Illumination Adaptive Person Reid Based On Teacher-Student Model And Adversarial Training
2020 IEEE INTERNATIONAL CONFERENCE ON IMAGE PROCESSING (ICIP)(2020)
摘要
Most existing works in Person Re-identification (ReID) focus on settings where illumination either is kept the same or has very little fluctuation. However, the changes in the illumination degree may affect the robustness of a ReID algorithm significantly. To address this problem, we proposed a Two-Stream Network that can separate ReID features from lighting features to enhance ReID performance. Its innovations are threefold: (1) A discriminative entropy loss to ensure the ReID features contain no lighting information. (2) A ReID Teacher model trained by images under "neutral" lighting conditions to guide ReID classification. (3) An illumination Teacher model trained by the differences between the illumination-adjusted and original images to guide illumination classification. We construct two augmented datasets by synthetically changing a set of predefined lighting conditions in two of the most popular ReID benchmarks: Market1501 and DukeMTMC-ReID. Experiments demonstrate that our algorithm outperforms other state-of-the-art works and particularly potent in handling images under extremely low light.
更多查看译文
关键词
Person re-identification, illumination adaptive, discriminative loss, Teacher-Student model
AI 理解论文
溯源树
样例
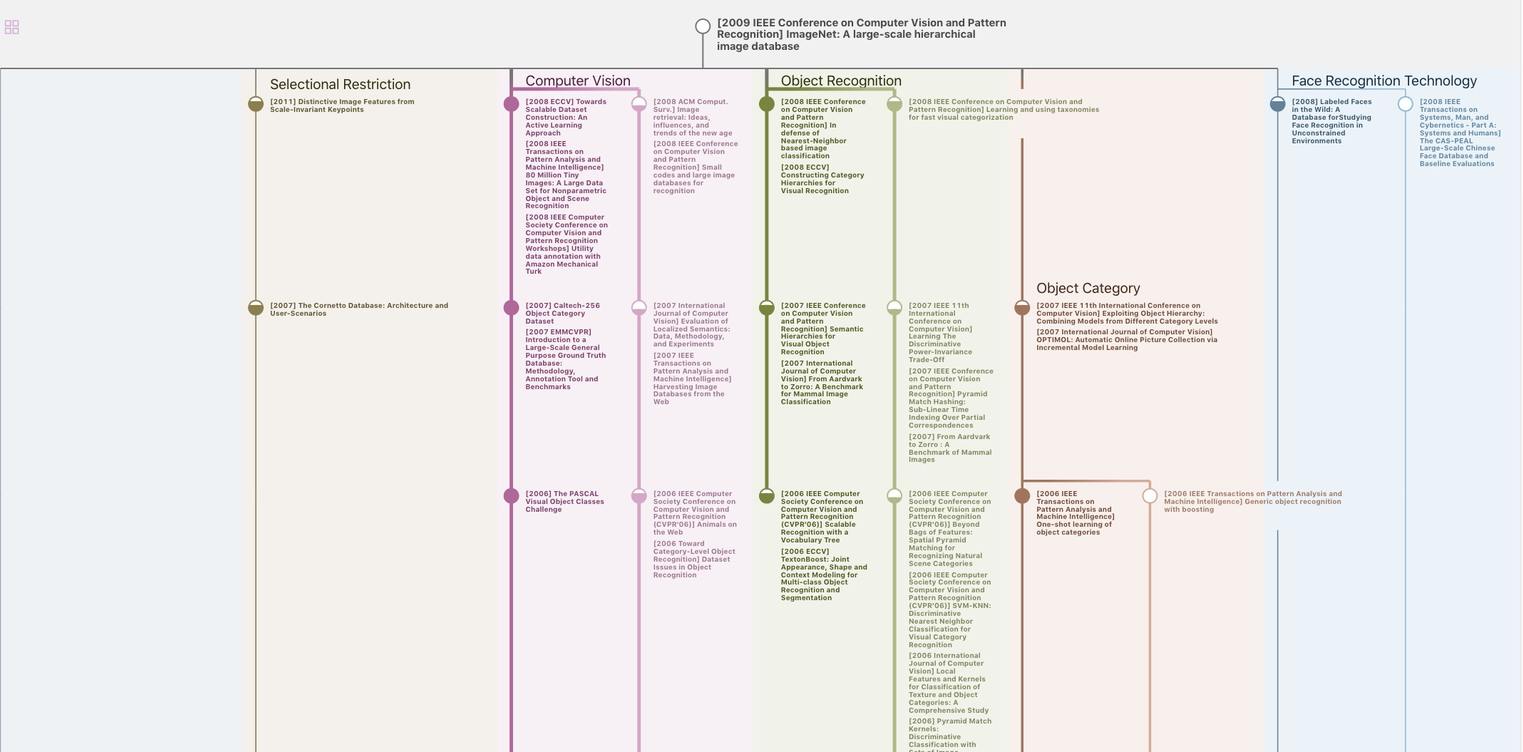
生成溯源树,研究论文发展脉络
Chat Paper
正在生成论文摘要