Semi-Supervised Batch Mode Active Learning For Multi-Class Classification
ADVANCES IN BRAIN INSPIRED COGNITIVE SYSTEMS(2020)
摘要
Most of classification tasks typically require a large number of labelled samples to learn an accurate classifier, while labelled samples are usually limited in practice, and obtaining a lot of labelled samples is a time consuming and laborious work. Active learning is a paradigm to reduce the expensive human action by selecting the most valuable unlabeled samples iteratively. Existing works on active learning evaluate an unlabeled sample by one or two criteria, such as uncertainty, diversity, representativeness and so on. In this paper, three criteria, i.e., uncertainty based on a semi-supervised classifier, diversity based on the distance between samples and representativeness based on the distribution of data, are combined to design a batch mode sample selection strategy for multi-class active learning. By minimizing the expected error with respect to the optimal classifier, we can select the most valuable unlabeled samples for labeling. Experimental results on three public image datasets have demonstrated the effectiveness of our proposed method.
更多查看译文
关键词
Active learning, Semi-supervised learning, Multi-class classification
AI 理解论文
溯源树
样例
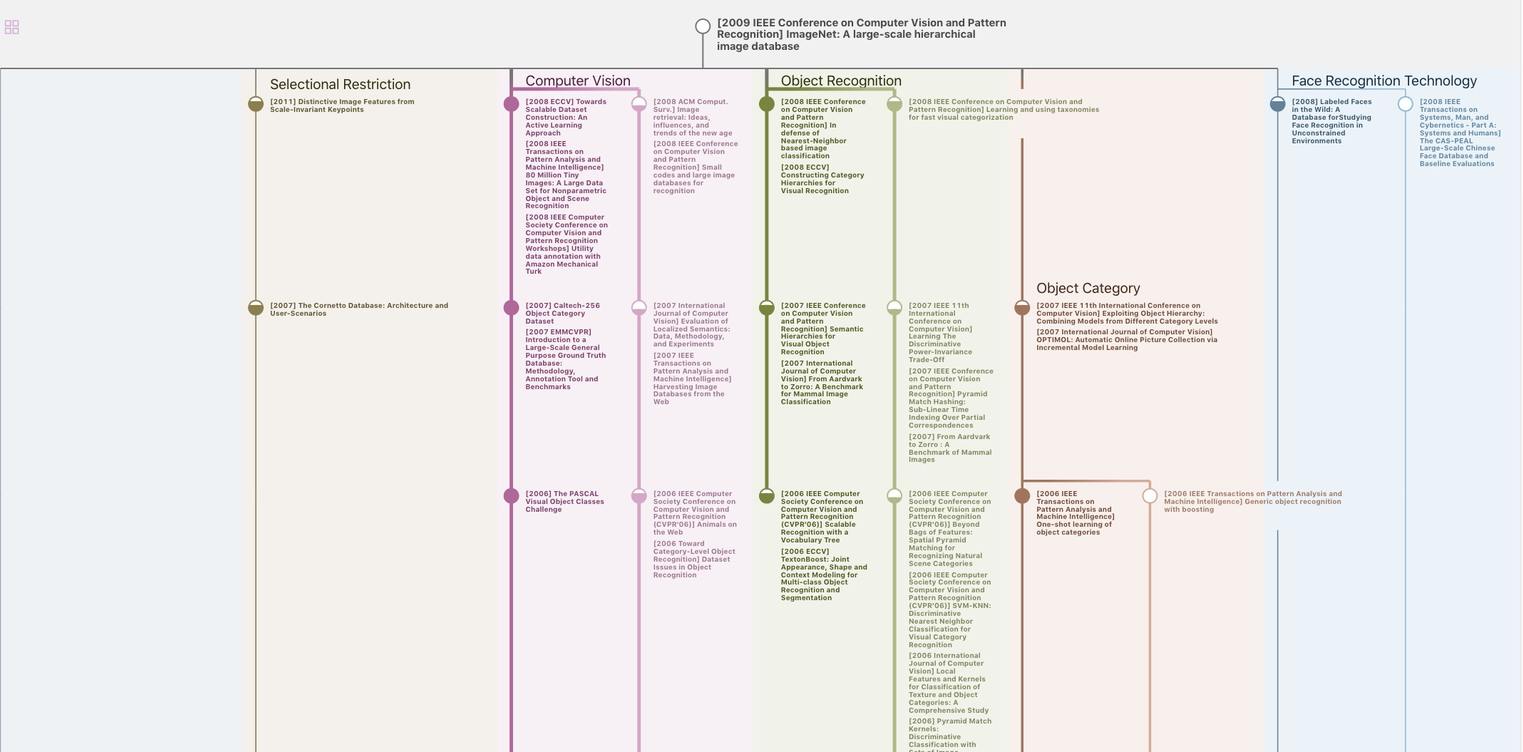
生成溯源树,研究论文发展脉络
Chat Paper
正在生成论文摘要