Multi-Vehicle Routing Problems with Soft Time Windows: A Multi-Agent Reinforcement Learning Approach
TRANSPORTATION RESEARCH PART C-EMERGING TECHNOLOGIES(2020)
摘要
Multi-vehicle routing problem with soft time windows (MVRPSTW) is an indispensable constituent in urban logistics distribution system. In the last decade, numerous methods for MVRPSTW have sprung up, but most of them are based on heuristic rules which require huge computation time. With the rapid increasing of logistics demand, traditional methods incur the dilemma of computation efficiency. To efficiently solve the problem, we propose a novel reinforcement learning algorithm named Multi-Agent Attention Model in this paper. Specifically, the vehicle routing problem is regarded as a vehicle tour generation process, and an encoder-decoder framework with attention layers is proposed to generate tours of multiple vehicles iteratively. Furthermore, a multi-agent reinforcement learning method with an unsupervised auxiliary network is developed for model training. By evaluated on three synthetic networks with different scale, the results demonstrate that the proposed method consistently outperforms traditional methods with little computation time. In addition, we validate the extensibility of the well-trained model by varying the number of customers and capacity of vehicles. Finally, the impact of parameters settings on the algorithmic performance are investigated.
更多查看译文
关键词
Reinforcement learning, Vehicle routing problem, Attention mechanism, Computational efficiency, Multi-agent
AI 理解论文
溯源树
样例
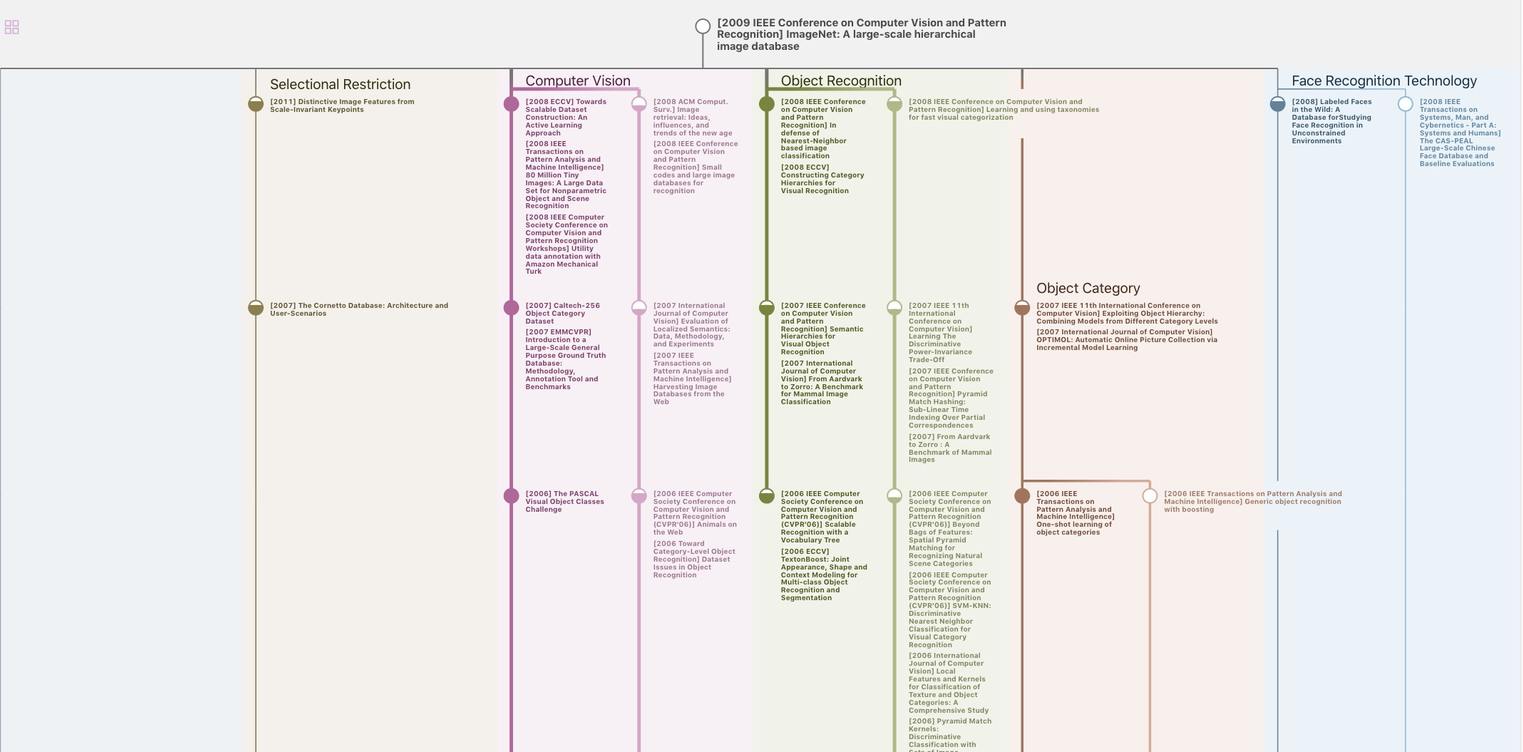
生成溯源树,研究论文发展脉络
Chat Paper
正在生成论文摘要