Summarizing The Performances Of A Background Subtraction Algorithm Measured On Several Videos
2020 IEEE INTERNATIONAL CONFERENCE ON IMAGE PROCESSING (ICIP)(2020)
摘要
There exist many background subtraction algorithms to detect motion in videos. To help comparing them, datasets with ground-truth data such as CDNET or LASIESTA have been proposed. These datasets organize videos in categories that represent typical challenges for background subtraction. The evaluation procedure promoted by their authors consists in measuring performance indicators for each video separately and to average them hierarchically, within a category first, then between categories, a procedure which we name "summarization". While the summarization by averaging performance indicators is a valuable effort to standardize the evaluation procedure, it has no theoretical justification and it breaks the intrinsic relationships between summarized indicators. This leads to interpretation inconsistencies. In this paper, we present a theoretical approach to summarize the performances for multiple videos that preserves the relationships between performance indicators. In addition, we give formulas and an algorithm to calculate summarized performances. Finally, we showcase our observations on CDNET 2014.
更多查看译文
关键词
performance summarization, background subtraction, multiple evaluations, CDNET, classification performance
AI 理解论文
溯源树
样例
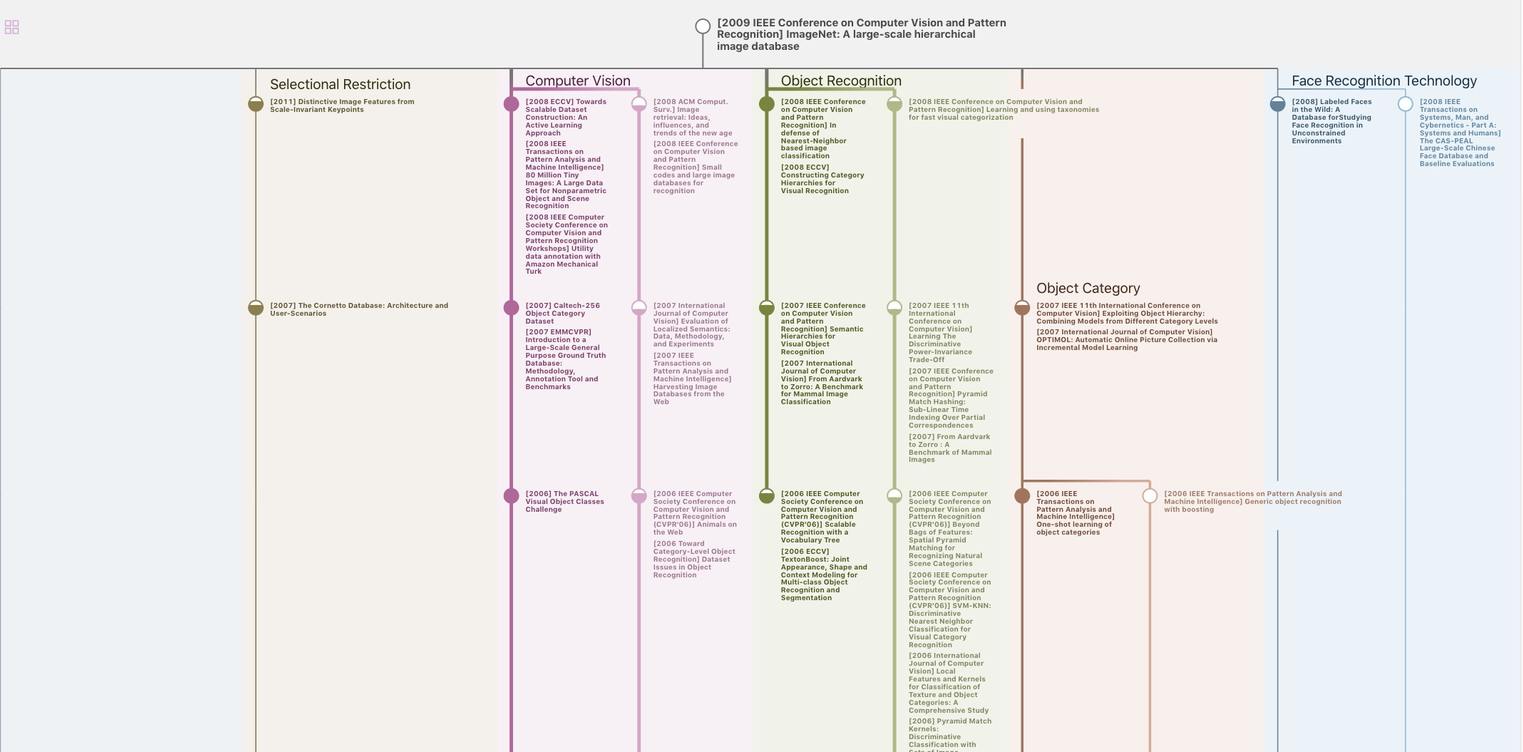
生成溯源树,研究论文发展脉络
Chat Paper
正在生成论文摘要