Using probe electrospray ionization mass spectrometry and machine learning for detecting pancreatic cancer with high performance.
AMERICAN JOURNAL OF TRANSLATIONAL RESEARCH(2020)
Abstract
A rapid blood-based diagnostic modality to detect pancreatic ductal adenocarcinoma (PDAC) with high accuracy is an unmet medical need. The study aimed to validate a unique diagnosis system using Probe Electrospray Ionization Mass Spectrometry (PESI-MS) and Machine Learning to the diagnosis of PDAC. Peripheral blood samples were collected from a total of 322 consecutive PDAC patients and 265 controls with a family history of PDAC. Five mu l of serum samples were analyzed using PESI MS system. The mass spectra from each specimen were then fed into machine learning algorithms to discriminate between control and cancer cases. A total of 587 serum samples were analyzed. The sensitivity of the machine learning algorithm using PESI-MS profiles to identify PDAC is 90.8% with specificity of 91.7% (95% CI 83.9%-97.4% and 82.8% 97.7% respectively). Combined PEST MS profiles with age and CA19-9 as predictors, the accuracy for stage 1 or 2 of PDAC is 92.9% and for stage 3 or 4 is 93% (95% CI 86.3-98.2; 87.9 97.4 respectively). The accuracy and simplicity of the PESI MS profiles combined with machine learning provide an opportunity to detect PDAC at an early stage and must be applicable to the examination of at risk populations.
MoreTranslated text
Key words
Probe electrospray ionization mass spectrometry (PESI-MS),pancreatic ductal adenocarcinoma (PDAC),machine learning
AI Read Science
Must-Reading Tree
Example
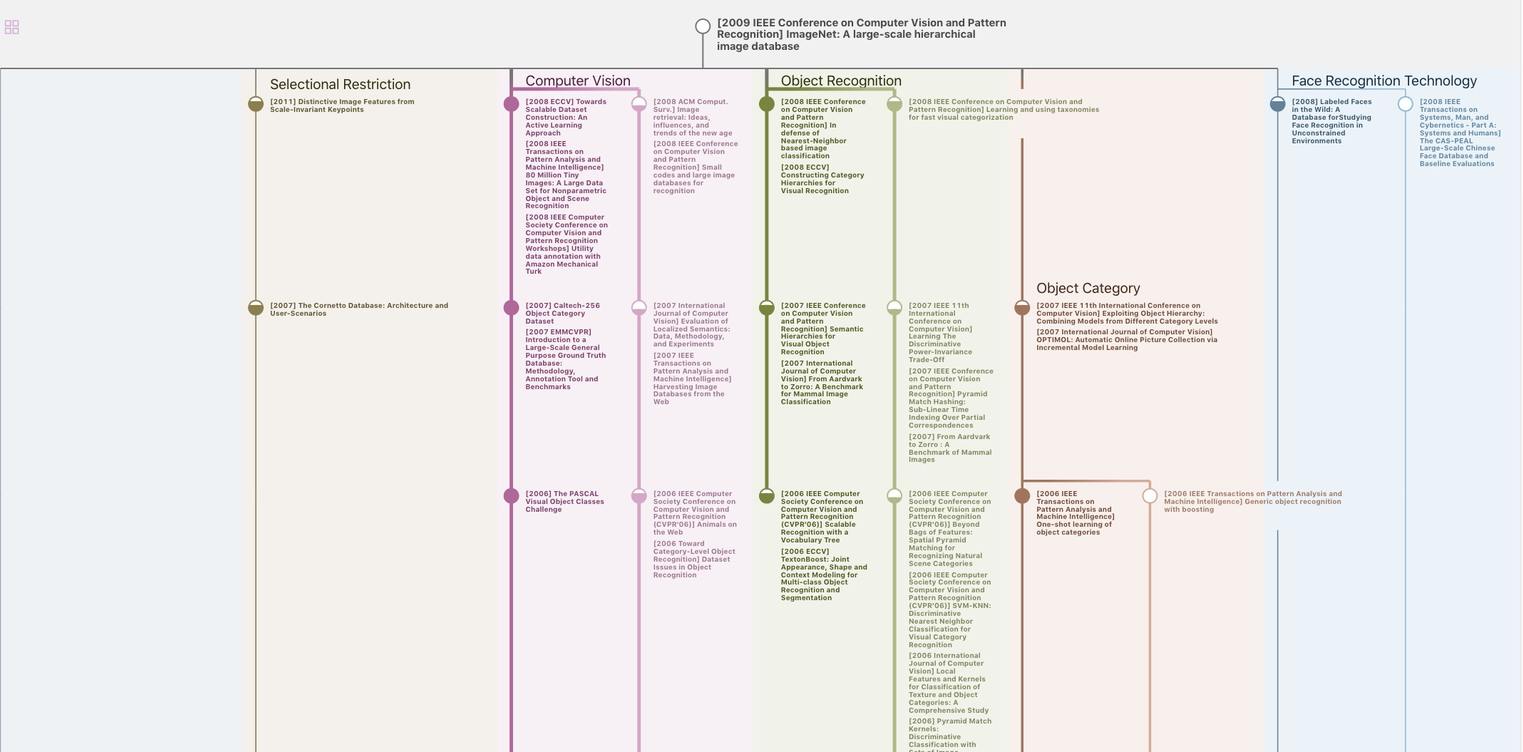
Generate MRT to find the research sequence of this paper
Chat Paper
Summary is being generated by the instructions you defined