Improving Small-Scale Pedestrian Detection Using Informed Context
VCIP(2019)
摘要
Finding small objects is fundamentally challenging because there is little signal on the object to exploit. For the small-scale pedestrian detection, one must use image evidence beyond the pedestrian extent, which is often formulated as context. Unlike existing object detection methods that use adjacent regions or whole image as the context simply, we focus on more informed contexts exploiting and utilizing to improve small-scale pedestrian detection: firstly, one relationship network is developed to utilize the correlation among pedestrian instances in one image; secondly, two spatial regions, overhead area and feet bottom area, are taken as spatial context to exploit the relevance between pedestrian and scenes; at last, GRU [7] (Gated Recurrent Units) modules are introduced to take encoded contexts as input to guide the feature selection and fusion of each proposal. Instead of getting all of the outputs at once, we also iterate twice to refine the detection incrementally. Comprehensive experiments on Caltech Pedestrian [8] and SJTU-SPID [9] datasets, indicate that, with more informed context, the detection performance can be improved significantly, especially for the small-scale pedestrians.
更多查看译文
关键词
context,relation,pedestrian detection,GRU
AI 理解论文
溯源树
样例
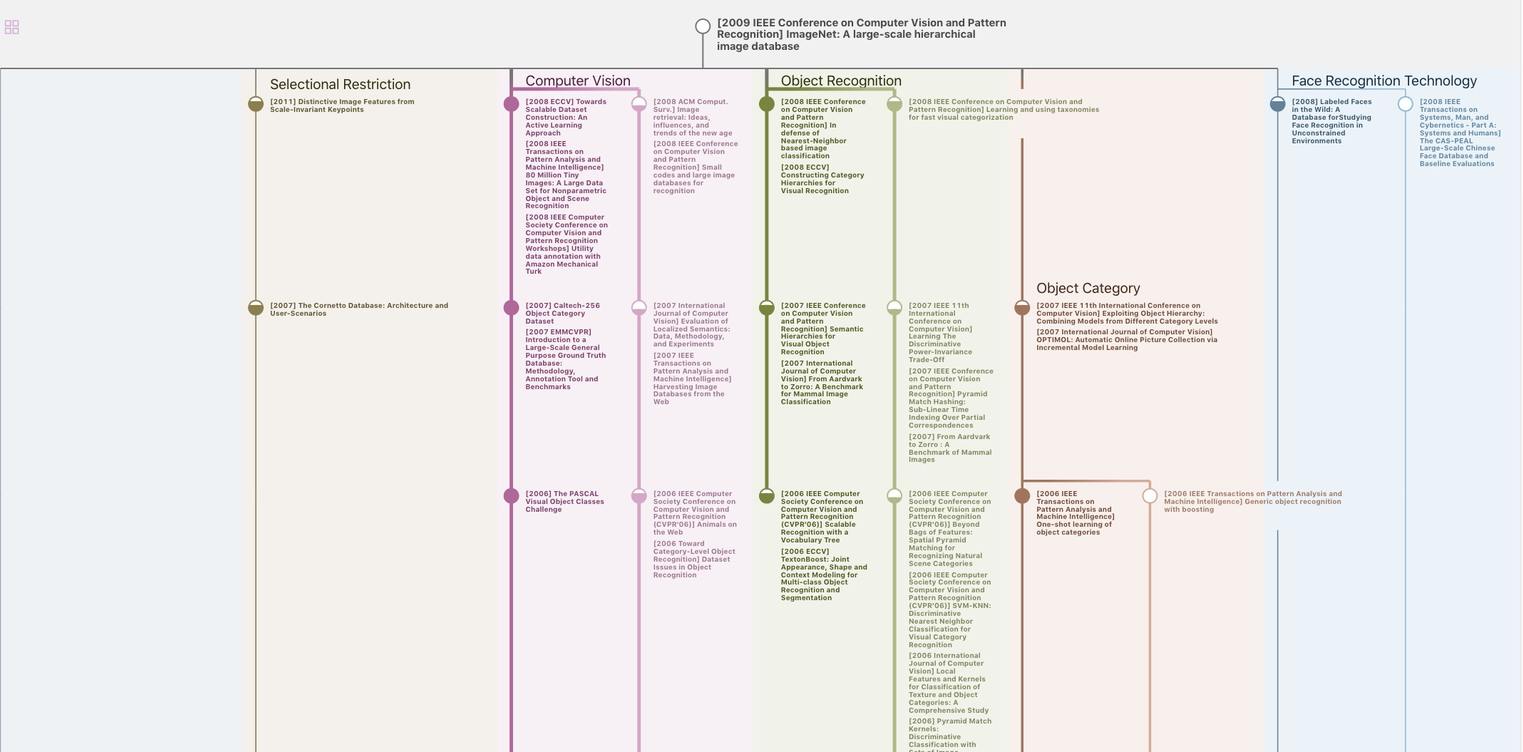
生成溯源树,研究论文发展脉络
Chat Paper
正在生成论文摘要