Unsupervised OCR Model Evaluation Using GAN
ICDAR(2019)
摘要
Optical Character Recognition (OCR) has achieved its state-of-the-art performance with the use of Deep Learning for character recognition. Deep Learning techniques need large amount of data along with ground truth. Out of the available data, small portion of it has to be used for validation purpose as well. Preparing ground truth for historical documents is expensive and hence availability of data is of utmost concern. Jenckel et al. jenckel came up with an idea of using all the available data for training the OCR model and for the purpose of validation, they generated the input image from Softmax layer of the OCR model; using the decoder setup which can be used to compare with the original input image to validate the OCR model. In this paper, we have explored the possibilities of using Generative Adversial Networks (GANs) gan for generating the image directly from the text obtained from OCR model instead of using the Softmax layer which is not always accessible for all the Deep Learning based OCR models. Using text directly to generate the input image back gives us the advantage to use this pipeline for any OCR models even whose Softmax layer is not accessible. In the results section, we have shown that the current state of using GANs for unsupervised OCR model evaluation.
更多查看译文
关键词
ocr model, dense layer, uwiii data, ground truth image, text line, encoded text, ground truth, text line image, softmax layer, latin data, real image, ocred text, final dense layer, synthetic image, encoder decoder setup, pix2pixhd network, top line, bottom line, black pixel, white pixel, deep learning, transposed convolution layer, sigmoid cross entropy, pango library, convolution layer, technical document image database, activation function, deep learning based ocr
AI 理解论文
溯源树
样例
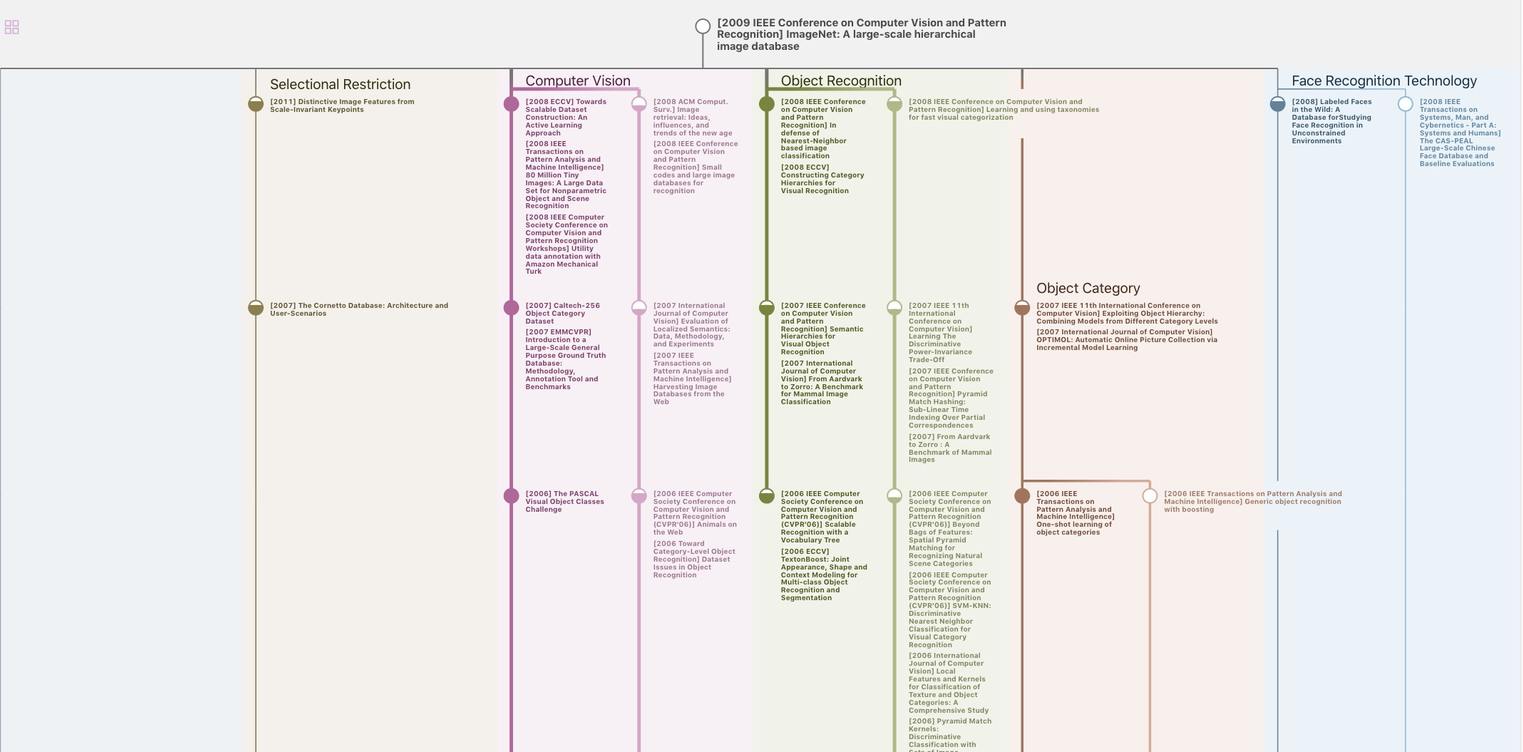
生成溯源树,研究论文发展脉络
Chat Paper
正在生成论文摘要