Deep learning-based MR fingerprinting ASL ReconStruction (DeepMARS)
MAGNETIC RESONANCE IN MEDICINE(2020)
摘要
Purpose To develop a reproducible and fast method to reconstruct MR fingerprinting arterial spin labeling (MRF-ASL) perfusion maps using deep learning. Method A fully connected neural network, denoted as DeepMARS, was trained using simulation data and added Gaussian noise. Two MRF-ASL models were used to generate the simulation data, specifically a single-compartment model with 4 unknowns parameters and a two-compartment model with 7 unknown parameters. The DeepMARS method was evaluated using MRF-ASL data from healthy subjects (N = 7) and patients with Moymoya disease (N = 3). Computation time, coefficient of determination (R-2), and intraclass correlation coefficient (ICC) were compared between DeepMARS and conventional dictionary matching (DM). The relationship between DeepMARS and Look-Locker PASL was evaluated by a linear mixed model. Results Computation time per voxel was 4 seconds for DM in the single-compartment model. Compared with DM, the DeepMARS showed higher R-2 and significantly improved ICC for single-compartment derived bolus arrival time (BAT) and two-compartment derived cerebral blood flow (CBF) and higher or similar R-2/ICC for other parameters. In addition, the DeepMARS was significantly correlated with Look-Locker PASL for BAT (single-compartment) and CBF (two-compartment). Moreover, for Moyamoya patients, the location of diminished CBF and prolonged BAT shown in DeepMARS was consistent with the position of occluded arteries shown in time-of-flight MR angiography. Conclusion Reconstruction of MRF-ASL with DeepMARS is faster and more reproducible than DM.
更多查看译文
关键词
deep learning,DeepMARS,MRF-ASL,reconstruction,reproducibility
AI 理解论文
溯源树
样例
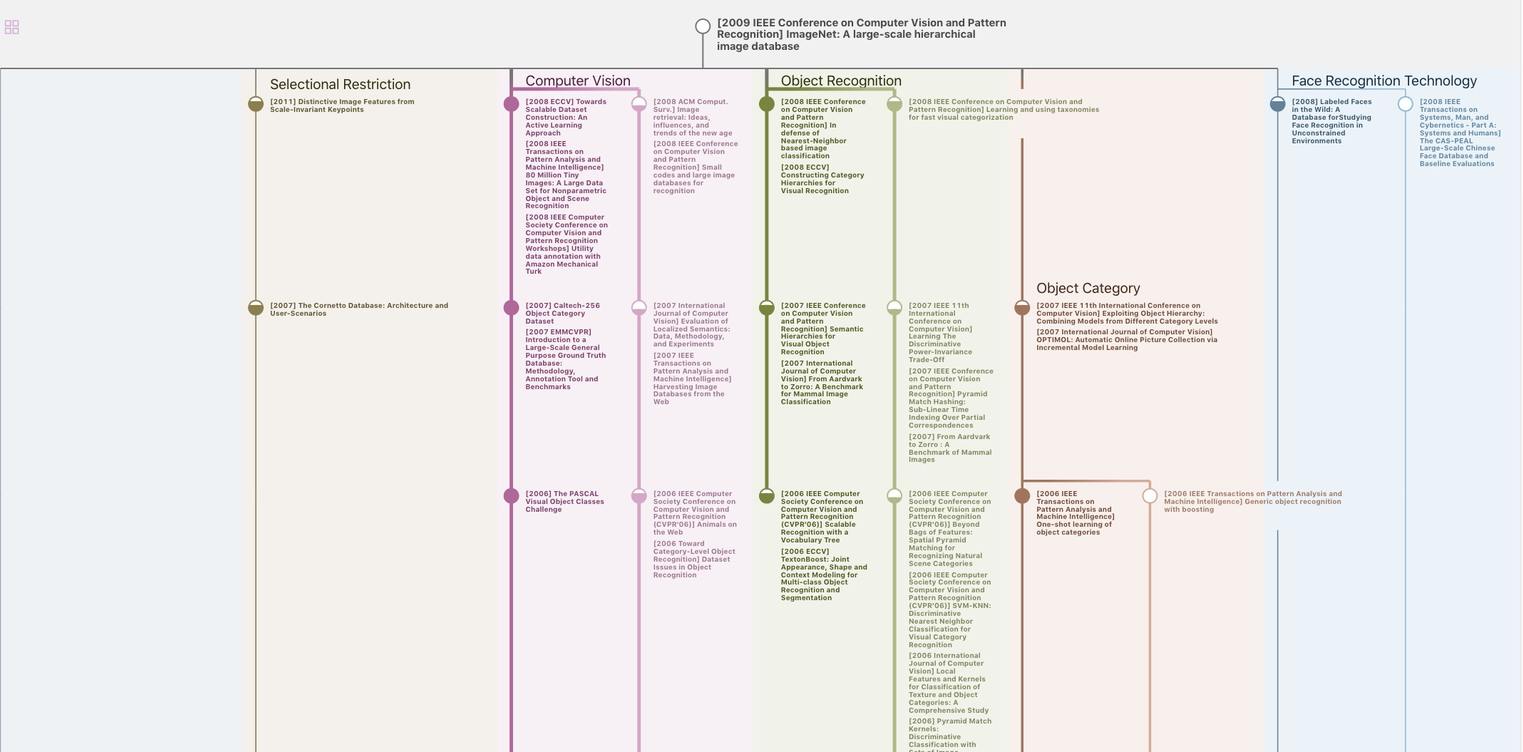
生成溯源树,研究论文发展脉络
Chat Paper
正在生成论文摘要