Camp: Co-Attention Memory Networks For Diagnosis Prediction In Healthcare
2019 19TH IEEE INTERNATIONAL CONFERENCE ON DATA MINING (ICDM 2019)(2019)
摘要
Diagnosis prediction, which aims to predict future health information of patients from historical electronic health records (EHRs), is a core research task in personalized healthcare. Although some RNN-based methods have been proposed to model sequential EHR data, these methods have two major issues. First, they cannot capture fine-grained progression patterns of patient health conditions. Second, they do not consider the mutual effect between important context (e.g., patient demographics) and historical diagnosis. To tackle these challenges, we propose a model called Co-Attention Memory networks for diagnosis Prediction (CAMP), which tightly integrates historical records, fine-grained patient conditions, and demographics with a three-way interaction architecture built on co-attention. Our model augments RNNs with a memory network to enrich the representation capacity. The memory network enables analysis of fine-grained patient conditions by explicitly incorporating a taxonomy of diseases into an array of memory slots. We instantiate the READ/WRITE operations of the memory network so that the memory cooperates effectively with the patient demographics through co-attention mechanism. Experiments on real-world datasets demonstrate that CAMP consistently performs better than state-of-the-art methods.
更多查看译文
关键词
diagnosis prediction, memory networks, attention mechanism, healthcare informatics
AI 理解论文
溯源树
样例
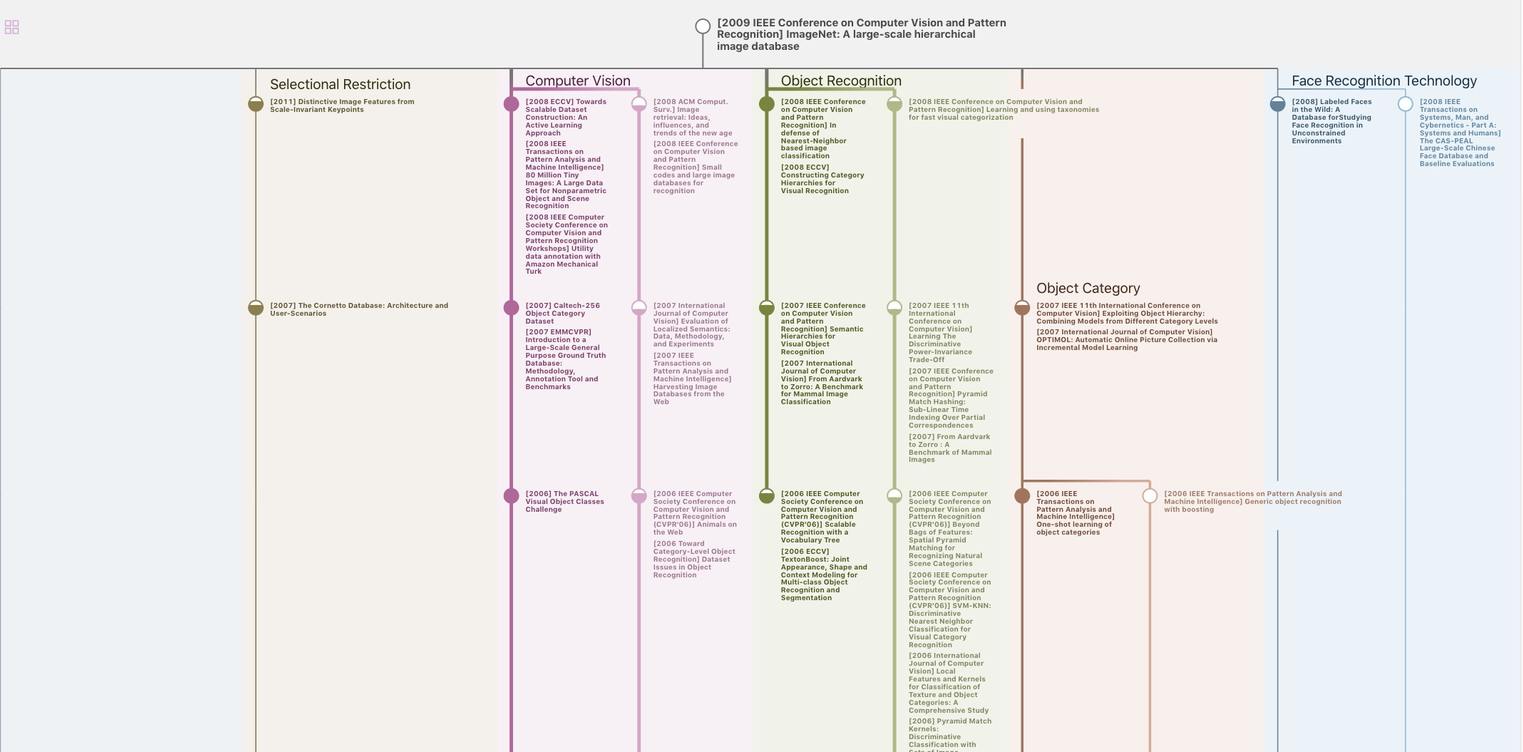
生成溯源树,研究论文发展脉络
Chat Paper
正在生成论文摘要