Learning Spatio-Temporal Representations With Temporal Squeeze Pooling
2020 IEEE INTERNATIONAL CONFERENCE ON ACOUSTICS, SPEECH, AND SIGNAL PROCESSING(2020)
摘要
In this paper, we propose a new video representation learning method, named Temporal Squeeze (TS) pooling, which can extract the essential movement information from a long sequence of video frames and map it into a set of few images, named Squeezed Images. By embedding the Temporal Squeeze pooling as a layer into off-the-shelf Convolution Neural Networks (CNN), we design a new video classification model, named Temporal Squeeze Network (TeSNet). The resulting Squeezed Images contain the essential movement information from the video frames, corresponding to the optimization of the video classification task. We evaluate our architecture on two video classification benchmarks, and the results achieved are compared to the state-of-the-art.
更多查看译文
关键词
Convolution Neural Networks (CNN), Temporal Squeeze pooling, video representation
AI 理解论文
溯源树
样例
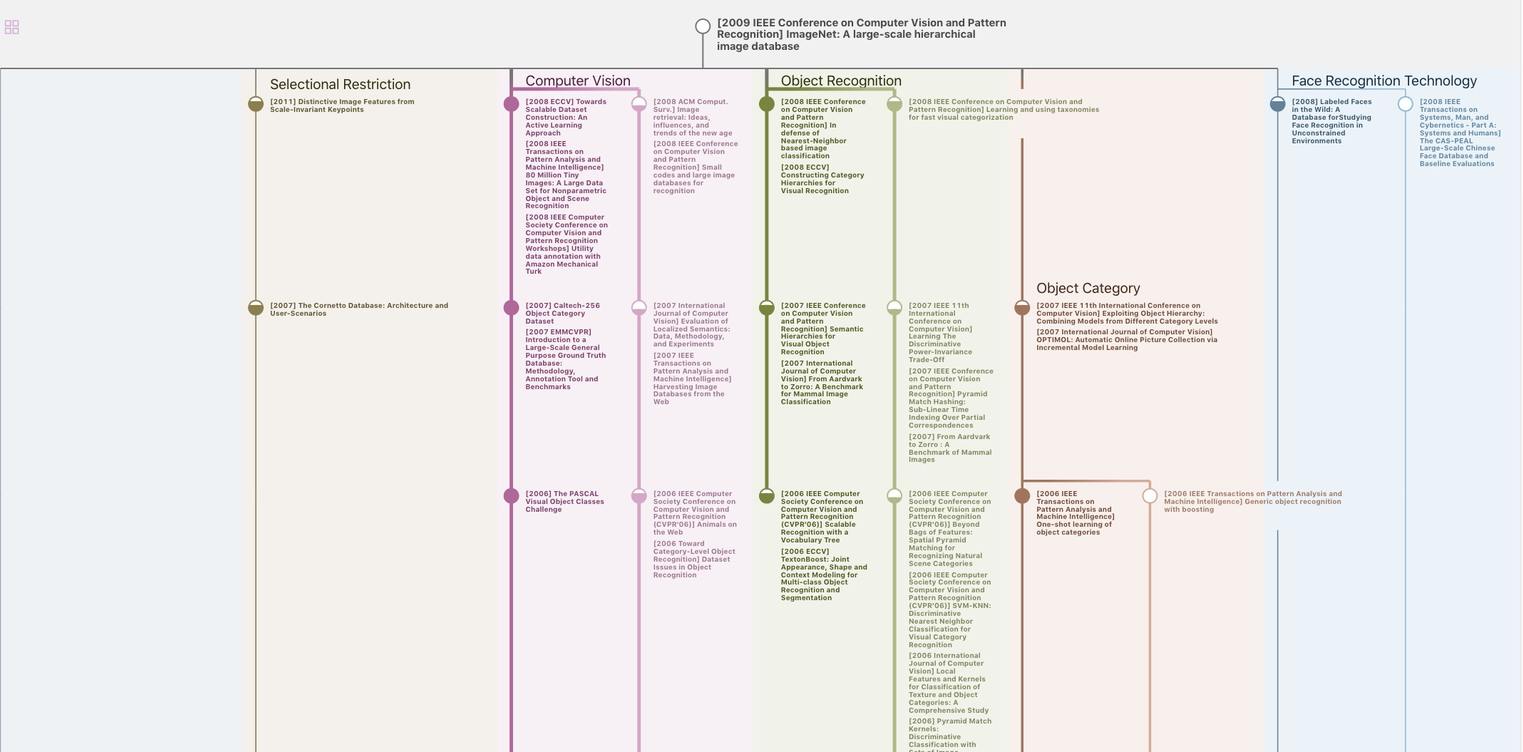
生成溯源树,研究论文发展脉络
Chat Paper
正在生成论文摘要