Deep Supervised Hashing With Similar Hierarchy For Place Recognition
2019 IEEE/RSJ INTERNATIONAL CONFERENCE ON INTELLIGENT ROBOTS AND SYSTEMS (IROS)(2019)
摘要
Place recognition as one of the most significant requirements for long-term simultaneous localization and mapping (SLAM) has been developed rapidly in recent years. Also, deep learning is proved to be more capable than traditional methods to extract features under some complex environments. However, in real-world environments, there are many challenging problems such as viewpoint changes and illumination changes. The existing deep learning-based place recognition in extracting feature phases and matching process is both time-consuming. Moreover, features extracted from convolution neural network (CNN) are floating-point type with high dimension. In this paper, we propose deep supervised hashing for place recognition, where we design a similar hierarchy loss function to learn a model. The model can distinguish the similar images more accurately which is well suitable to place recognition. Besides the model can learn high quality hash codes by maximizing the likelihood of triplet labels. Experiments on several benchmark datasets for place recognition show that our approach is robust to viewpoints, illuminations and season changes with high accuracy. Furthermore, the trained model can extract features and match in real time on CPU with less memory consumption.
更多查看译文
关键词
deep supervised hashing,long-term simultaneous localization,deep learning,similar hierarchy loss function,high quality hash codes,place recognition,simultaneous localization and mapping,SLAM,convolution neural network,CNN,CPU
AI 理解论文
溯源树
样例
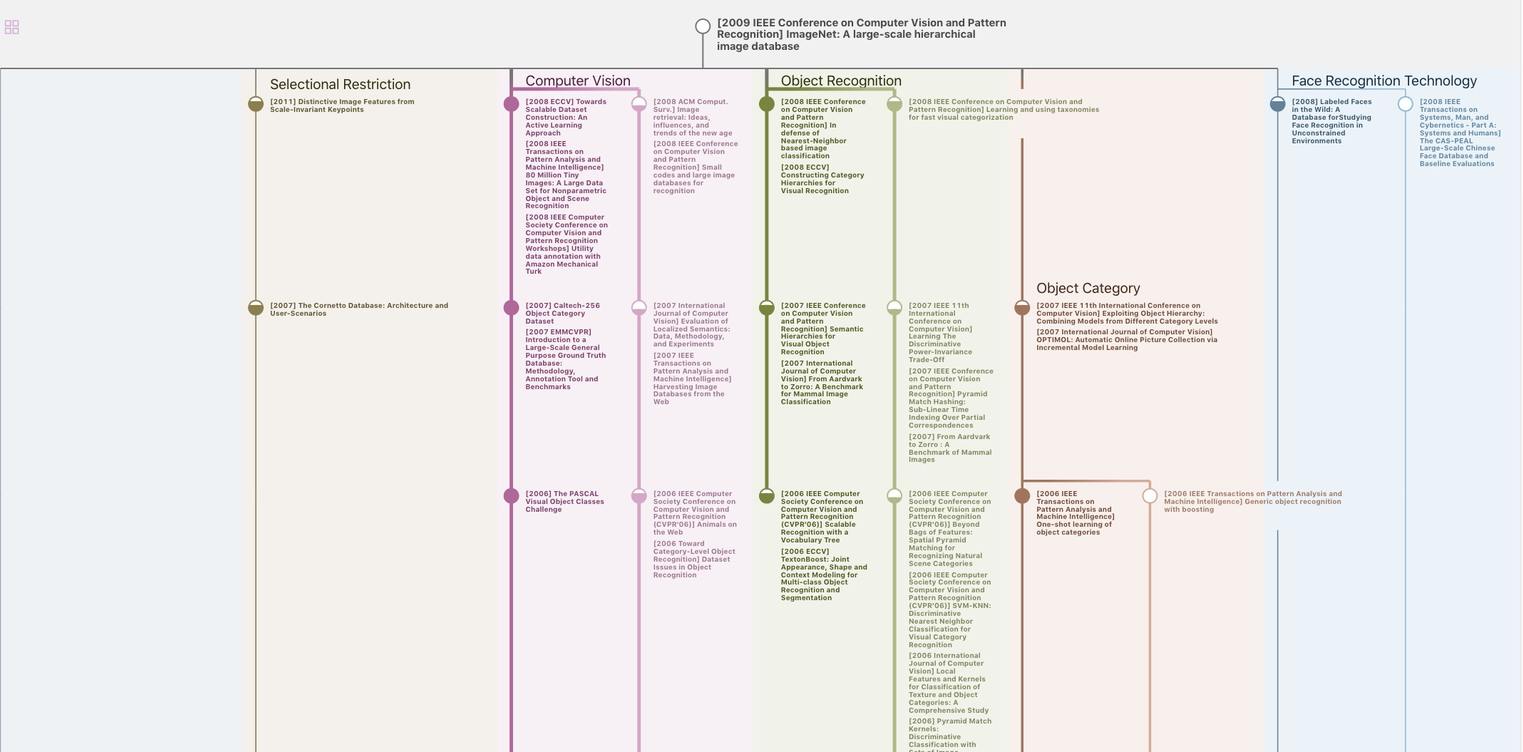
生成溯源树,研究论文发展脉络
Chat Paper
正在生成论文摘要