Deep Reinforcement Learning for Backscatter-Aided Data Offloading in Mobile Edge Computing
IEEE Network(2020)
摘要
Wireless network optimization has been becoming very challenging as the problem size and complexity increase tremendously, due to close couplings among network entities with heterogeneous service and resource requirements. By continuously interacting with the environment, DRL provides a mechanism for different network entities to build knowledge and make autonomous decisions to improve network performance. In this article, we first review typical DRL approaches and recent enhancements. We then discuss the applications of DRL for mobile edge computing (MEC), which can be used for user devices to offload computation workload to MEC servers. However, for the low-power user devices, for example, wireless sensors, MEC can be costly as data offloading also consumes high power in RF communications. To balance the energy consumption in local computation and data offloading, we propose a novel hybrid offloading model that exploits the complementary operations of active RF communications and low-power backscatter communications. To maximize the energy efficiency in MEC offloading, the DRL framework is customized to learn the optimal transmission scheduling and workload allocation in two communications technologies. Numerical results show that the hybrid offloading scheme can improve the energy efficiency over 20 percent compared to existing schemes.
更多查看译文
关键词
Reinforcement learning,Optimization,Servers,Radio frequency,Resource management,Training,Wireless networks
AI 理解论文
溯源树
样例
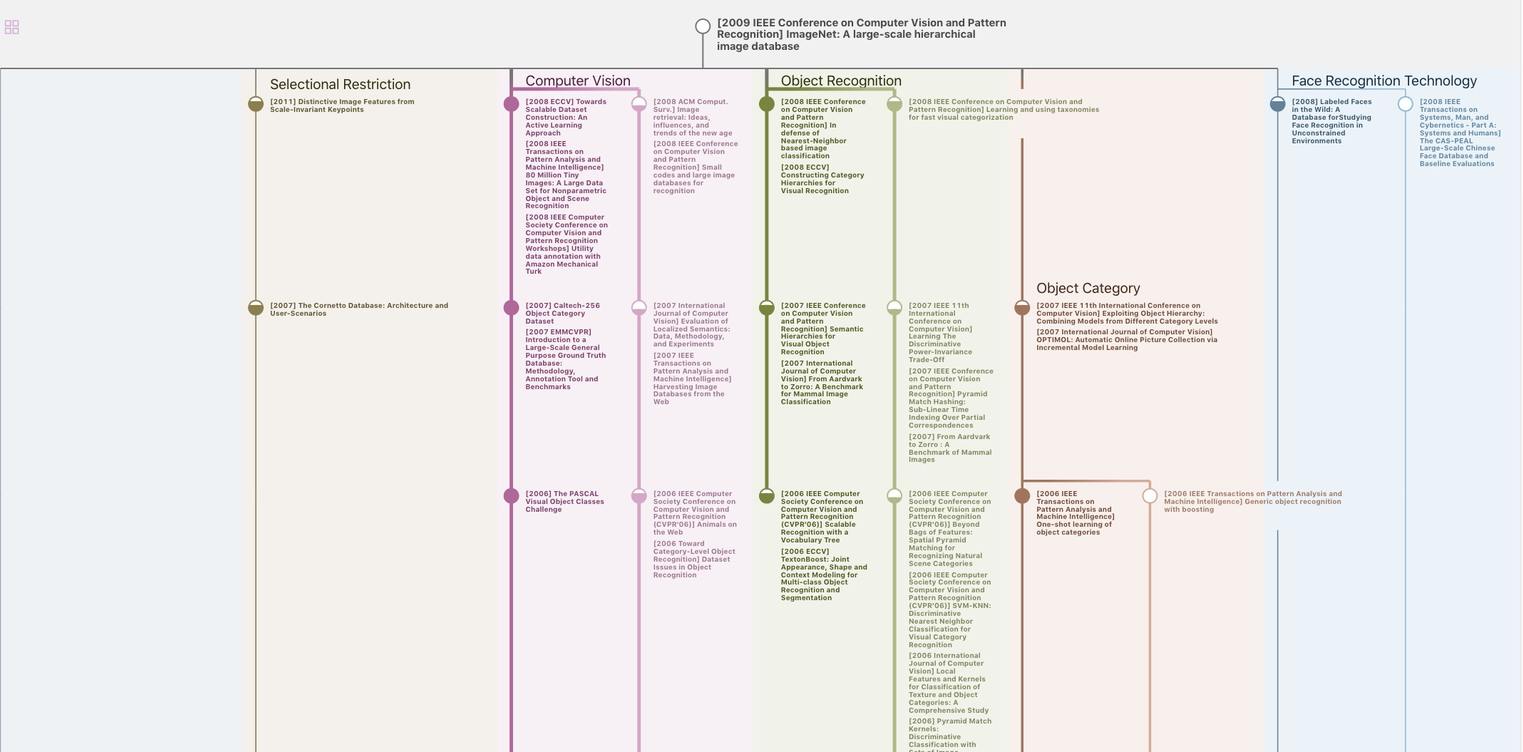
生成溯源树,研究论文发展脉络
Chat Paper
正在生成论文摘要