CosmoVAE: Variational Autoencoder for CMB Image Inpainting
2020 INTERNATIONAL JOINT CONFERENCE ON NEURAL NETWORKS (IJCNN)(2020)
摘要
Cosmic microwave background radiation (CMB) is critical to the understanding of the early universe and precise estimation of cosmological constants. Due to the contamination of thermal dust noise in the galaxy, the CMB map that is an image on the two-dimensional sphere has missing observations, mainly concentrated on the equatorial region. The noise of the CMB map has a significant impact on the estimation precision for cosmological parameters. Inpainting the CMB map can effectively reduce the uncertainty of parametric estimation. In this paper, we propose a deep learning-based variational autoencoder --- CosmoVAE, to restoring the missing observations of the CMB map. The input and output of CosmoVAE are square images. To generate training, validation, and test data sets, we segment the full-sky CMB map into many small images by Cartesian projection. CosmoVAE assigns physical quantities to the parameters of the VAE network by using the angular power spectrum of the Gaussian random field as latent variables. CosmoVAE adopts a new loss function to improve the learning performance of the model, which consists of $\ell_1$ reconstruction loss, Kullback-Leibler divergence between the posterior distribution of encoder network and the prior distribution of latent variables, perceptual loss, and total-variation regularizer. The proposed model achieves state of the art performance for Planck \texttt{Commander} 2018 CMB map inpainting.
更多查看译文
关键词
variational autoencoder, cosmic microwave background, inpainting, deep learning, convolutional neural networks, uncertainty quantification, KL-divergence regularization, perceptual loss, total variation, angular power spectrum, VGG-16, ImageNet
AI 理解论文
溯源树
样例
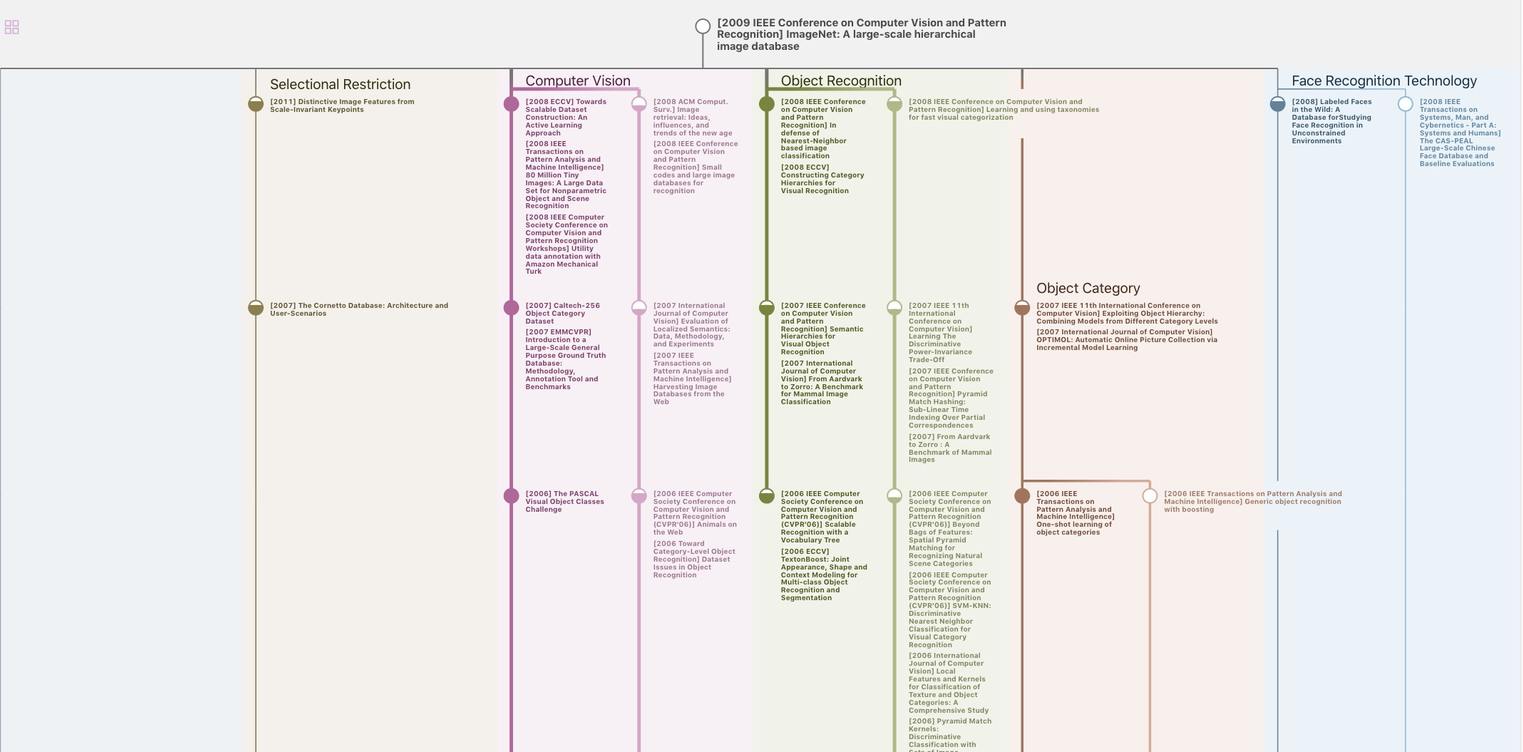
生成溯源树,研究论文发展脉络
Chat Paper
正在生成论文摘要