An Efficient Machine Learning Method to Solve Imbalanced Data in Metabolic Disease Prediction
2019 11th International Conference on Knowledge and Systems Engineering (KSE)(2019)
摘要
The increase of obesity, its related diseases and the high incidence of metabolic diseases as a whole, constitute a major public health problem on a global scale. New strategies that allow for the discovery of novel metabolic disease-related genes are necessary to develop new treatments. In this paper, we proposed an efficient method to predict metabolic disease genes, solving the problem of imbalanced data. The method combined protein-protein interactions and miRNA-target interactions to construct integrated networks, whose topological properties can be used as features to train machine learning classifiers. We applied different strategies to optimize imbalanced class. The best model of gradient boosting achieved a significant F1-score of 0.82. When testing the model with non-disease genes, we predicted 549 candidates, out of which 123 were validated indirectly from literature to be related to metabolic diseases. The remaining genes' functions were investigated by gene enrichment analysis, revealing their association with diseases known to co-occur with metabolic diseases, such as cancer and cardiovascular conditions. These results indicated that this method contributed to the identification of novel metabolic disease-related genes.
更多查看译文
关键词
metabolic disease,protein-protein interaction network,miRNA-target interaction,machine learning,disease gene prediction,imbalanced data.
AI 理解论文
溯源树
样例
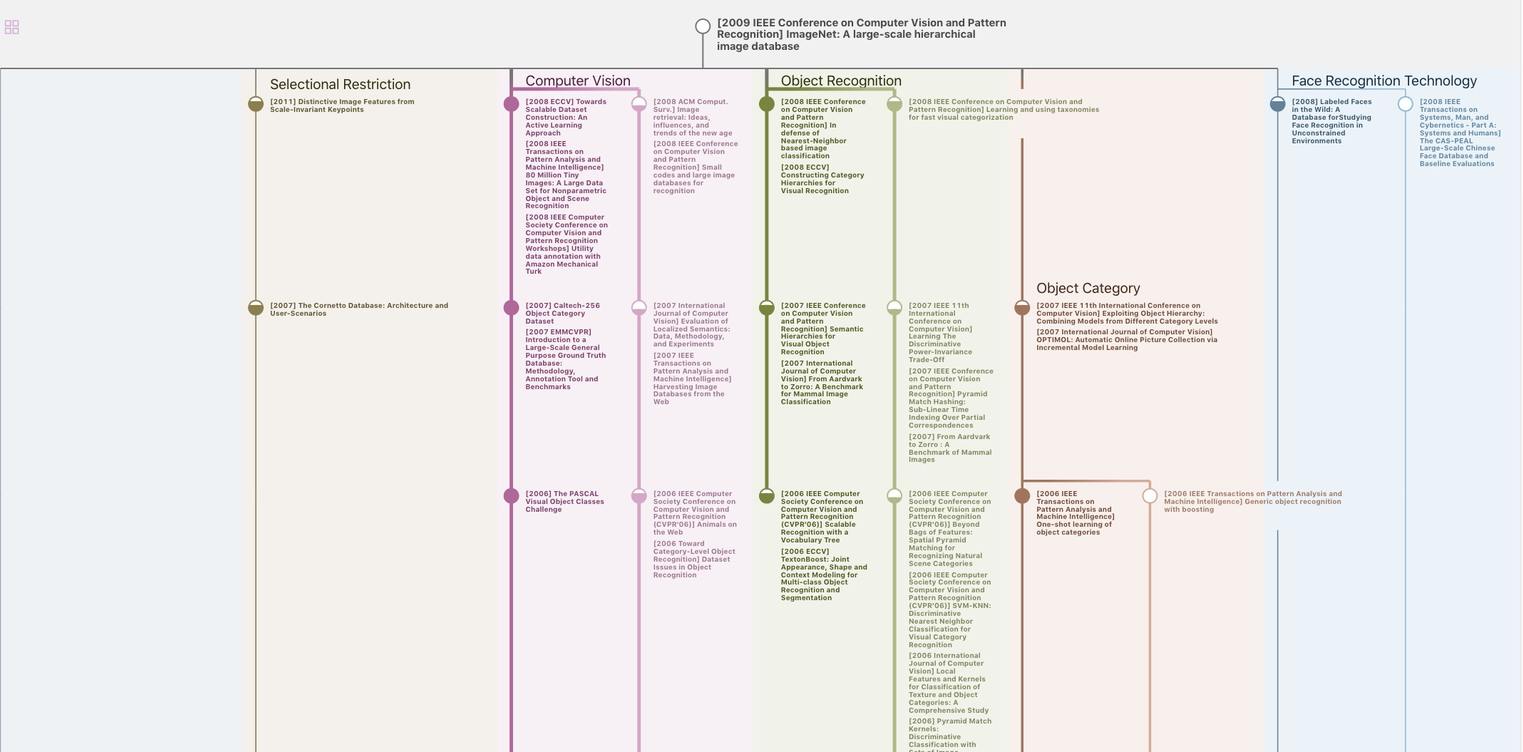
生成溯源树,研究论文发展脉络
Chat Paper
正在生成论文摘要