Poi Recommendation Based On Locality-Specific Seasonality And Long-Term Trends
SOFSEM 2020: THEORY AND PRACTICE OF COMPUTER SCIENCE(2020)
摘要
This work deals with time-aware recommender systems in a domain of location-based social networks, such as Yelp or Foursquare. We propose a novel method to recommend Points of Interest (POIs) which considers their yearly seasonality and long-term trends. In contrast to the existing methods, we model these temporal aspects specifically for individual geographical localities instead of globally. According to the results achieved by the experimental evaluation on Yelp dataset, locality-specific seasonality can significantly improve the recommendation performance in comparison to its global alternative. We found out that it is helpful mostly within recommendations for highly-active users (it has a smaller influence for the novice users) and as expected, in localities with a strong seasonal weather variation. Another interesting finding is that in contrast to seasonality, we did not observe an improvement in case of locality-specific long-term trends.
更多查看译文
关键词
Recommender systems, Points of interest, Time-aware recommendation, Seasonality, Long-term trends
AI 理解论文
溯源树
样例
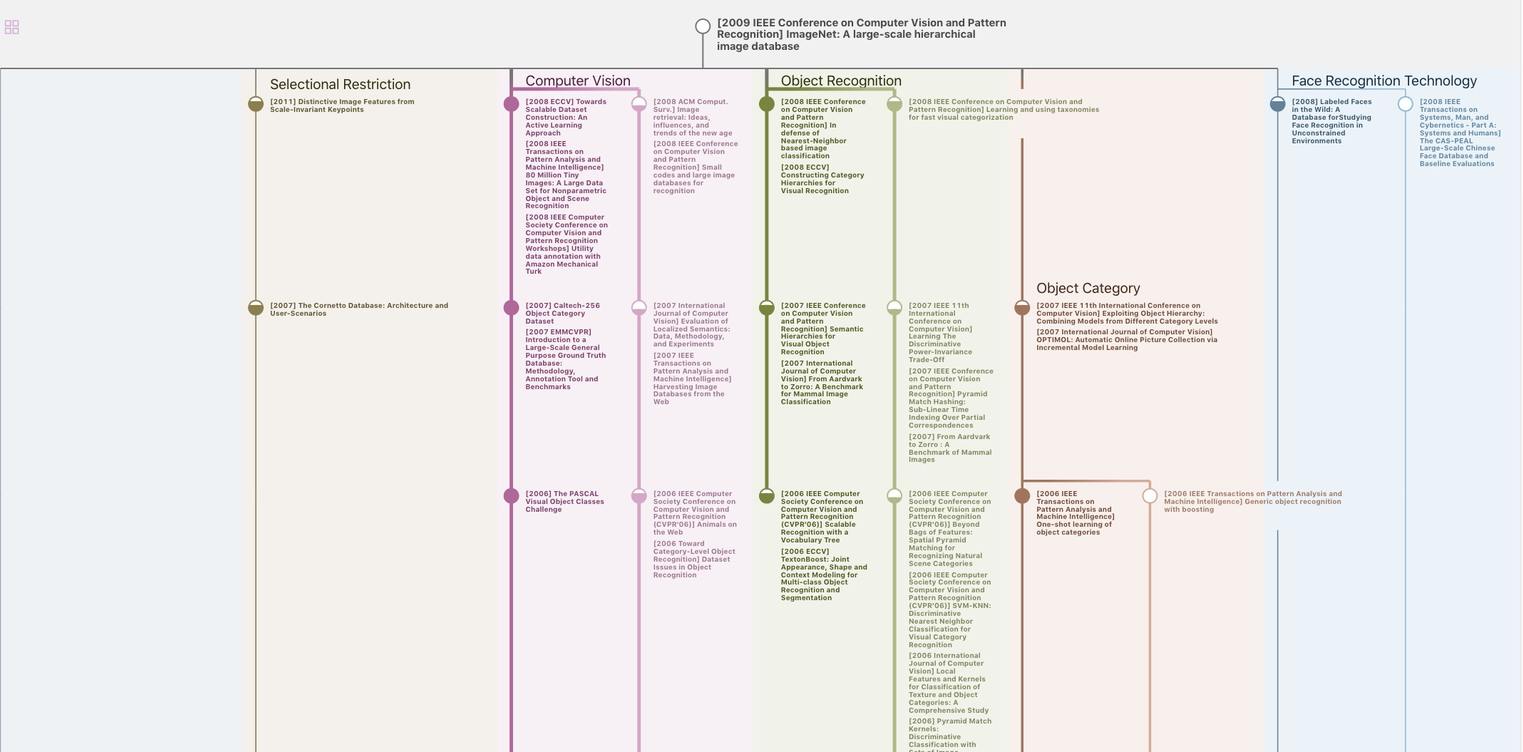
生成溯源树,研究论文发展脉络
Chat Paper
正在生成论文摘要