Texture classification using block intensity and gradient difference (BIGD) descriptor
Signal Processing: Image Communication(2020)
摘要
In this paper, we present an efficient and distinctive local descriptor, namely block intensity and gradient difference (BIGD). In an image patch, we randomly sample multi-scale block pairs and utilize the intensity and gradient differences of pairwise blocks to construct the local BIGD descriptor. The random sampling strategy and the multi-scale framework help BIGD descriptors capture the distinctive patterns of patches at different orientations and spatial granularity levels. We use vectors of locally aggregated descriptors (VLAD) or improved Fisher vector (IFV) to encode local BIGD descriptors into a full image descriptor, which is then fed into a linear support vector machine (SVM) classifier for texture classification. We compare the proposed descriptor with typical and state-of-the-art ones by evaluating their classification performance on five public texture data sets including Brodatz, CUReT, KTH-TIPS, and KTH-TIPS-2a and -2b. Experimental results show that the proposed BIGD descriptor with stronger discriminative power yields 0.12%∼6.43% higher classification accuracy than the state-of-the-art texture descriptor, dense microblock difference (DMD).
更多查看译文
关键词
Local descriptor,Block intensity and gradient difference (BIGD),Local feature extraction,Multi-scale,Texture classification
AI 理解论文
溯源树
样例
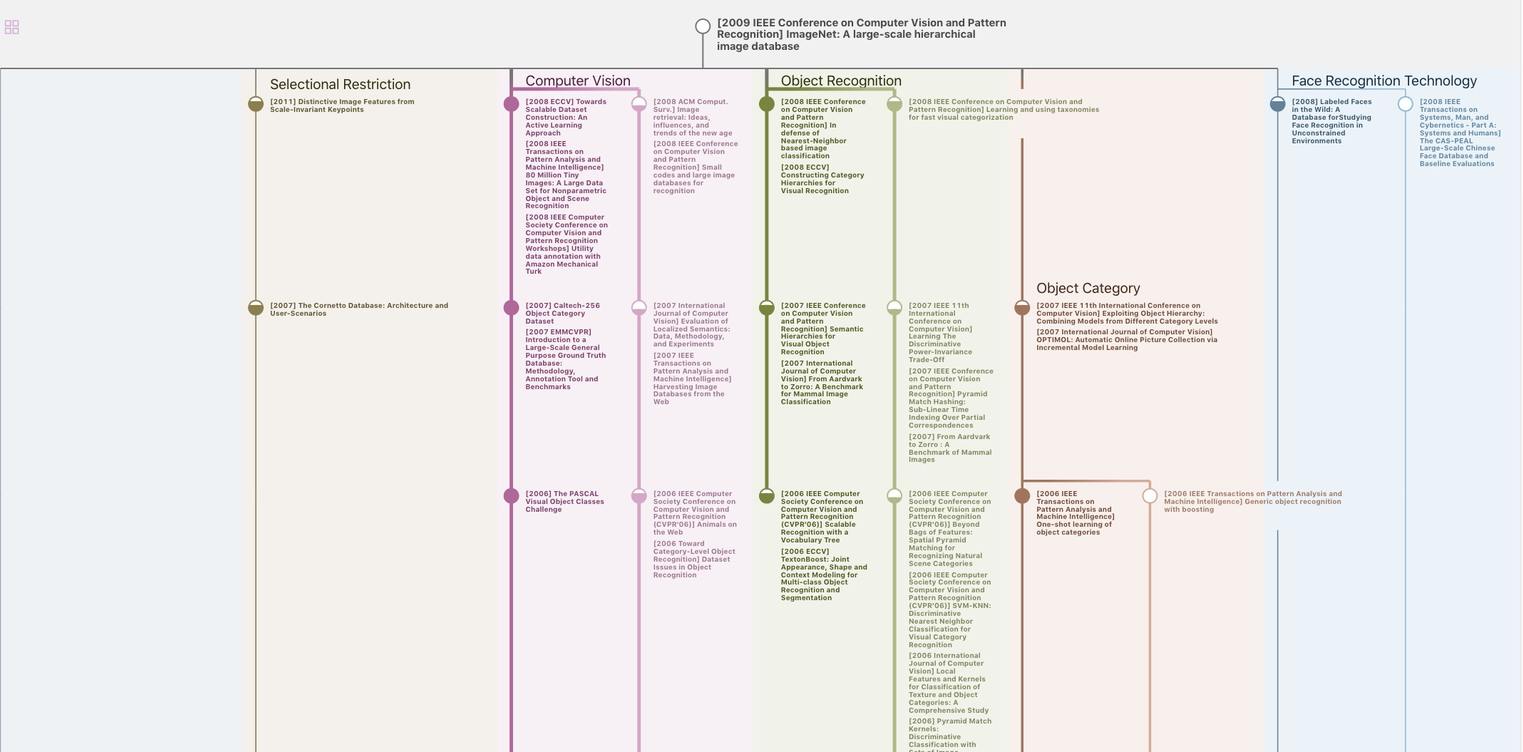
生成溯源树,研究论文发展脉络
Chat Paper
正在生成论文摘要