Learning How to Walk: Warm-starting Optimal Control Solver with Memory of Motion
ICRA(2020)
摘要
In this paper, we propose a framework to build a memory of motion to warm-start an optimal control solver for the locomotion task of the humanoid robot Talos. We use HPP Loco3D, a versatile locomotion planner, to generate offline a set of dynamically consistent whole-body trajectory to be stored as the memory of motion. The learning problem is formulated as a regression problem to predict a single-step motion given the desired contact locations, which is then used as building block for multi-step motions. The predicted trajectory is then used as warm-starts for the fast optimal control solver Crocoddyl. We have shown that the approach manages to reduce the required number of iterations to reach the convergence from $\sim$9.5 to only $\sim$3.0 iterations for the single-step motion and from $\sim$6.2 to $\sim$4.5 iterations for the multi-step motion, while maintaining the solution's quality.
更多查看译文
关键词
optimal control solver,locomotion task,humanoid robot,HPP Loco3D,versatile locomotion planner,whole-body trajectory,regression problem,single-step motion,multistep motion,predicted motion,Crocoddyl control solver
AI 理解论文
溯源树
样例
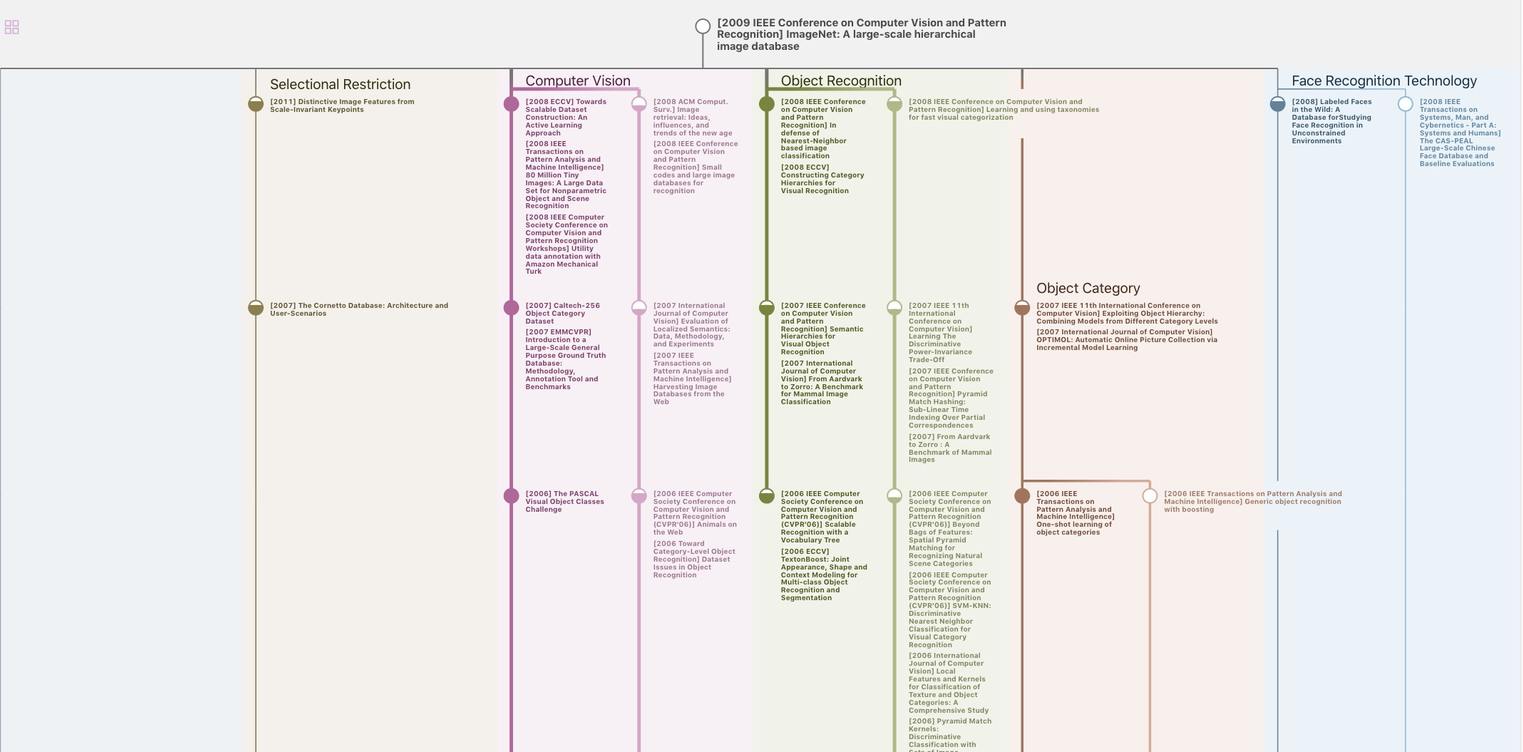
生成溯源树,研究论文发展脉络
Chat Paper
正在生成论文摘要