Investigation of Automatic Video Summarization using Viewer’s Physiological, Facial and Attentional Features
2019 IEEE 15th International Conference on Intelligent Computer Communication and Processing (ICCP)(2019)
摘要
Video summarization aims at the selection of a concise and representative set of keyframes or video segments that allows the identification of the video content. In either cases, traditional summarization techniques usually work by segmenting the video into shots, representing video frames as feature vectors of color, texture, audio, among other features, clustering frames with similar features and selecting most representative keyframes or segments, sometimes guided by a video-to-summary ratio target. The resulting summaries are typically subject independent and do not take into account specific viewer's behavior. Instead of using intrinsic features extracted from the video for summarization, in this article we study whether personalized (subject dependent) video summaries can be obtained from physiological, facial, and attentional data captured from the viewers. More specifically, we study the relationship between personalized video summaries reported by viewers and their data captured during the display of different video genres. A dataset of fifteen videos was used in the experiments. During the exhibition of the videos, the viewer's physiological, facial, and attentional data were recorded, analyzed and synchronized. Several machine learning models were trained to test our hypothesis. We obtained k-fold cross validation accuracies that were above the chance for the best learned models. As a result of this study, we conclude that it is possible to train a learning machine that can produce customized summaries that are closer to user preferences compared to randomly produced summaries.
更多查看译文
关键词
personalized video summaries,different video genres,randomly produced summaries,automatic video summarization,concise,video segments,video content,traditional summarization techniques,video frames,representative keyframes,video-to-summary ratio target,resulting summaries,account specific viewer,intrinsic features,physiological data,facial data,attentional data,machine learning models,k-fold cross validation
AI 理解论文
溯源树
样例
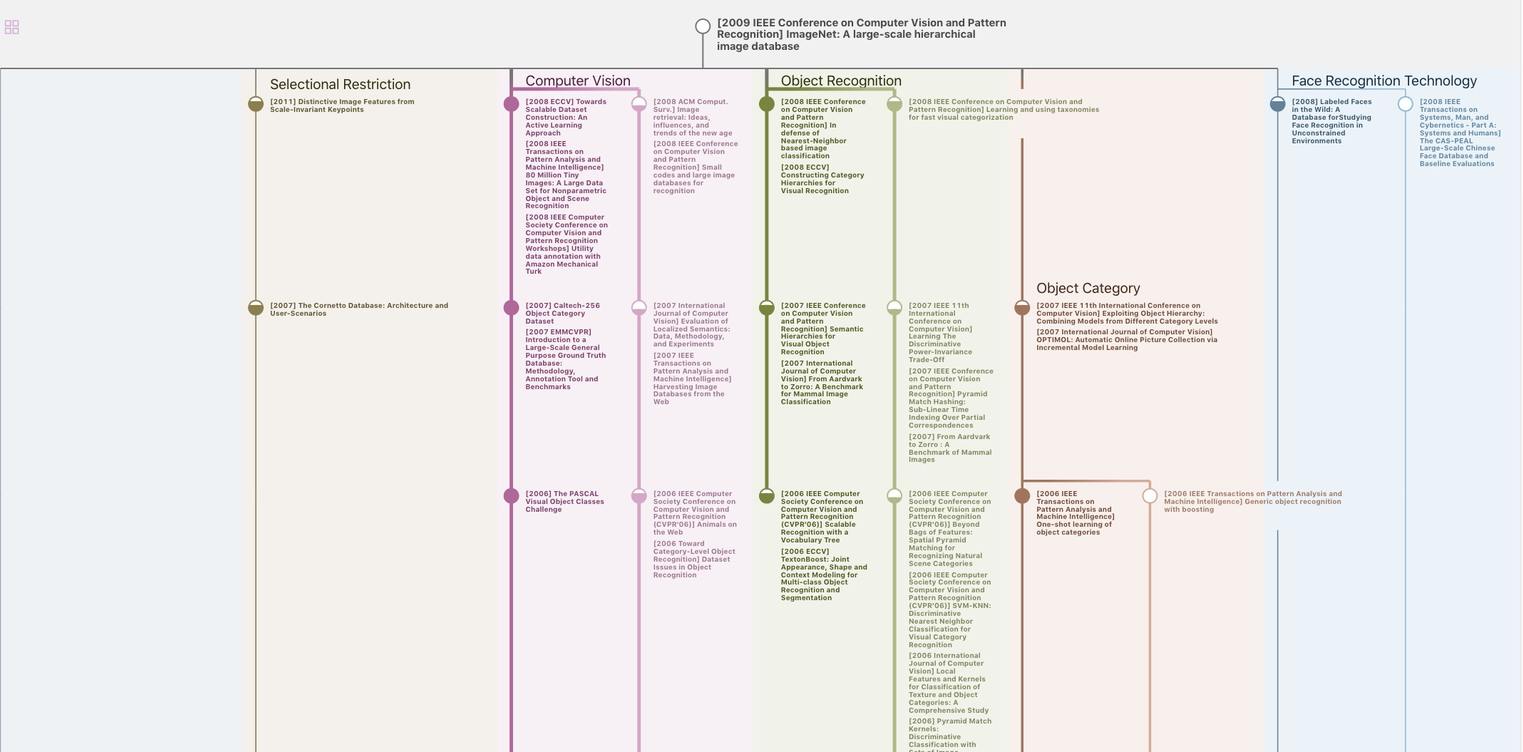
生成溯源树,研究论文发展脉络
Chat Paper
正在生成论文摘要