MuscleSense - Exploring Weight Sensing using Wearable Surface Electromyography (sEMG).
TEI '20: Fourteenth International Conference on Tangible, Embedded, and Embodied Interaction Sydney NSW Australia February, 2020(2020)
摘要
Strength training improves overall health, well-being, physical appearance, and sports performance.There are four major factors that affect training efficacy in a training session: exercise type, number of repetitions, movement velocity, and workload. Prior research has used wearable sensors to detect exercise type, number of repetitions, and movement velocity while training. However, detecting workload remains constrained to instrumented exercise equipment, such as smart exercise machines or RFID-tagged free weights.This paper presents MuscleSense, an approach that estimates exercise workload by using wearable Surface Electromyography (sEMG) sensors and regression analysis. We evaluated the accuracy of several regression models and the effects of sensor placement through a 20-person user study. Results showed that MuscleSense achieved an accuracy of 0.68kg (root mean square error, RMSE) in sensing workload using both forearm and arm sensors and support vector regression (SVR).
更多查看译文
关键词
Biosensing,Fitness,Health,Sports,Strength training,Wearables,Machine learning,Electromyography (EMG)
AI 理解论文
溯源树
样例
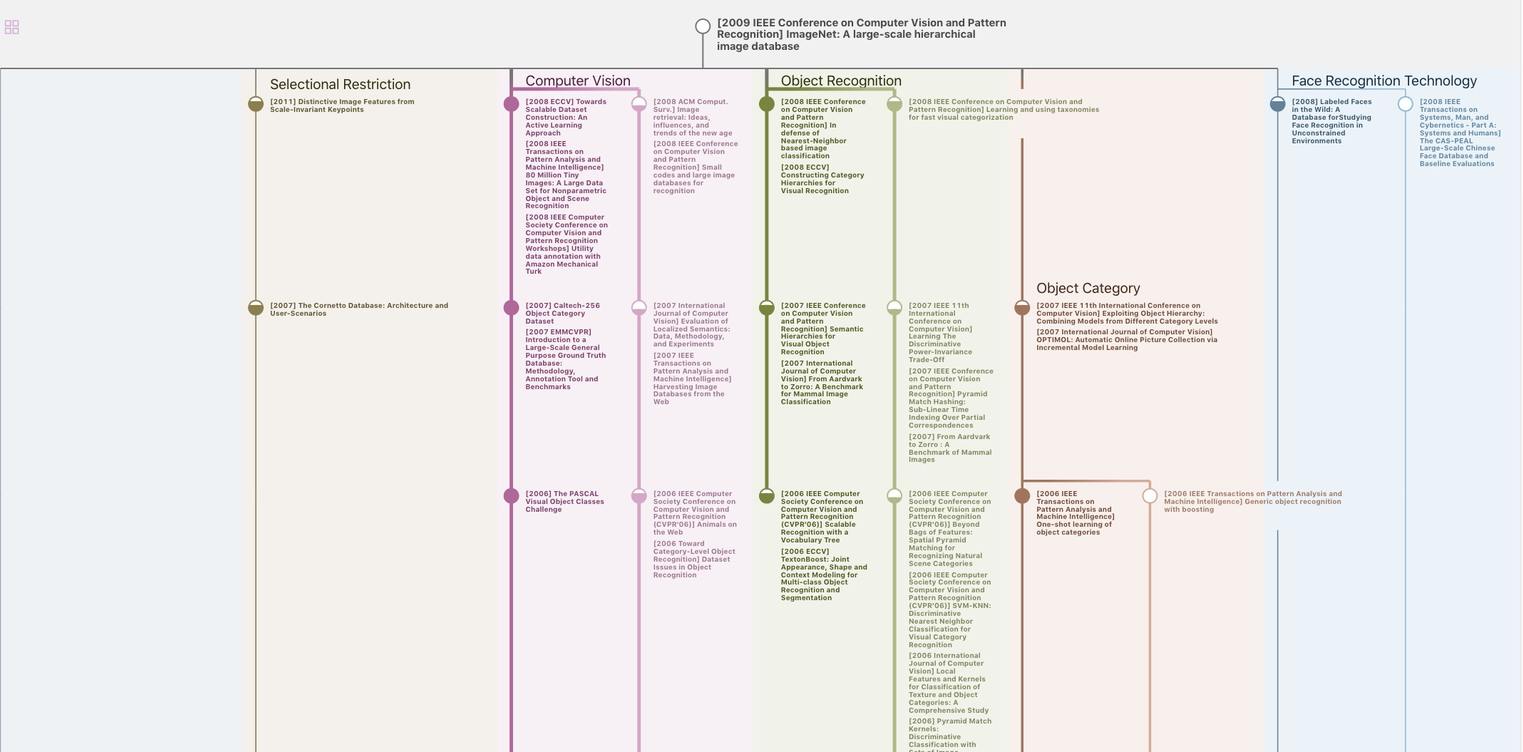
生成溯源树,研究论文发展脉络
Chat Paper
正在生成论文摘要