Multi-Step Pick-And-Place Tasks Using Object-Centric Dense Correspondences
2019 IEEE/RSJ INTERNATIONAL CONFERENCE ON INTELLIGENT ROBOTS AND SYSTEMS (IROS)(2019)
摘要
This paper presents an object-centric method for efficiently performing two types of challenging pick-and-place tasks, namely sequential pick and place and object sorting. We propose multiclass dense object nets (MCDONs) for learning object-centric dense descriptors that maintain not only intraclass variations but also inter-class separation. Intra-class consistency is also inherently learned and is useful for our pick-and-place tasks. All the tasks only require a single demonstration from users, which can then be generalized to all class instances. A dataset containing eight classes and a total of 52 objects was provided in this study. We obtained a task success rate of 93.33% on a five-block stacking task and 97.41% on a three-class object sorting task.
更多查看译文
关键词
inter-class separation,intra-class consistency,pick-and-place tasks,task success rate,three-class object sorting task,object-centric dense correspondences,object-centric method,sequential pick,multiclass dense object nets,object-centric dense descriptors,intra-class variations
AI 理解论文
溯源树
样例
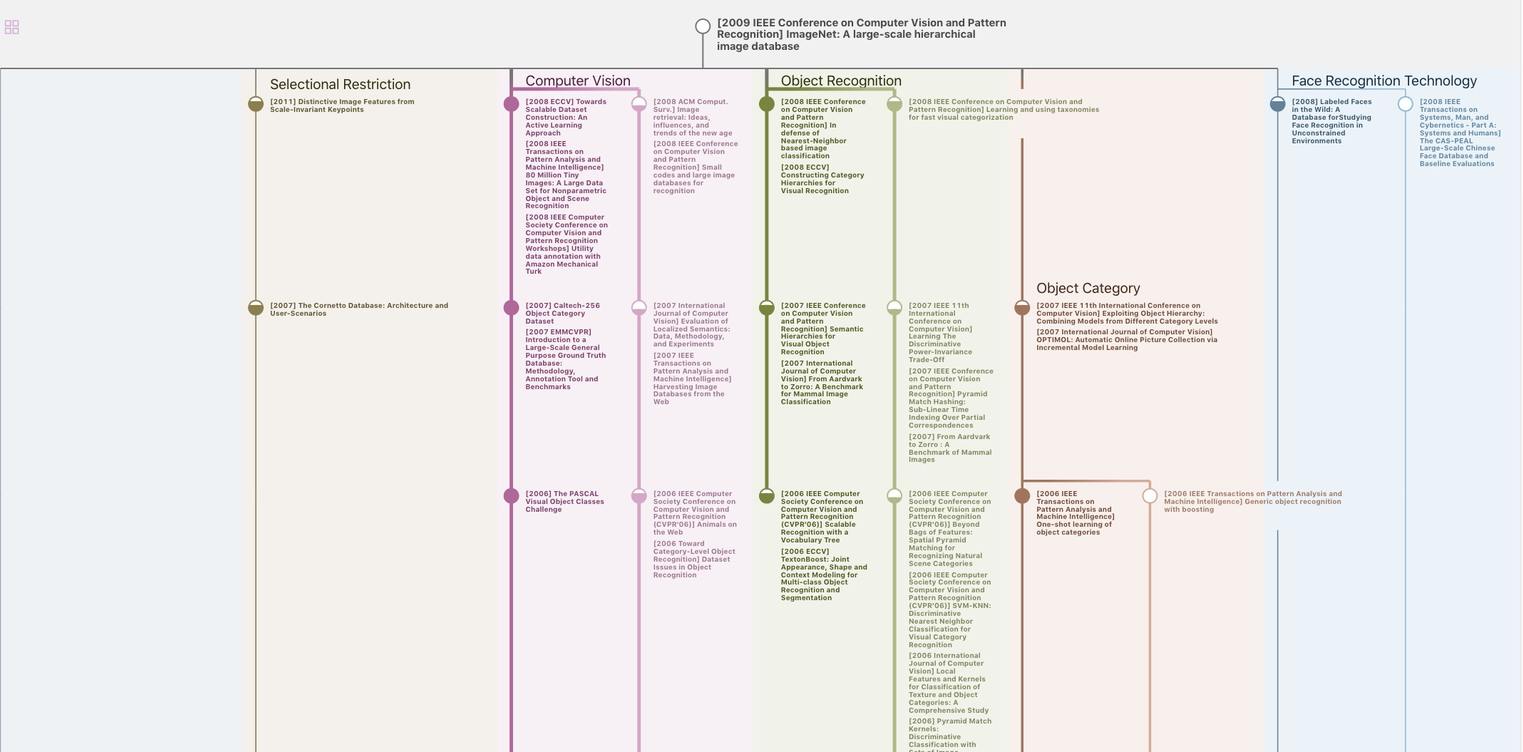
生成溯源树,研究论文发展脉络
Chat Paper
正在生成论文摘要