Understanding the Decision Boundary of Deep Neural Networks: An Empirical Study
CoRR(2020)
摘要
Despite achieving remarkable performance on many image classification tasks, state-of-the-art machine learning (ML) classifiers remain vulnerable to small input perturbations. Especially, the existence of adversarial examples raises concerns about the deployment of ML models in safety- and security-critical environments, like autonomous driving and disease detection. Over the last few years, numerous defense methods have been published with the goal of improving adversarial as well as corruption robustness. However, the proposed measures succeeded only to a very limited extent. This limited progress is partly due to the lack of understanding of the decision boundary and decision regions of deep neural networks. Therefore, we study the minimum distance of data points to the decision boundary and how this margin evolves over the training of a deep neural network. By conducting experiments on MNIST, FASHION-MNIST, and CIFAR-10, we observe that the decision boundary moves closer to natural images over training. This phenomenon even remains intact in the late epochs of training, where the classifier already obtains low training and test error rates. On the other hand, adversarial training appears to have the potential to prevent this undesired convergence of the decision boundary.
更多查看译文
关键词
decision boundary,deep neural networks,neural networks,empirical study
AI 理解论文
溯源树
样例
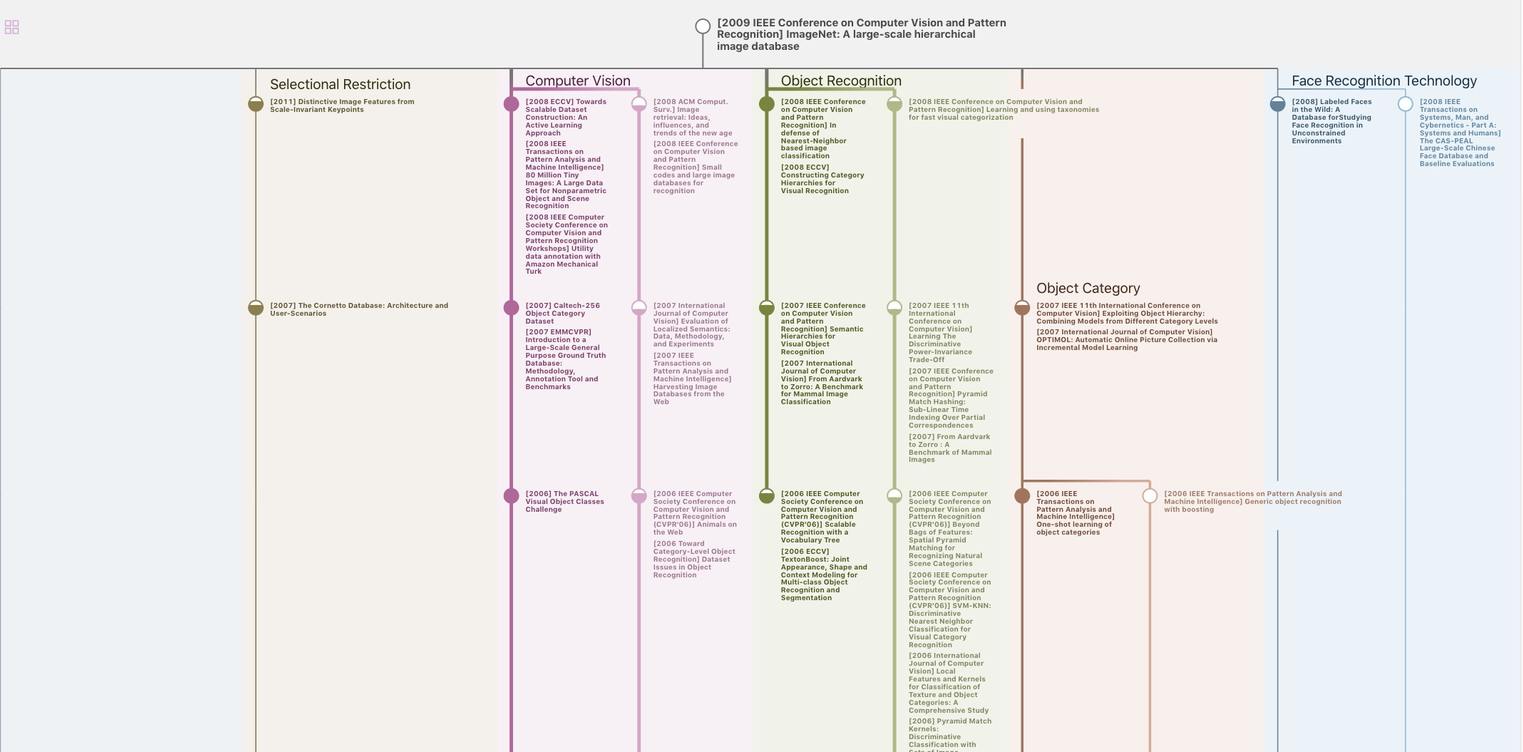
生成溯源树,研究论文发展脉络
Chat Paper
正在生成论文摘要