Leveraging Rationales to Improve Human Task Performance
PROCEEDINGS OF THE 25TH INTERNATIONAL CONFERENCE ON INTELLIGENT USER INTERFACES, IUI 2020(2020)
Abstract
Machine learning (ML) systems across many application areas are increasingly demonstrating performance that is beyond that of humans. In response to the proliferation of such models, the field of Explainable AI (XAI) has sought to develop techniques that enhance the transparency and interpretability of machine learning methods. In this work, we consider a question not previously explored within the XAI and ML communities: Given a computational system whose performance exceeds that of its human user, can explainable AI capabilities be leveraged to improve the performance of the human? We study this question in the context of the game of Chess, for which computational game engines that surpass the performance of the average player are widely available. We introduce the Rationale-Generating Algorithm, an automated technique for generating rationales for utility-based computational methods, which we evaluate with a multi-day user study against two baselines. The results show that our approach produces rationales that lead to statistically significant improvement in human task performance, demonstrating that rationales automatically generated from an AI's internal task model can be used not only to explain what the system is doing, but also to instruct the user and ultimately improve their task performance.
MoreTranslated text
Key words
Explainable AI,Machine Learning
AI Read Science
Must-Reading Tree
Example
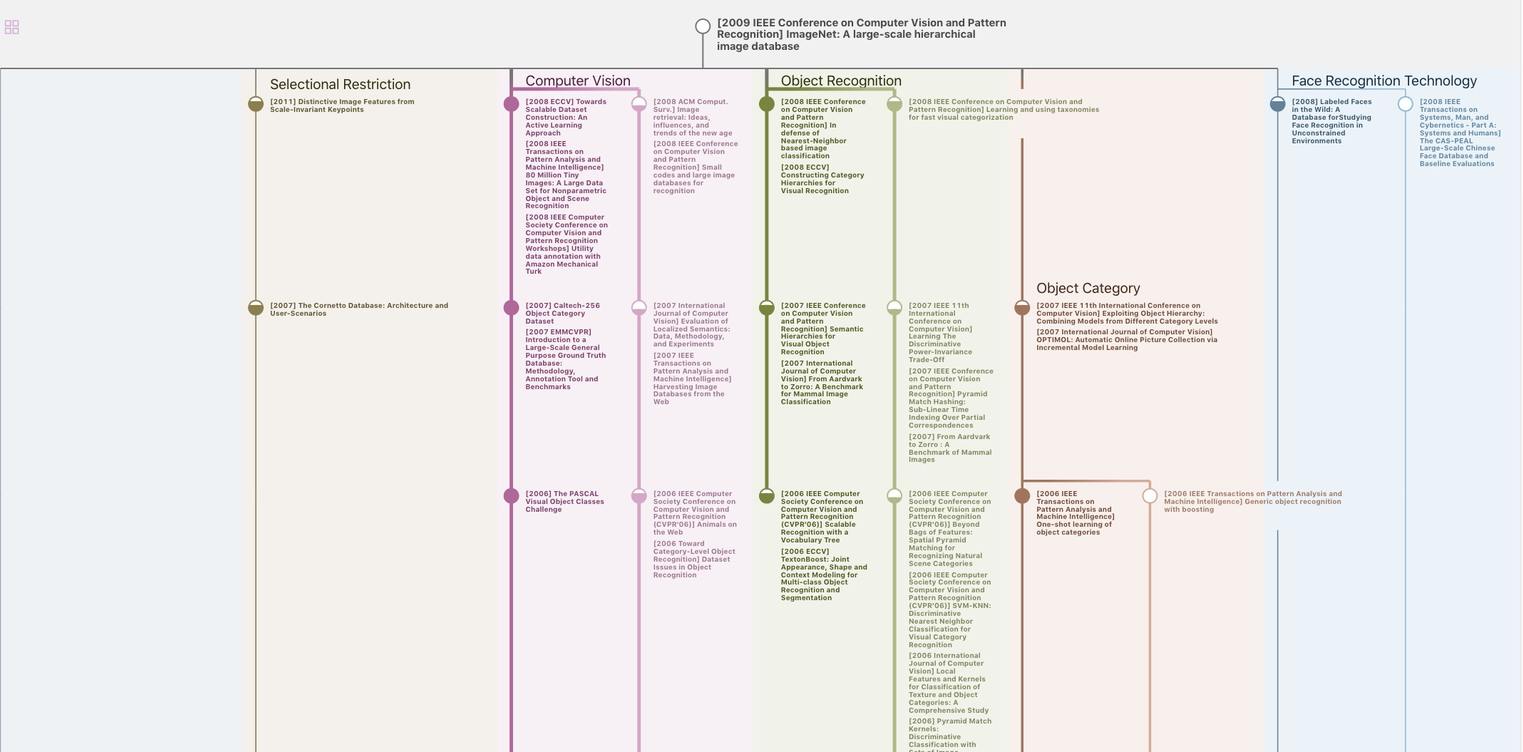
Generate MRT to find the research sequence of this paper
Chat Paper
Summary is being generated by the instructions you defined