Detection of Maize Tassels from UAV RGB Imagery with Faster R-CNN.
REMOTE SENSING(2020)
摘要
Maize tassels play a critical role in plant growth and yield. Extensive RGB images obtained using unmanned aerial vehicle (UAV) and the prevalence of deep learning provide a chance to improve the accuracy of detecting maize tassels. We used images from UAV, a mobile phone, and the Maize Tassel Counting dataset (MTC) to test the performance of faster region-based convolutional neural network (Faster R-CNN) with residual neural network (ResNet) and a visual geometry group neural network (VGGNet). The results showed that the ResNet, as the feature extraction network, was better than the VGGNet for detecting maize tassels from UAV images with 600 x 600 resolution. The prediction accuracy ranged from 87.94% to 94.99%. However, the prediction accuracy was less than 87.27% from the UAV images with 5280 x 2970 resolution. We modified the anchor size to [85(2), 128(2), 256(2)] in the region proposal network according to the width and height of pixel distribution to improve detection accuracy up to 89.96%. The accuracy reached up to 95.95% for mobile phone images. Then, we compared our trained model with TasselNet without training their datasets. The average difference of tassel number was 1.4 between the calculations with 40 images for the two methods. In the future, we could further improve the performance of the models by enlarging datasets and calculating other tassel traits such as the length, width, diameter, perimeter, and the branch number of the maize tassels.
更多查看译文
关键词
convolutional neural networks,maize tassel,Faster R-CNN,UAV,image analysis
AI 理解论文
溯源树
样例
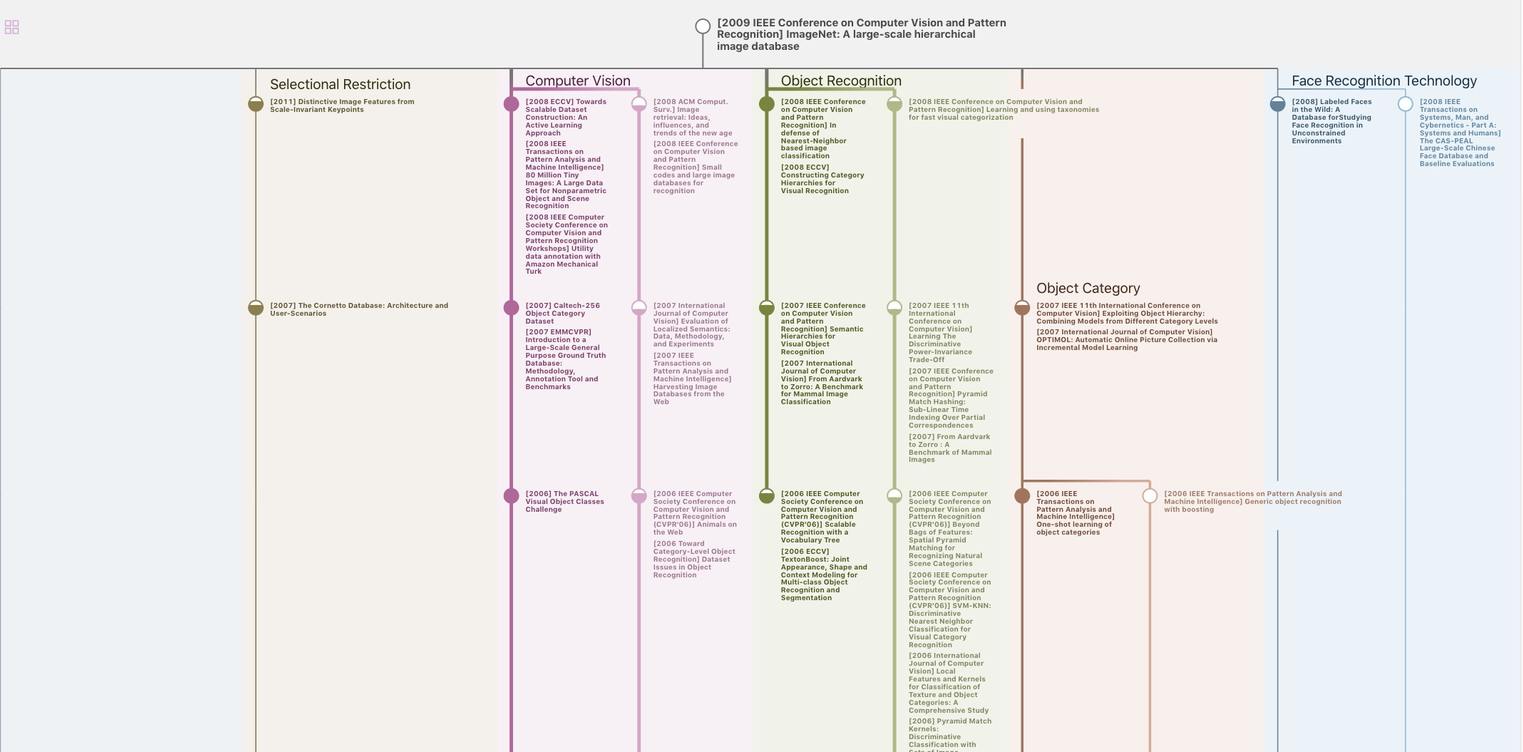
生成溯源树,研究论文发展脉络
Chat Paper
正在生成论文摘要