Transfer Heterogeneous Knowledge Among Peer-to-Peer Teammates: A Model Distillation Approach
arxiv(2020)
摘要
Peer-to-peer knowledge transfer in distributed environments has emerged as a promising method since it could accelerate learning and improve team-wide performance without relying on pre-trained teachers in deep reinforcement learning. However, for traditional peer-to-peer methods such as action advising, they have encountered difficulties in how to efficiently expressed knowledge and advice. As a result, we propose a brand new solution to reuse experiences and transfer value functions among multiple students via model distillation. But it is still challenging to transfer Q-function directly since it is unstable and not bounded. To address this issue confronted with existing works, we adopt Categorical Deep Q-Network. We also describe how to design an efficient communication protocol to exploit heterogeneous knowledge among multiple distributed agents. Our proposed framework, namely Learning and Teaching Categorical Reinforcement (LTCR), shows promising performance on stabilizing and accelerating learning progress with improved team-wide reward in four typical experimental environments.
更多查看译文
关键词
heterogeneous knowledge,model distillation approach,peer-to-peer
AI 理解论文
溯源树
样例
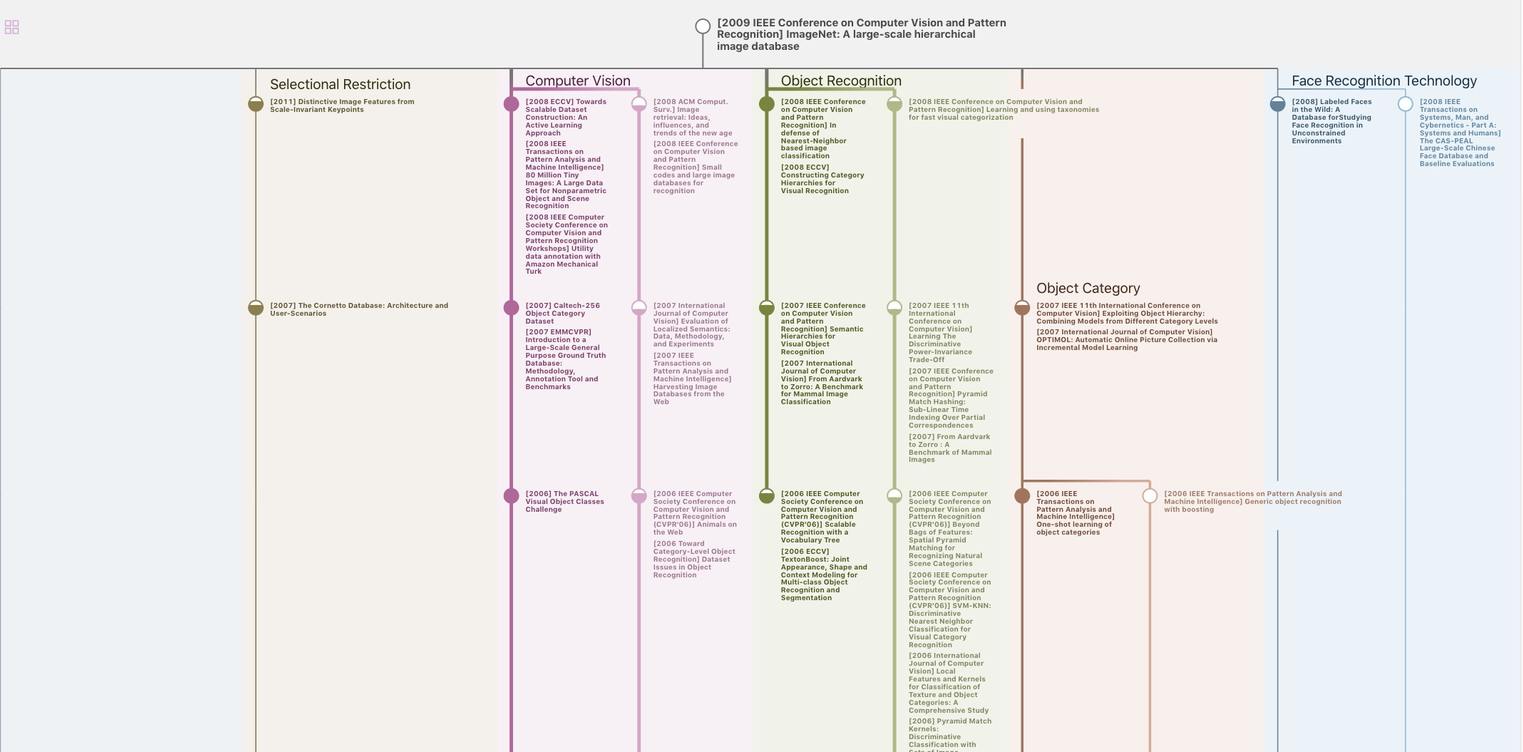
生成溯源树,研究论文发展脉络
Chat Paper
正在生成论文摘要