DNNLibGen: Deep Neural Network Based Fast Library Generator
2019 26TH IEEE INTERNATIONAL CONFERENCE ON ELECTRONICS, CIRCUITS AND SYSTEMS (ICECS)(2019)
摘要
We propose a new modeling methodology using deep learning techniques for generating timing models for Static Timing Analysis (STA). Current device behavior is non-linear, non-monotonic and exhibits high sensitivity to (Process Voltage Temperature) PVT variation which imposes a myriad of design challenges including the need for analysis at several PVT corners. While complete PVT coverage is crucial for detecting design issues early and achieving time-to-market goals with improved predictability, the number of PVT corners are growing exponentially and library generation has also become a significant bottleneck in current design cycles. To this end, we have developed a novel methodology for timing library generation that uses data from sparse characterization in PVT space and generates delay models at required sign-off corners. We have employed deep neural nets with residual connections for delay modeling and our methodology enables a `single model' to fully comprehend multiple cell types, PVT corners and generate required PVT timing libraries. The proposed library-generator uses a novel inter-corner model to generate delay tables at 17 test corners using 7 corners as reference. In addition, we have developed an intra-corner model, to generate dense 8x8 delay tables using delays from 10 slew/load points as reference. The results show that, using these models, we are able to achieve key improvements with over 98.7% of calculated delays within acceptable tolerance while reducing characterization run-time for early milestones by upto 60%.
更多查看译文
关键词
Library cell characterization, Neural networks, Spice, PVT, Static timing, EDA
AI 理解论文
溯源树
样例
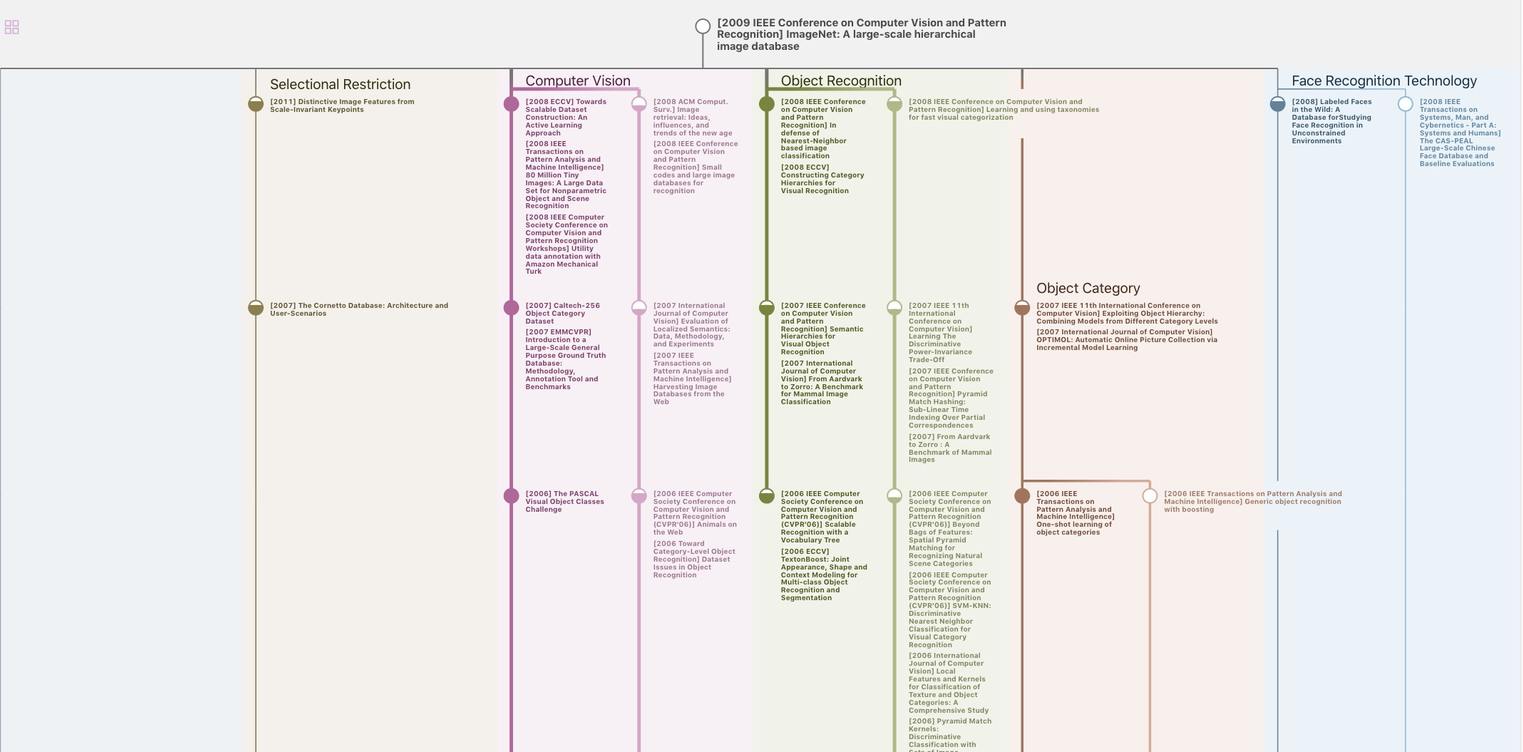
生成溯源树,研究论文发展脉络
Chat Paper
正在生成论文摘要