Deep-Url: A Model-Aware Approach To Blind Deconvolution Based On Deep Unfolded Richardson-Lucy Network
2020 IEEE INTERNATIONAL CONFERENCE ON IMAGE PROCESSING (ICIP)(2020)
摘要
The lack of interpretability in current deep learning models causes serious concerns as they are extensively used for various life-critical applications. Hence, it is of paramount importance to develop interpretable deep learning models. In this paper, we consider the problem of blind deconvolution and propose a novel model-aware deep architecture that allows for the recovery of both the blur kernel and the sharp image from the blurred image. In particular, we propose the Deep Unfolded Richardson-Lucy (Deep-URL) framework-an interpretable deep-learning architecture that can be seen as an amalgamation of classical estimation technique and deep neural network, and consequently leads to improved performance. Our numerical investigations demonstrate significant improvement compared to state-of-the-art algorithms.
更多查看译文
关键词
Blind deconvolution, model-aware deep learning, machine learning, deep unfolding, non-convex optimization
AI 理解论文
溯源树
样例
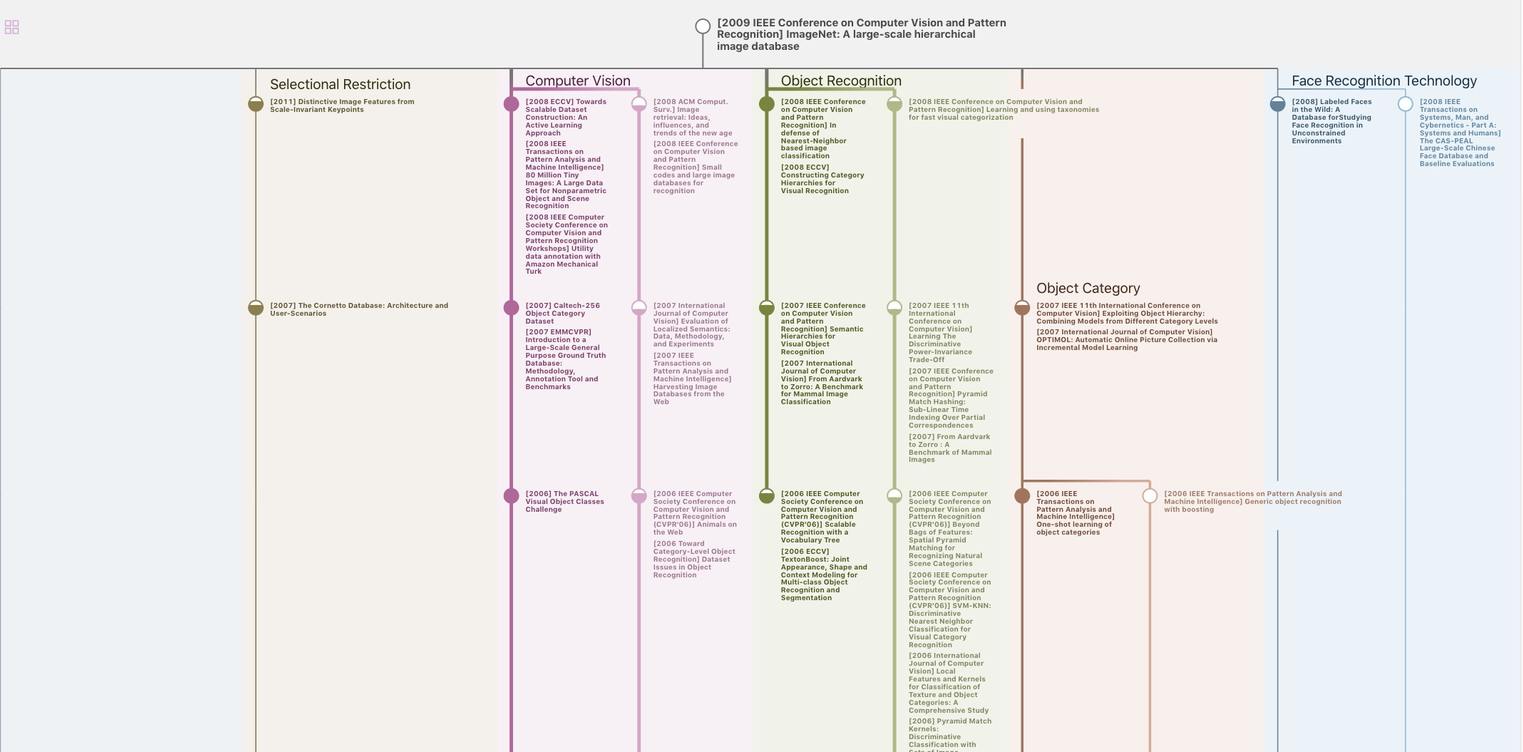
生成溯源树,研究论文发展脉络
Chat Paper
正在生成论文摘要