Dwm: A Decomposable Winograd Method For Convolution Acceleration
THIRTY-FOURTH AAAI CONFERENCE ON ARTIFICIAL INTELLIGENCE, THE THIRTY-SECOND INNOVATIVE APPLICATIONS OF ARTIFICIAL INTELLIGENCE CONFERENCE AND THE TENTH AAAI SYMPOSIUM ON EDUCATIONAL ADVANCES IN ARTIFICIAL INTELLIGENCE(2020)
Abstract
Winograd's minimal filtering algorithm has been widely used in Convolutional Neural Networks (CNNs) to reduce the number of multiplications for faster processing. I Iowever, it is only effective on convolutions with kernel size as 3x3 and stride as 1, because it suffers from significantly increased FLOPs and numerical accuracy problem for kernel size larger than 3x3 and fails on convolution with stride larger than 1. In this paper, we propose a novel Decomposable Winograd Method (DWM), which breaks through the limitation of original Winograd's minimal filtering algorithm to a wide and general convolutions. DWM decomposes kernels with large size or large stride to several small kernels with stride as 1 for further applying Winograd method, so that DWM can reduce the number of multiplications while keeping the numerical accuracy. It enables the fast exploring of larger kernel size and larger stride value in CNNs for high performance and accuracy and even the potential for new CNNs. Comparing against the original Winograd, the proposed DWM is able to support all kinds of convolutions with a speedup of similar to 2 without affecting the numerical accuracy.
MoreTranslated text
AI Read Science
Must-Reading Tree
Example
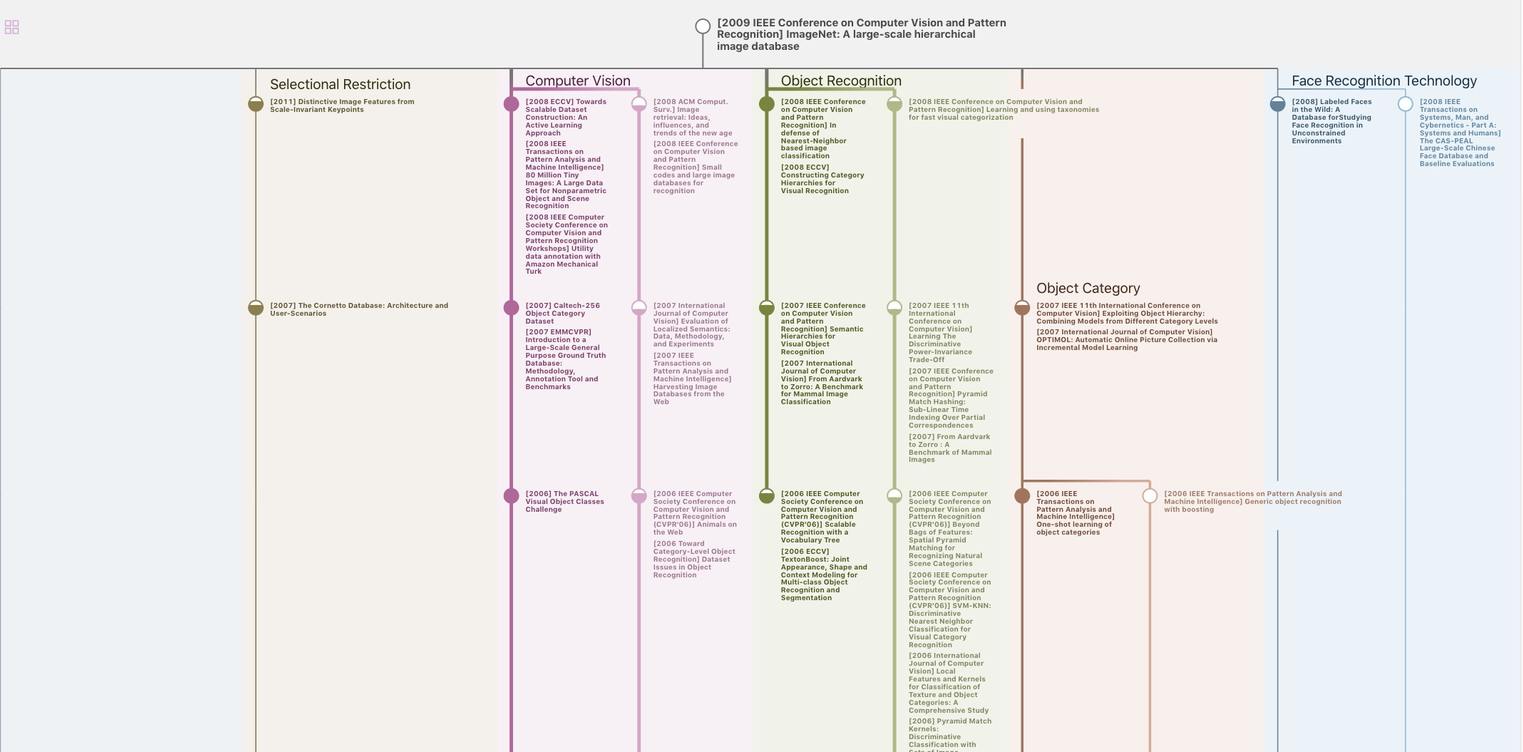
Generate MRT to find the research sequence of this paper
Chat Paper
Summary is being generated by the instructions you defined