Deep Reinforcement Learning for Robotic Pushing and Picking in Cluttered Environment
2019 IEEE/RSJ International Conference on Intelligent Robots and Systems (IROS)(2019)
摘要
In this paper, a novel robotic grasping system is established to automatically pick up objects in cluttered scenes. A composite robotic hand composed of a suction cup and a gripper is designed for grasping the object stably. The suction cup is used for lifting the object from the clutter first and the gripper for grasping the object accordingly. We utilize the affordance map to provide pixel-wise lifting point candidates for the suction cup. To obtain a good affordance map, the active exploration mechanism is introduced to the system. An effective metric is designed to calculate the reward for the current affordance map, and a deep Q-Network (DQN) is employed to guide the robotic hand to actively explore the environment until the generated affordance map is suitable for grasping. Experimental results have demonstrated that the proposed robotic grasping system is able to greatly increase the success rate of the robotic grasping in cluttered scenes.
更多查看译文
关键词
novel robotic grasping system,cluttered scenes,composite robotic hand,suction cup,gripper,clutter,pixel-wise lifting point candidates,active exploration mechanism,deep Q-Network,generated affordance map,robotic pushing,cluttered environment,deep reinforcement learning
AI 理解论文
溯源树
样例
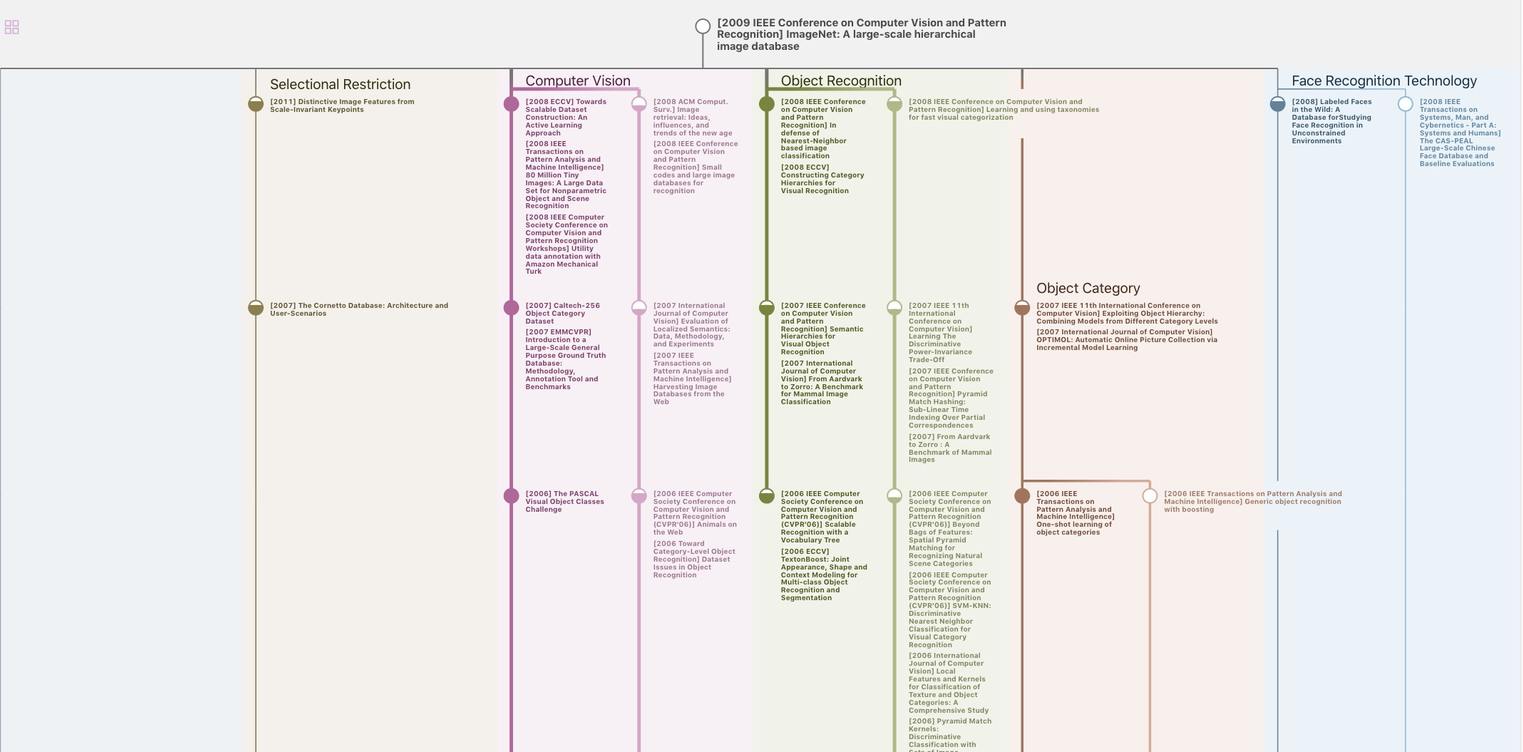
生成溯源树,研究论文发展脉络
Chat Paper
正在生成论文摘要