Data-Driven Modeling Of Semi-Batch Manufacturing: A Rubber Compounding Test Case
2019 IEEE 17TH INTERNATIONAL CONFERENCE ON INDUSTRIAL INFORMATICS (INDIN)(2019)
摘要
The continuously growing amount of available data from manufacturing processes supports the development of data-driven models. The typical target application of these models is optimal control and continuous quality management within an objective of zero-defect manufacturing. However, data obtained from batch processes are characterized by its high dimensionality that exceeds the computational capabilities of online applications and data-driven model's reliability must be guaranteed for proper industrial implementation. We explore two approaches to reduce problem's size: feature extraction and feature selection; several multivariate regression methods are also compared regarding it precision and robustness. We base our analysis on an industrial rubber compounding process where natural rubber is blended in a semi-batch mixer with several additives, then it is further mixed up using cylinders and it is conditioned in bands for storing. For this process, real production data is collected and stored in the manufacturing execution system of the company. The objective of the analysis is to predict mechanical properties of the rubber at the end of the processes. Based on the provided data, several data-driven models are built and tested. From the comparison among them it is concluded: models based on feature extraction and artificial neural networks yield the highest accuracy, while feature-selected models provide better physical interpretability and increased robustness regarding industrial deployment.
更多查看译文
关键词
Process modeling, Data analysis, Rubber industry, Quality prediction, Process monitoring
AI 理解论文
溯源树
样例
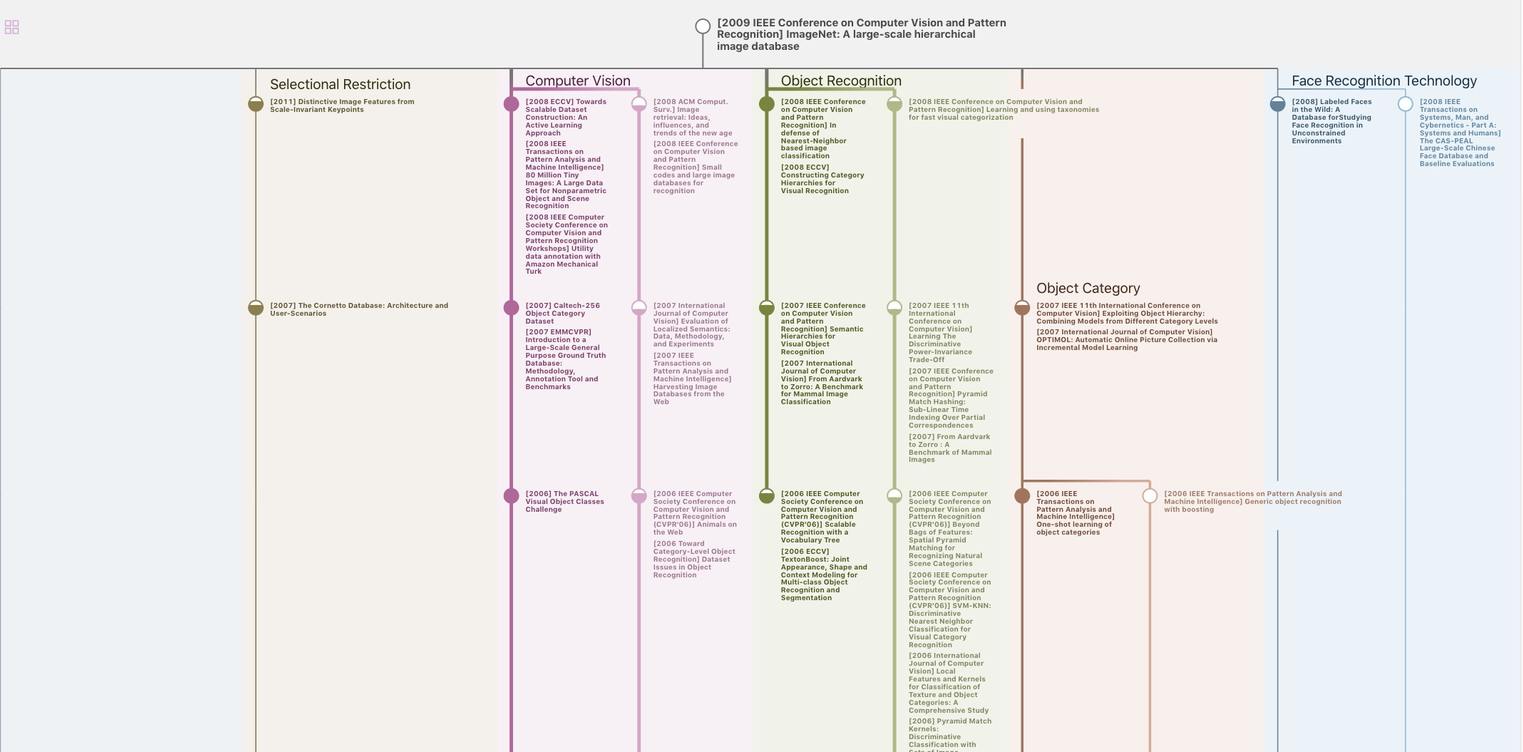
生成溯源树,研究论文发展脉络
Chat Paper
正在生成论文摘要