Multilingual Acoustic Word Embedding Models For Processing Zero-Resource Languages
2020 IEEE INTERNATIONAL CONFERENCE ON ACOUSTICS, SPEECH, AND SIGNAL PROCESSING(2020)
摘要
Acoustic word embeddings are fixed-dimensional representations of variable-length speech segments. In settings where unlabelled speech is the only available resource, such embeddings can be used in "zero-resource" speech search, indexing and discovery systems. Here we propose to train a single supervised embedding model on labelled data from multiple well-resourced languages and then apply it to unseen zero-resource languages. For this transfer learning approach, we consider two multilingual recurrent neural network models: a discriminative classifier trained on the joint vocabularies of all training languages, and a correspondence autoencoder trained to reconstruct word pairs. We test these using a word discrimination task on six target zero-resource languages. When trained on seven well-resourced languages, both models perform similarly and outperform unsupervised models trained on the zero-resource languages. With just a single training language, the second model works better, but performance depends more on the particular training-testing language pair.
更多查看译文
关键词
Acoustic word embeddings, multilingual models, zero-resource speech processing, query-by-example
AI 理解论文
溯源树
样例
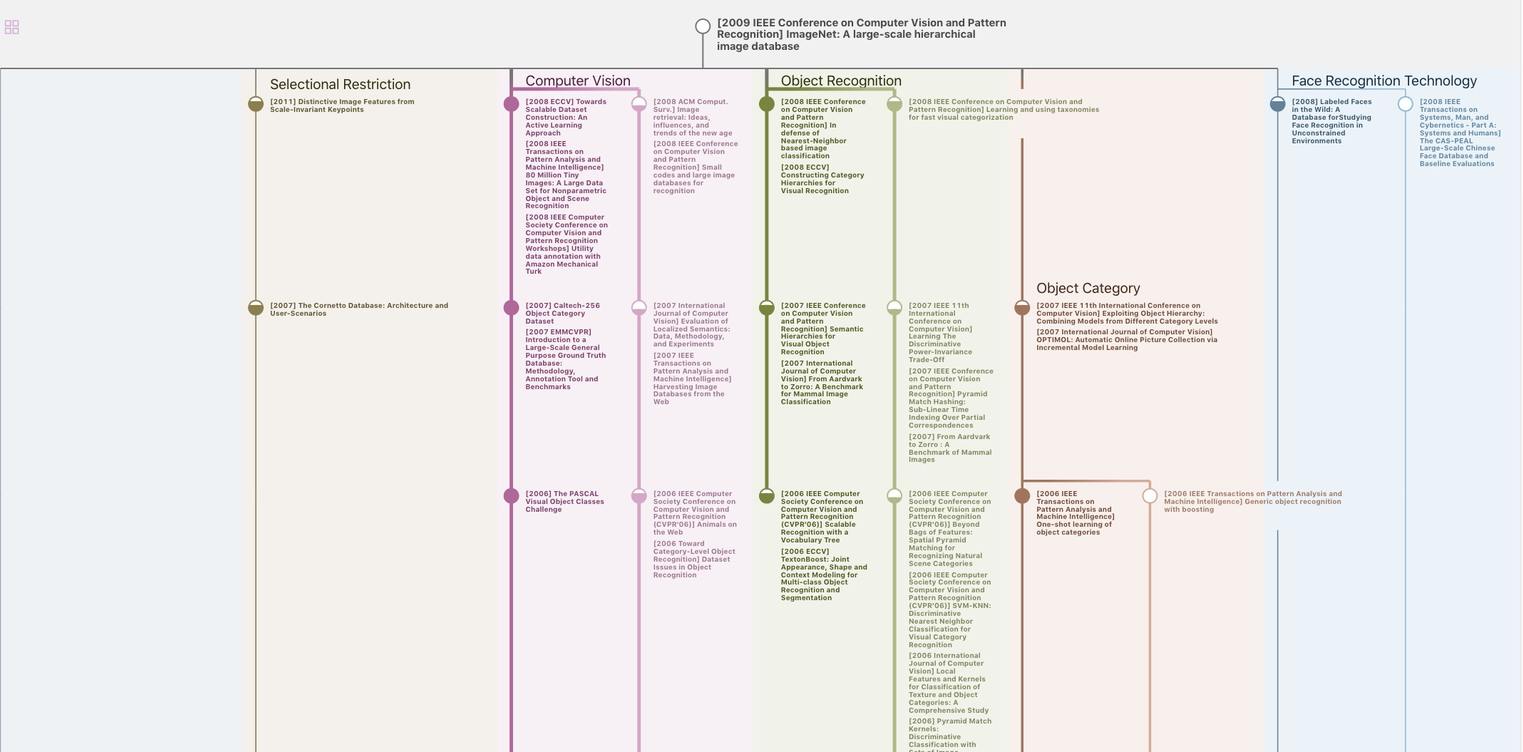
生成溯源树,研究论文发展脉络
Chat Paper
正在生成论文摘要