Finding Novelty With Uncertainty
MEDICAL IMAGING 2020: IMAGE PROCESSING(2021)
摘要
Medical images are often used to detect and characterize pathology and disease; however, automatically identifying and segmenting pathology in medical images is challenging because the appearance of pathology across diseases varies widely. To address this challenge, we propose a Bayesian deep learning method that learns to translate healthy computed tomography images to magnetic resonance images and simultaneously calculates voxel-wise uncertainty. Since high uncertainty occurs in pathological regions of the image, this uncertainty can be used for unsupervised anomaly segmentation. We show encouraging experimental results on an unsupervised anomaly segmentation task by combining two types of uncertainty into a novel quantity we call scibilic uncertainty.
更多查看译文
关键词
Unsupervised anomaly segmentation, image translation, uncertainty quantification
AI 理解论文
溯源树
样例
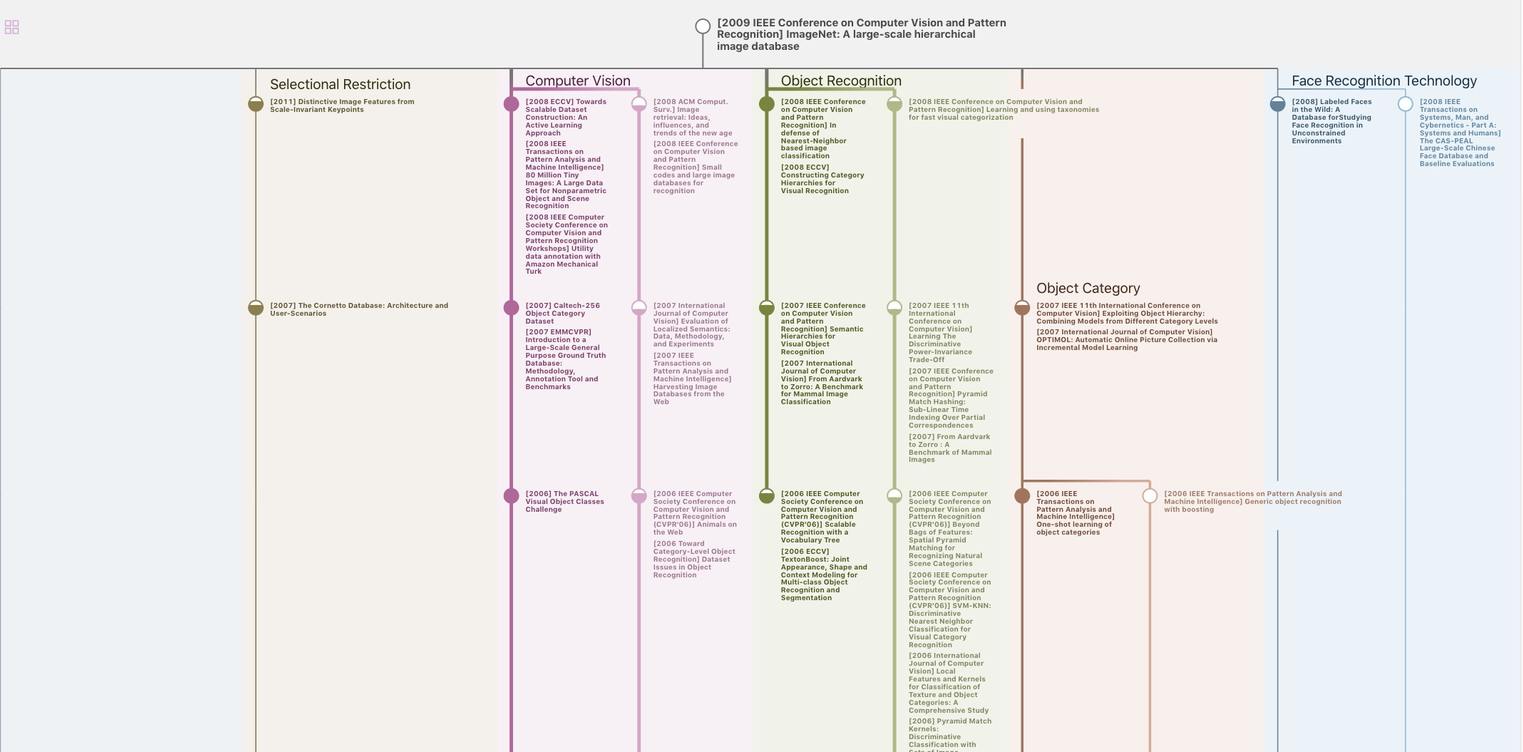
生成溯源树,研究论文发展脉络
Chat Paper
正在生成论文摘要