Towards Precise Intra-camera Supervised Person Re-Identification
arXiv (Cornell University)(2020)
Key words
Re-ID datasets,person reidentification,camera view,desirable Re-ID performance,intercamera labels,Re-ID problem,camera-specific nonparametric classifiers,hybrid mining quintuplet loss,Re-ID model updating step,intercamera learning module,intracamera learning,intracamera supervision,precise intracamera supervised person reidentification,intercamera identity association,graph-based ID association step
AI Read Science
Must-Reading Tree
Example
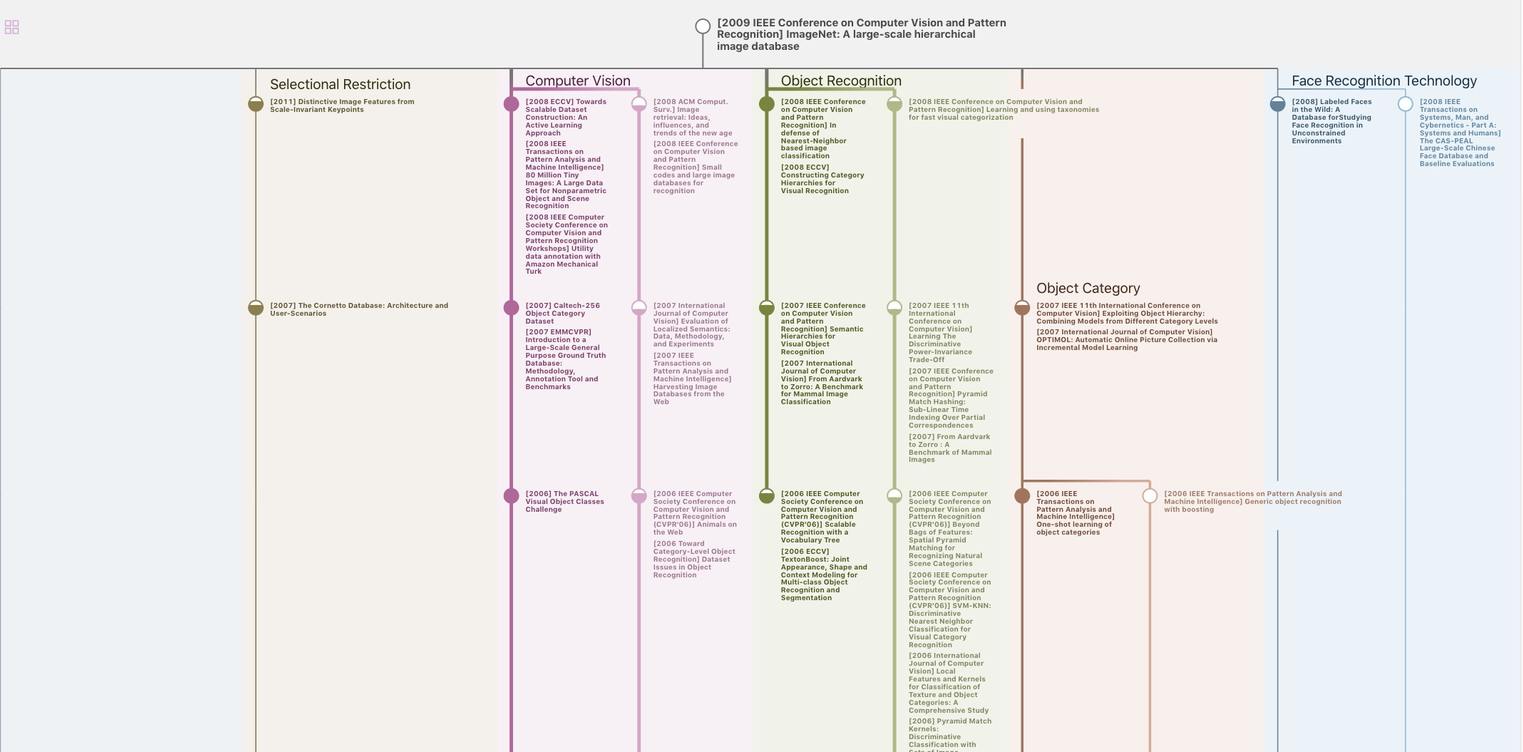
Generate MRT to find the research sequence of this paper
Chat Paper
Summary is being generated by the instructions you defined