Infinity Learning: Learning Markov Chains From Aggregate Steady-State Observations
THIRTY-FOURTH AAAI CONFERENCE ON ARTIFICIAL INTELLIGENCE, THE THIRTY-SECOND INNOVATIVE APPLICATIONS OF ARTIFICIAL INTELLIGENCE CONFERENCE AND THE TENTH AAAI SYMPOSIUM ON EDUCATIONAL ADVANCES IN ARTIFICIAL INTELLIGENCE(2020)
摘要
We consider the task of learning a parametric Continuous Tune Markov Chain (CTMC) sequence model without examples of sequences, where the training data consists entirely of aggregate steady-state statistics. Making the problem harder, we assume that the states we wish to predict are unobserved in the training data. Specifically, given a parametric model over the transition rates of a CTMC and some known transition rates, we wish to extrapolate its steady state distribution to states that are unobserved. A technical roadblock to learn a CTMC from its steady state has been that the chain rule to compute gradients will not work over the arbitrarily long sequences necessary to reach steady state -from where the aggregate statistics are sampled. To overcome this optimization challenge, we propose infinity-SGD, a principled stochastic gradient descent method that uses randomly-stopped estimators to avoid infinite sums required by the steady state computation, while learning even when only a subset of the CTMC states can be observed. We apply infinity-SGD to a real-world testbed and synthetic experiments showcasing its accuracy, ability to extrapolate the steady state distribution to unobserved states under unobserved conditions (heavy loads, when training under light loads), and succeeding in difficult scenarios where even a tailor-made extension of existing methods fails.
更多查看译文
关键词
infinity learning,learning markov chains,markov chains,steady-state
AI 理解论文
溯源树
样例
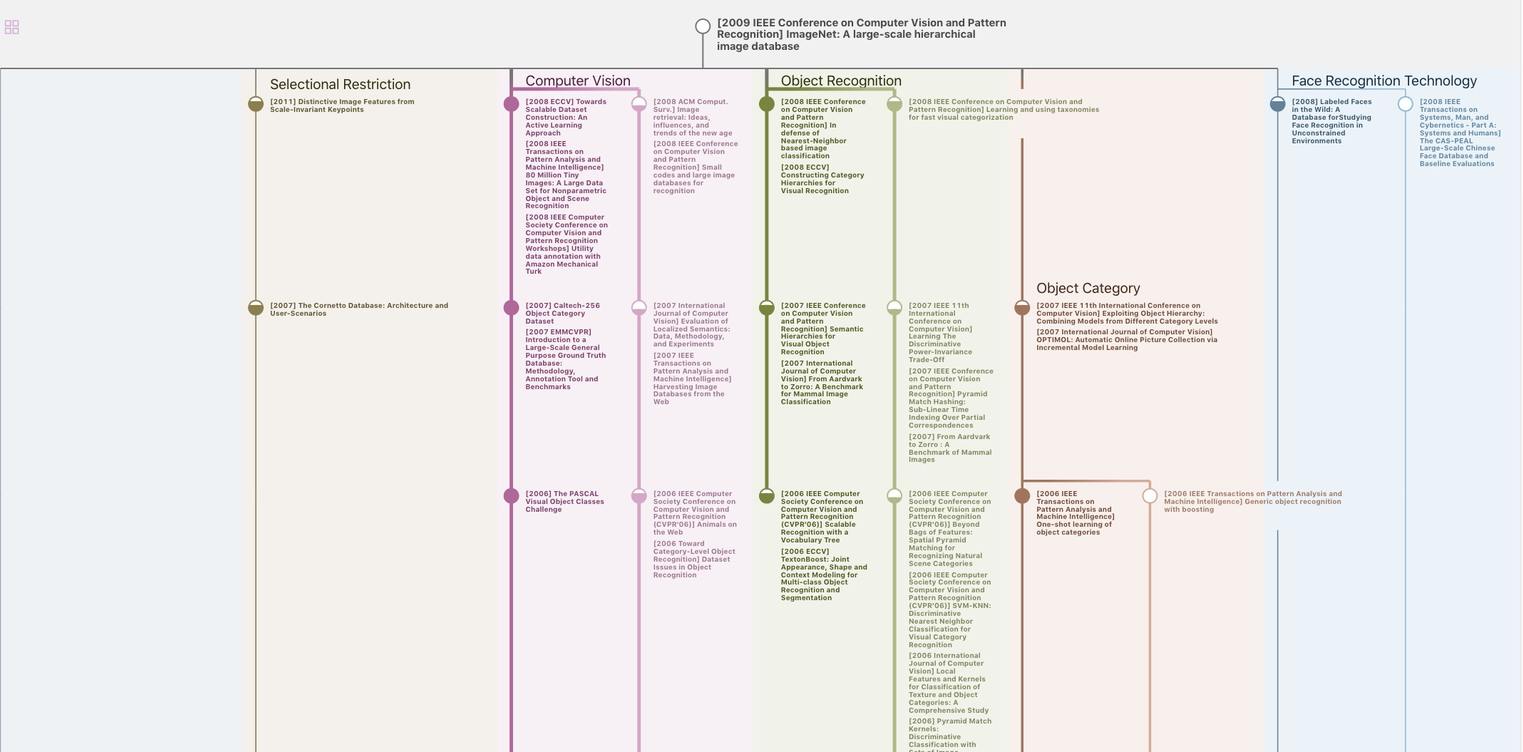
生成溯源树,研究论文发展脉络
Chat Paper
正在生成论文摘要