A Small-Footprint End-to-End KWS System in Low Resources.
SPML(2019)
摘要
In this paper, we propose an efficient end-to-end architecture, based on Connectionist Temporal Classification (CTC), for low-resource small-footprint keyword spotting (KWS) system. For a low-resource KWS system, it is difficult for the network to thoroughly learn the features of keywords. The intuition behind our new model is that a priori information of the keyword is available. In contrast to the conventional KWS system, we modify the label set by adding the preset keyword(s) to the original label set to enhance the learning performance and optimize the final detection task of the system. Besides, CTC is applied to address the sequential alignment problem. We employ GRU as the encoding layer in our system because of the dataset small. Experiments using the WSJ0 dataset show that the proposed KWS system is significantly more accurate than the baseline system. Compared to the character-level-only KWS system, the proposed system can obviously improve the performance. Furthermore, the improved system works well in terms of low resource conditions, especially for long words.
更多查看译文
AI 理解论文
溯源树
样例
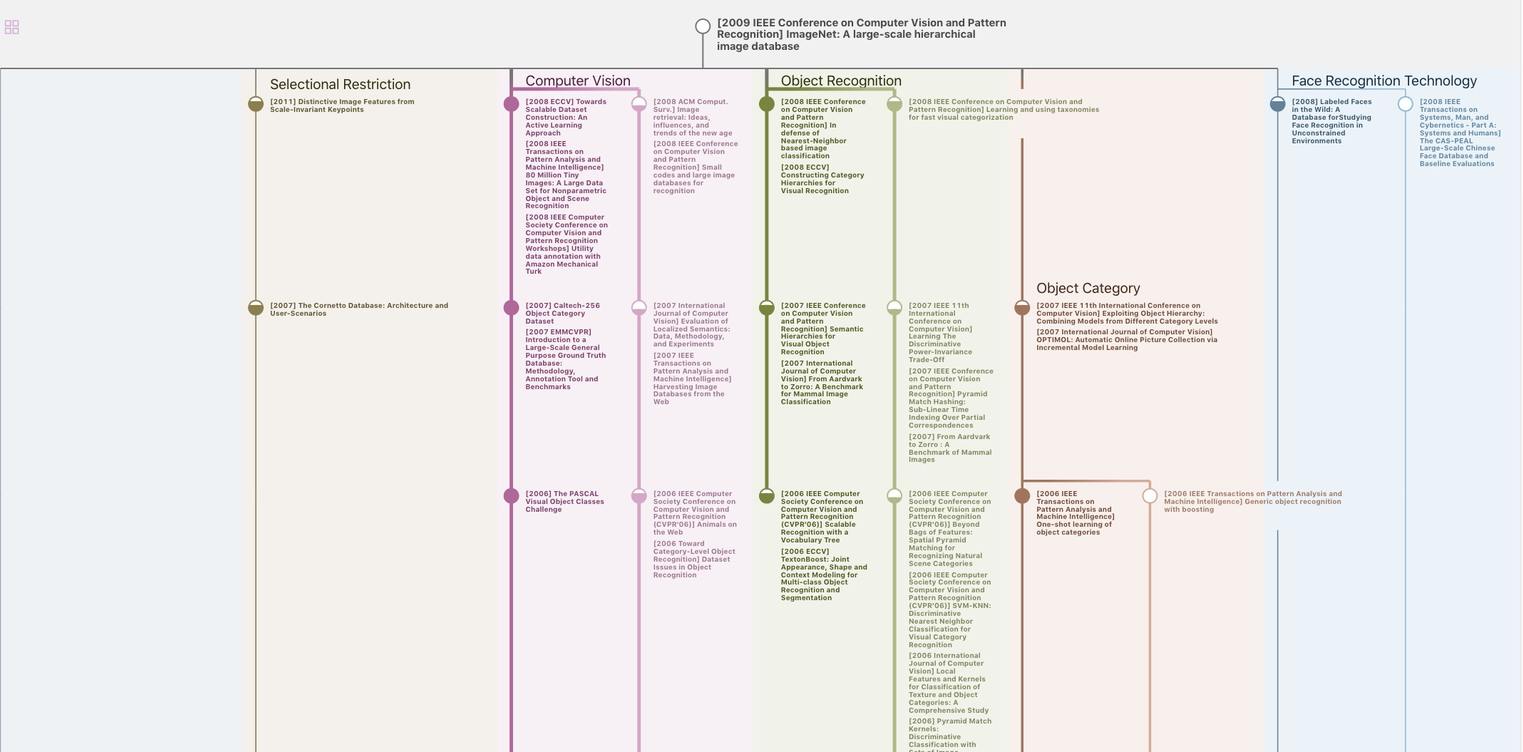
生成溯源树,研究论文发展脉络
Chat Paper
正在生成论文摘要