Turbo-Aggregate: Breaking the Quadratic Aggregation Barrier in Secure Federated Learning
IEEE Journal on Selected Areas in Information Theory(2021)
摘要
Federated learning is a distributed framework for training machine learning models over the data residing at mobile devices, while protecting the privacy of individual users. A major bottleneck in scaling federated learning to a large number of users is the overhead of secure model aggregation across many users. In particular, the overhead of the state-of-the-art protocols for secure model aggregation grows quadratically with the number of users. In this article, we propose the first secure aggregation framework, named Turbo-Aggregate, that in a network with N users achieves a secure aggregation overhead of O(NlogN), as opposed to O(N
2
), while tolerating up to a user dropout rate of 50%. Turbo-Aggregate employs a multi-group circular strategy for efficient model aggregation, and leverages additive secret sharing and novel coding techniques for injecting aggregation redundancy in order to handle user dropouts while guaranteeing user privacy. We experimentally demonstrate that Turbo-Aggregate achieves a total running time that grows almost linear in the number of users, and provides up to 40× speedup over the state-of-the-art protocols with up to N=200 users. Our experiments also demonstrate the impact of model size and bandwidth on the performance of Turbo-Aggregate.
更多查看译文
关键词
Federated learning,privacy-preserving machine learning,secure aggregation
AI 理解论文
溯源树
样例
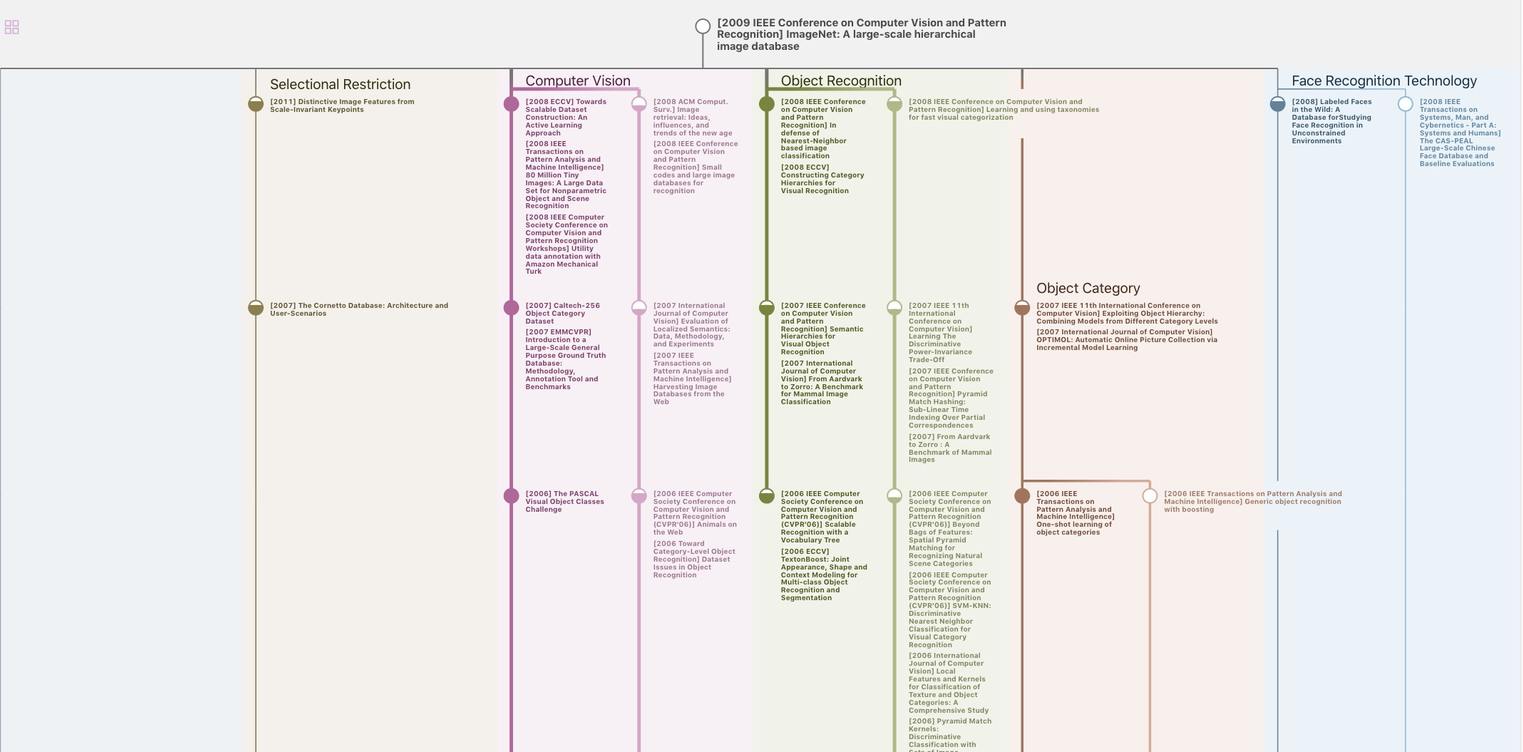
生成溯源树,研究论文发展脉络
Chat Paper
正在生成论文摘要