Robust Visual Ship Tracking with an Ensemble Framework via Multi-View Learning and Wavelet Filter.
SENSORS(2020)
摘要
Maritime surveillance videos provide crucial on-spot kinematic traffic information (traffic volume, ship speeds, headings, etc.) for varied traffic participants (maritime regulation departments, ship crew, ship owners, etc.) which greatly benefits automated maritime situational awareness and maritime safety improvement. Conventional models heavily rely on visual ship features for the purpose of tracking ships from maritime image sequences which may contain arbitrary tracking oscillations. To address this issue, we propose an ensemble ship tracking framework with a multi-view learning algorithm and wavelet filter model. First, the proposed model samples ship candidates with a particle filter following the sequential importance sampling rule. Second, we propose a multi-view learning algorithm to obtain raw ship tracking results in two steps: extracting a group of distinct ship contour relevant features (i.e., Laplacian of Gaussian, local binary pattern, Gabor filter, histogram of oriented gradient, and canny descriptors) and learning high-level intrinsic ship features by jointly exploiting underlying relationships shared by each type of ship contour features. Third, with the help of the wavelet filter, we performed a data quality control procedure to identify abnormal oscillations in the ship positions which were further corrected to generate the final ship tracking results. We demonstrate the proposed ship tracker's performance on typical maritime traffic scenarios through four maritime surveillance videos.
更多查看译文
关键词
visual ship tracking,multi-view learning,wavelet filter,data quality control,smart ship
AI 理解论文
溯源树
样例
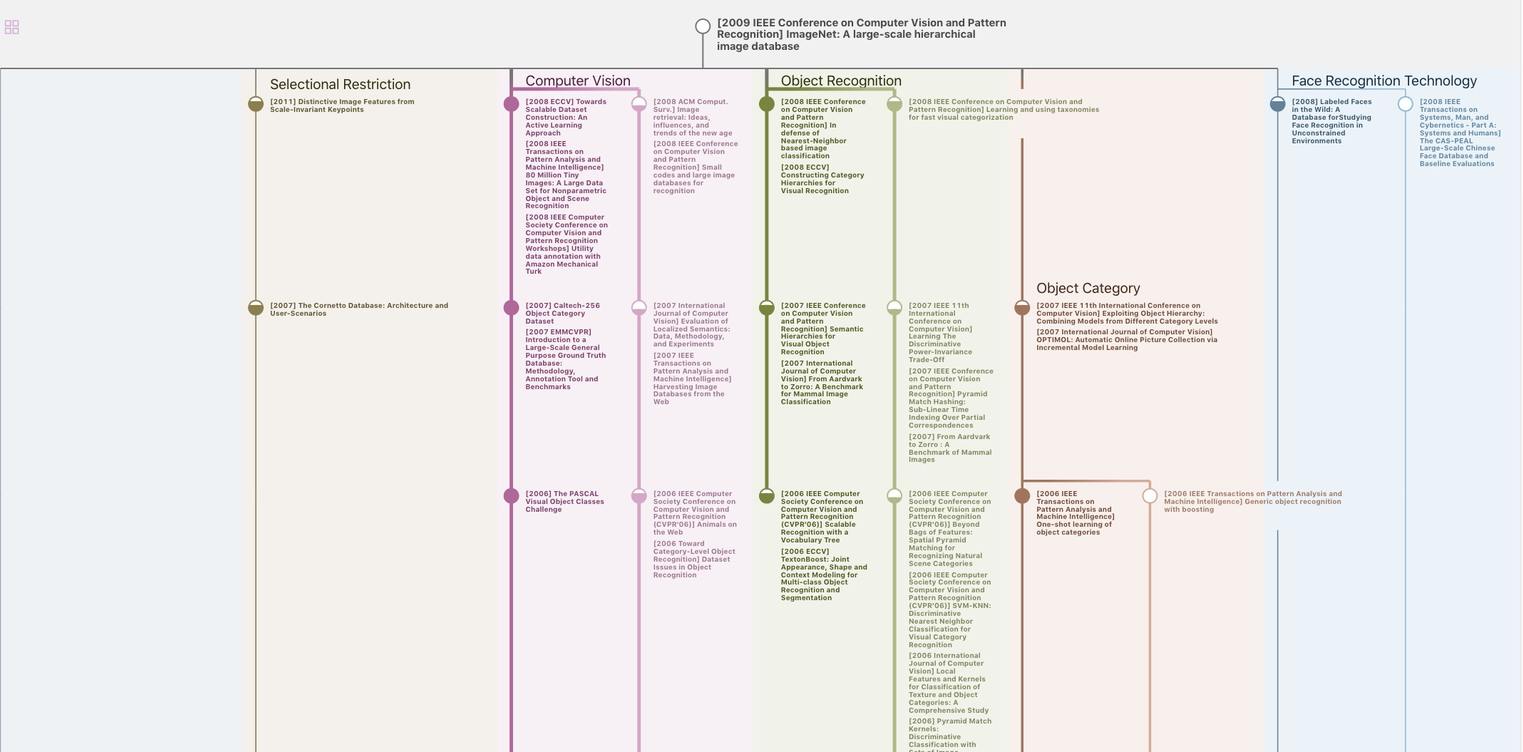
生成溯源树,研究论文发展脉络
Chat Paper
正在生成论文摘要