Proactive Fiber Break Detection Based On Quaternion Time Series And Automatic Variable Selection From Relational Data
ADVANCED ANALYTICS AND LEARNING ON TEMPORAL DATA, AALTD 2019(2019)
摘要
We address the problem of event classification for proactive fiber break detection in high-speed optical communication systems. The proposed approach is based on monitoring the State of Polarization (SOP) via digital signal processing in a coherent receiver. We describe in details the design of a classifier providing interpretable decision rules and enabling low-complexity real-time detection embedded in network elements. The proposed method operates on SOP time series, which define trajectories on the 3D sphere; SOP time series are low-pass filtered (to reduce measurement noise), pre-rotated (to provide invariance to the starting point of trajectories) and converted to quaternion domain. Then quaternion sequences are recoded to relational data for automatic variable construction and selection. We show that a naive Bayes classifier using a limited subset of variables can achieve an event classification accuracy of more than 99% for the tested conditions.
更多查看译文
关键词
quaternion time series,fiber,automatic variable selection
AI 理解论文
溯源树
样例
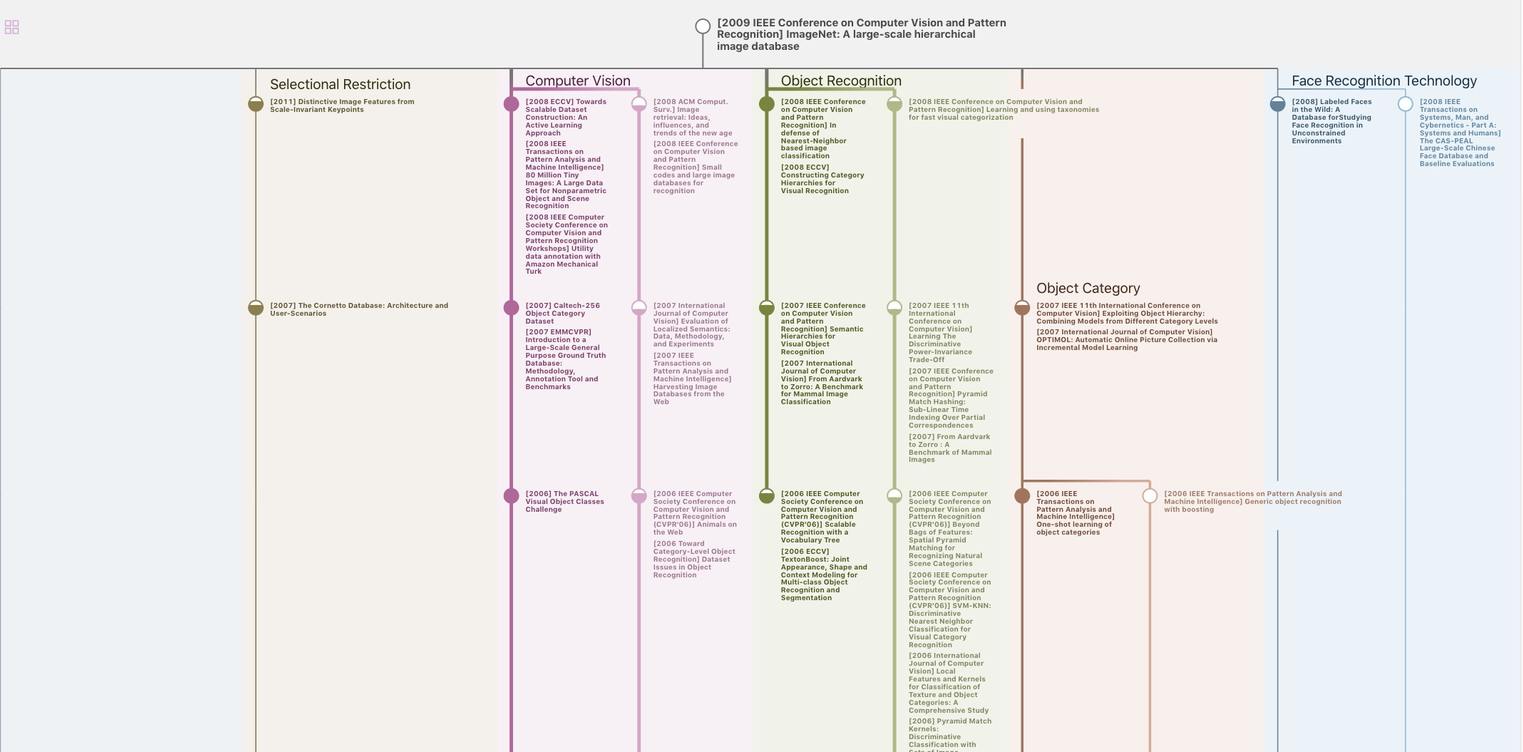
生成溯源树,研究论文发展脉络
Chat Paper
正在生成论文摘要