Analysis of Unsupervised Training Approaches for LSTM-Based OCR.
ICDAR(2019)
摘要
In the context of historical documents, where labeled training data is especially expensive to acquire, the prospect of using unlabeled training data to improve and speed up the overall training is desirable. The most common way to use unlabeled data is unsupervised pretraining, which has been successfully applied to various CNN and RNN architectures in different domains. There is however not sufficient work for its application in the field of OCR. In this paper we investigate multiple architectures and how unlabeled data could be applied to them. We show that in combination with Connectionist Temporal Classification (CTC), a reconstruction objective has no apparent synergistic effect, with both objectives learning different representations. We therefore investigate the use of an LSTM-based Seq2Seq OCR architecture which shows promise regarding unsupervised pretraining.
更多查看译文
关键词
OCR,Unsupervised Pretraining,Historical Documents
AI 理解论文
溯源树
样例
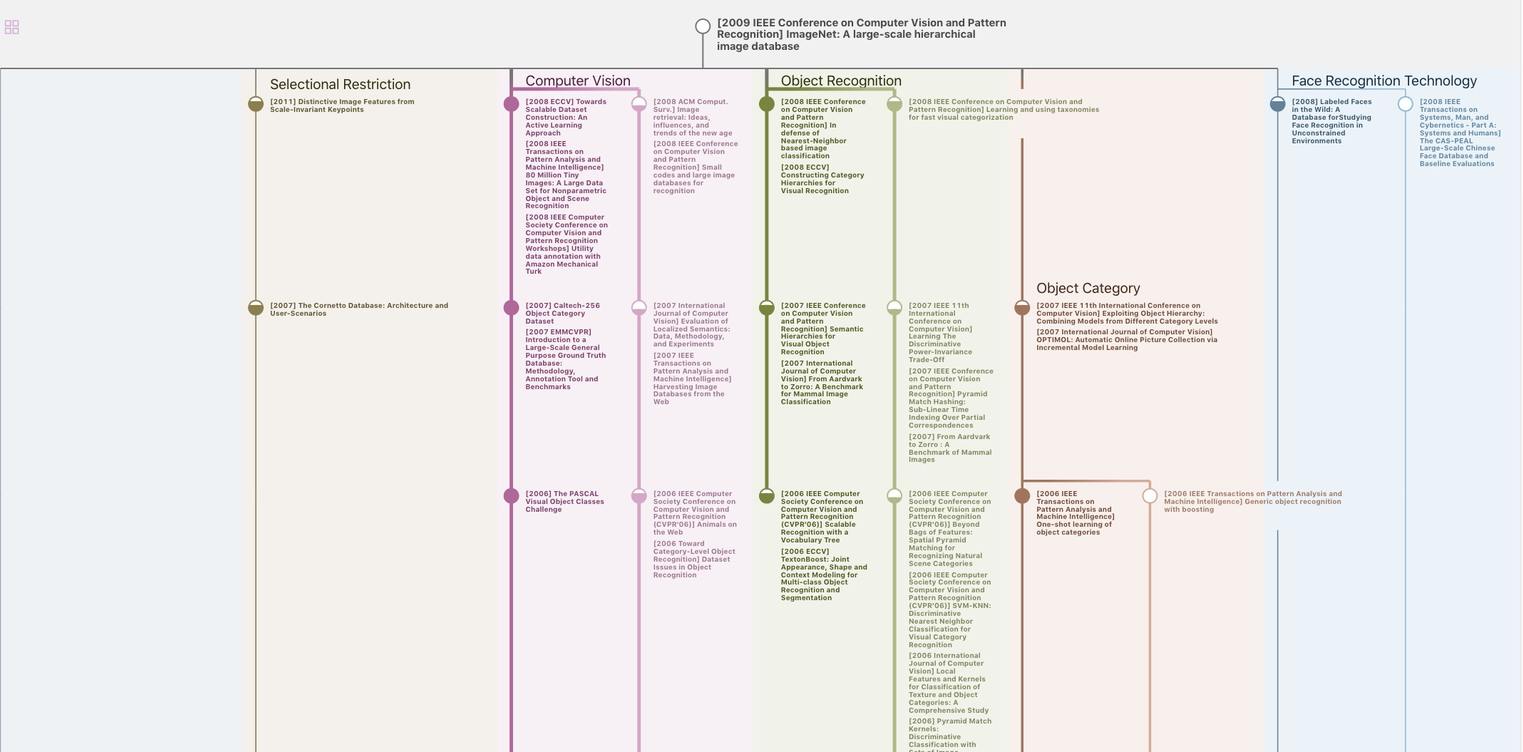
生成溯源树,研究论文发展脉络
Chat Paper
正在生成论文摘要