Limits on Gradient Compression for Stochastic Optimization
ISIT(2020)
摘要
We consider stochastic optimization overl p spaces using access to a first-order oracle. We ask: What is the minimum precision required for oracle outputs to retain the unrestricted convergence ratesƒ We characterize this precision for every p≥ 1 by deriving information theoretic lower bounds and by providing quantizers that (almost) achieve these lower bounds. Our quantizers are new and easy to implement. In particular, our results are exact for p = 2 and p = ∞, showing the minimum precision needed in these settings are Θ(d) and Θ(log d), respectively. The latter result is surprising since recovering the gradient vector will require Ω(d) bits.
更多查看译文
关键词
gradient compression,stochastic optimization,first-order oracle,minimum precision,unrestricted convergence rates,lower bounds,quantizers,gradient vector
AI 理解论文
溯源树
样例
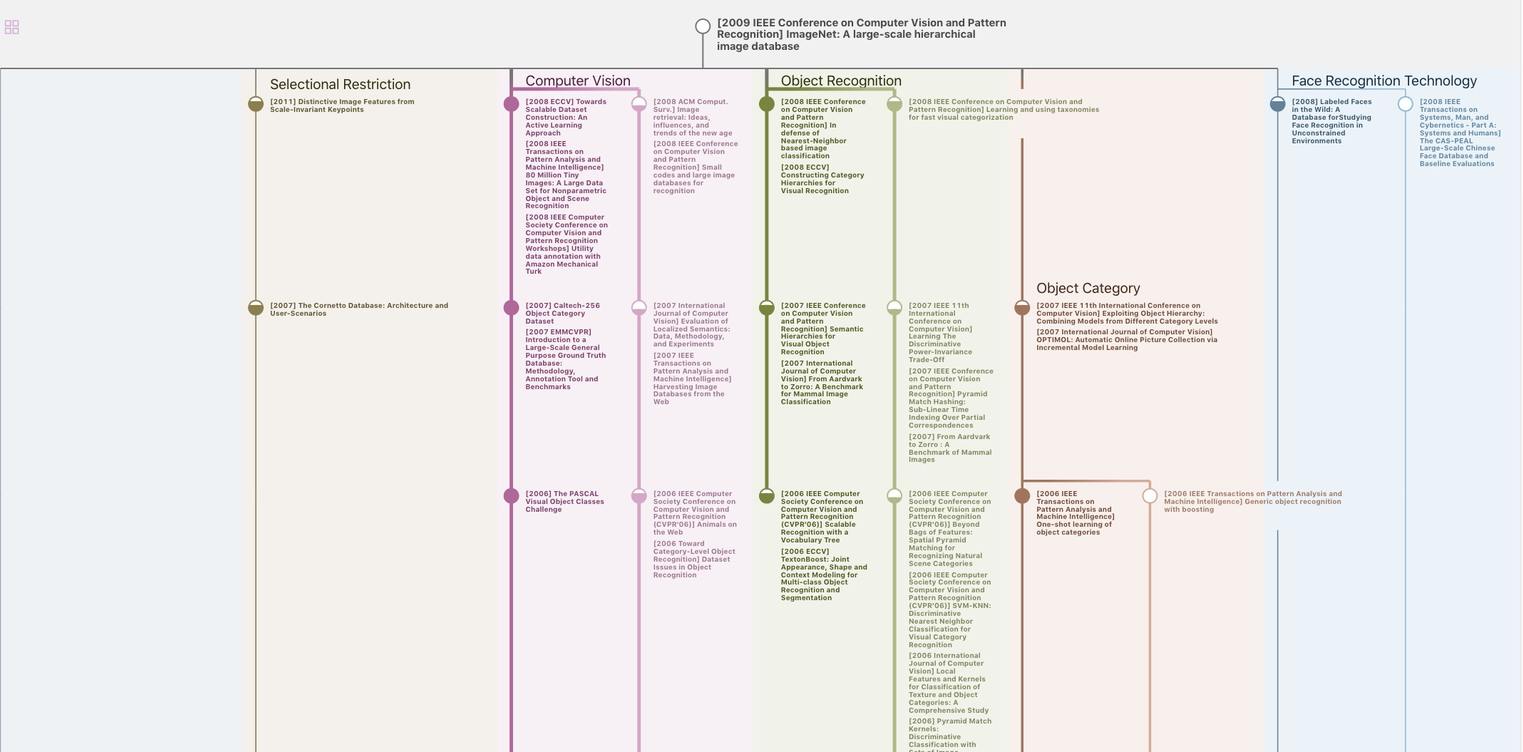
生成溯源树,研究论文发展脉络
Chat Paper
正在生成论文摘要