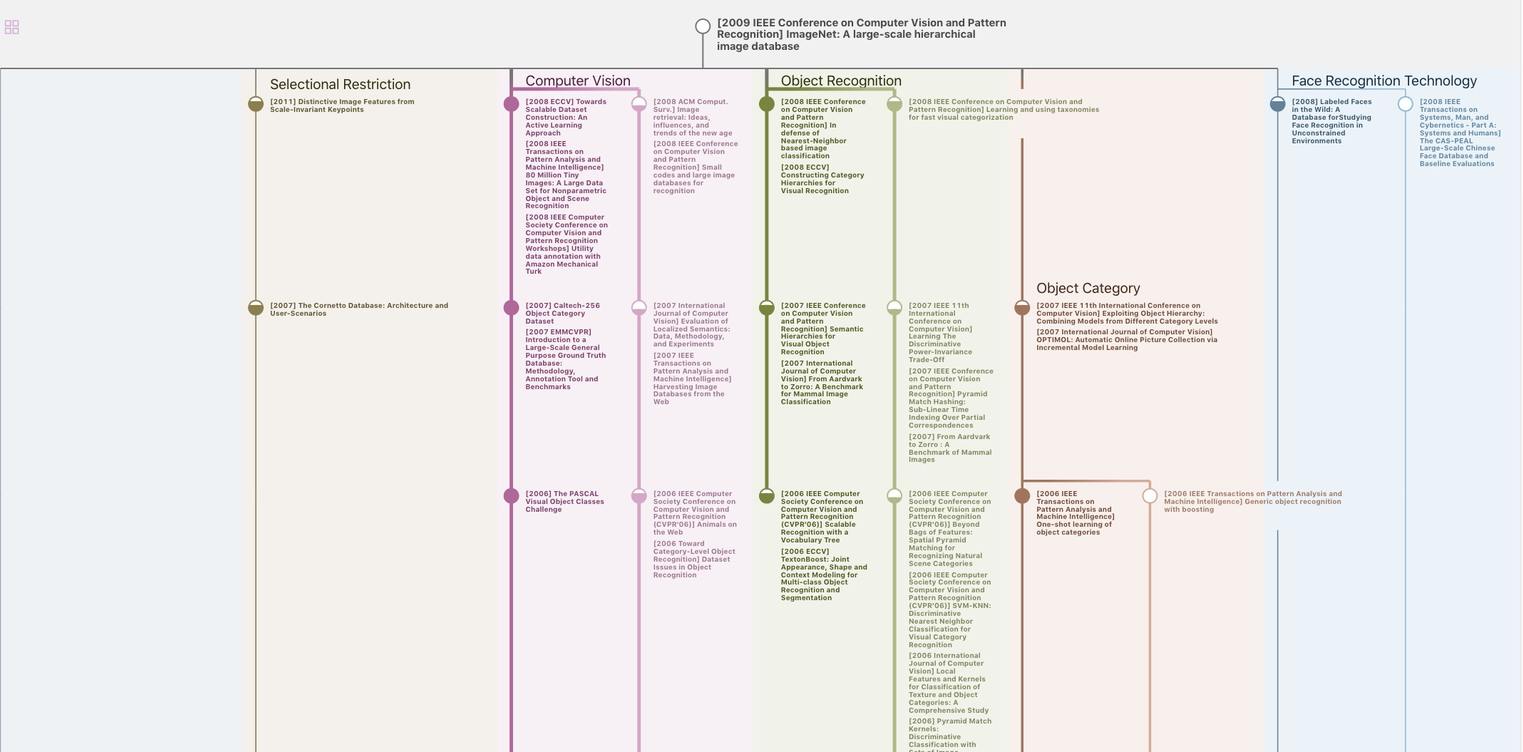
生成溯源树,研究论文发展脉络
Chat Paper
正在生成论文摘要