IART: Intent-aware Response Ranking with Transformers in Information-seeking Conversation Systems
WWW '20: The Web Conference 2020 Taipei Taiwan April, 2020(2020)
Abstract
Personal assistant systems, such as Apple Siri, Google Assistant, Amazon Alexa, and Microsoft Cortana, are becoming ever more widely used. Understanding user intent such as clarification questions, potential answers and user feedback in information-seeking conversations is critical for retrieving good responses. In this paper, we analyze user intent patterns in information-seeking conversations and propose an intent-aware neural response ranking model “IART”, which refers to “Intent-Aware Ranking with Transformers”. IART is built on top of the integration of user intent modeling and language representation learning with the Transformer architecture, which relies entirely on a self-attention mechanism instead of recurrent nets [35]. It incorporates intent-aware utterance attention to derive an importance weighting scheme of utterances in conversation context with the aim of better conversation history understanding. We conduct extensive experiments with three information-seeking conversation data sets including both standard benchmarks and commercial data. Our proposed model outperforms all baseline methods with respect to a variety of metrics. We also perform case studies and analysis of learned user intent and its impact on response ranking in information-seeking conversations to provide interpretation of results.
MoreTranslated text
Key words
conversation systems,response,intent-aware,information-seeking
AI Read Science
Must-Reading Tree
Example
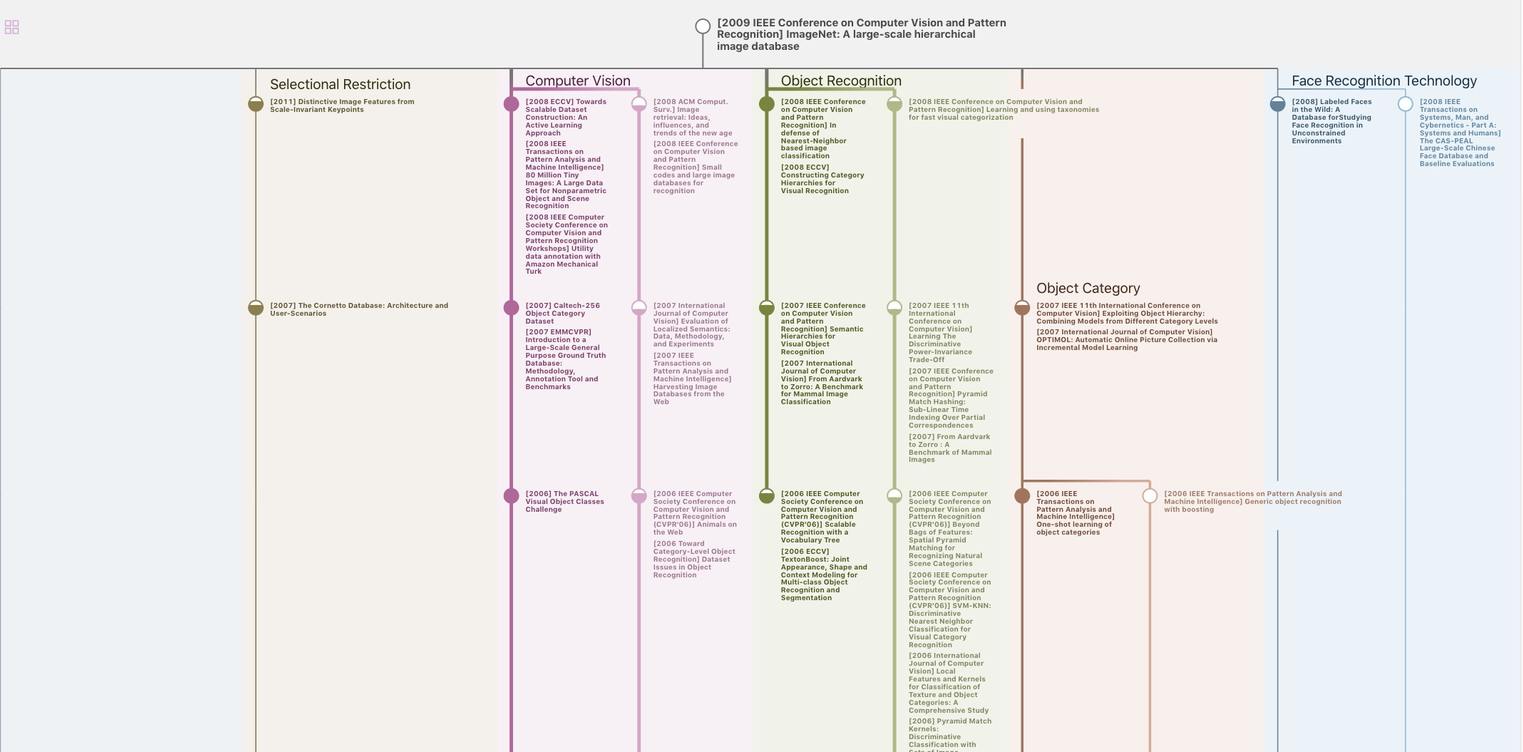
Generate MRT to find the research sequence of this paper
Chat Paper
Summary is being generated by the instructions you defined