Attention-Based Hierarchical Deep Reinforcement Learning for Lane Change Behaviors in Autonomous Driving
2019 IEEE/RSJ INTERNATIONAL CONFERENCE ON INTELLIGENT ROBOTS AND SYSTEMS (IROS)(2019)
摘要
Performing safe and efficient lane changes is a crucial feature for creating fully autonomous vehicles. Recent advances have demonstrated successful lane following behavior using deep reinforcement learning, yet the interactions with other vehicles on road for lane changes are rarely considered. In this paper, we design a hierarchical Deep Reinforcement Learning (DRL) algorithm to learn lane change behaviors in dense traffic. By breaking down overall behavior to sub-policies, faster and safer lane change actions can be learned. We also apply temporal and spatial attention to the DRL architecture, which helps the vehicle focus more on surrounding vehicles and leads to smoother lane change behavior. We conduct our experiments in the TORCS simulator and the results outperform the state-of-art deep reinforcement learning algorithm in various lane change scenarios.
更多查看译文
关键词
lane change scenarios,autonomous driving,fully autonomous vehicles,faster lane change actions,safer lane change actions,lane change behavior,attention-based hierarchical deep reinforcement learning,hierarchical DRL algorithm,temporal attention,spatial attention,DRL architecture,TORCS simulator
AI 理解论文
溯源树
样例
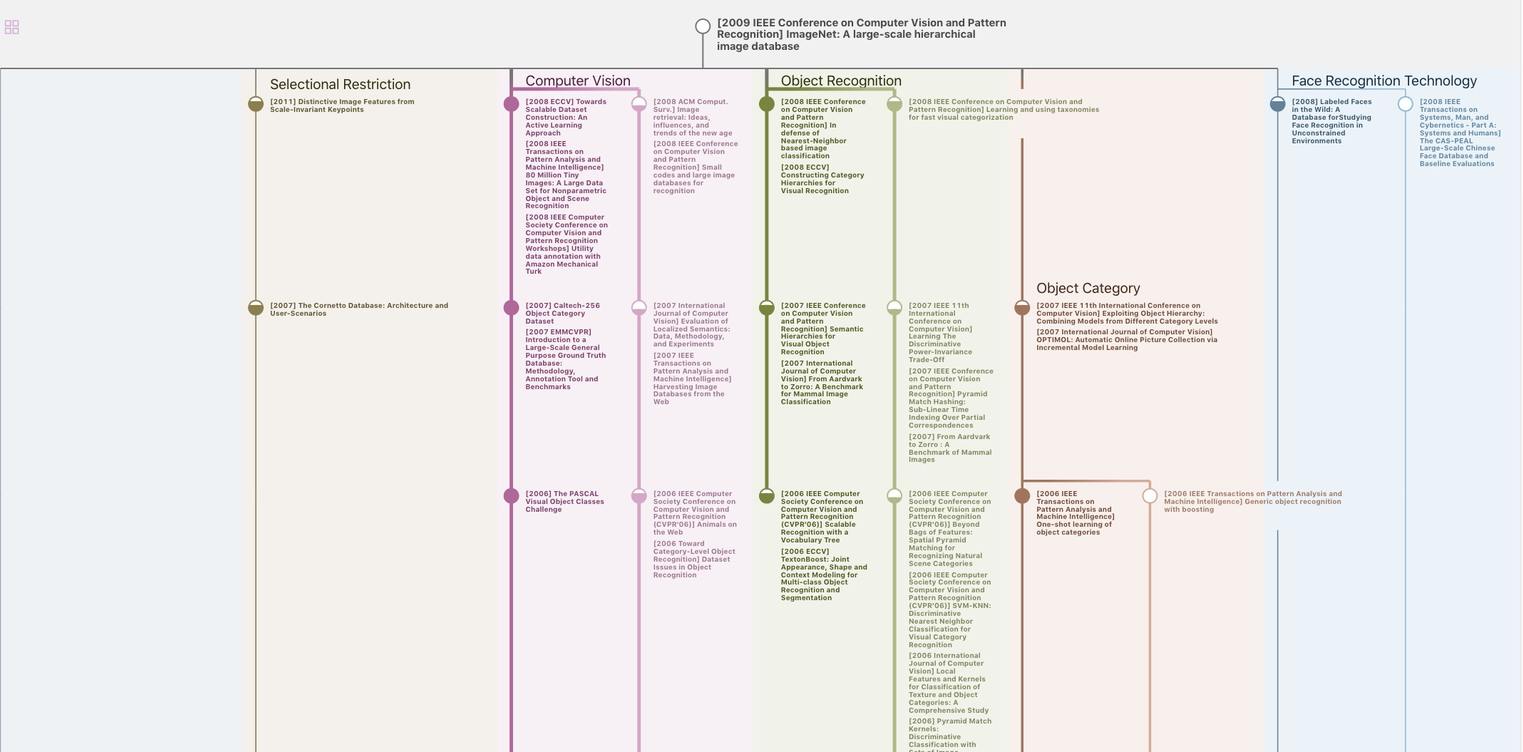
生成溯源树,研究论文发展脉络
Chat Paper
正在生成论文摘要