Adversarial Graph Attention Network for Multi-modal Cross-Modal Retrieval
2019 IEEE International Conference on Big Knowledge (ICBK)(2019)
Abstract
Existing cross-modal retrieval methods are mainly constrained to the bimodal case. When applied to the multi-modal case, we need to train O(K^2) (K: number of modalities) separate models, which is inefficient and unable to exploit common information among multiple modalities. Though some studies focused on learning a common space of multiple modalities for retrieval, they assumed data to be i.i.d. and failed to learn the underlying semantic structure which could be important for retrieval. To tackle this issue, we propose an extensive Adversarial Graph Attention Network for Multi-modal Cross-modal Retrieval (AGAT). AGAT synthesizes a self-attention network (SAT), a graph attention network (GAT) and a multi-modal generative adversarial network (MGAN). The SAT generates high-level embeddings for data items from different modalities, with self-attention capturing feature-level correlations in each modality. The GAT then uses attention to aggregate embeddings of matched items from different modalities to build a common embedding space. The MGAN aims to "cluster" matched embeddings of different modalities in the common space by forcing them to be similar to the aggregation. Finally, we train the common space so that it captures the semantic structure by constraining within-class/between-class distances. Experiments on three datasets show the effectiveness of AGAT.
MoreTranslated text
Key words
Cross-modal retrieval,graph attention,self attention,generative adversarial network
AI Read Science
Must-Reading Tree
Example
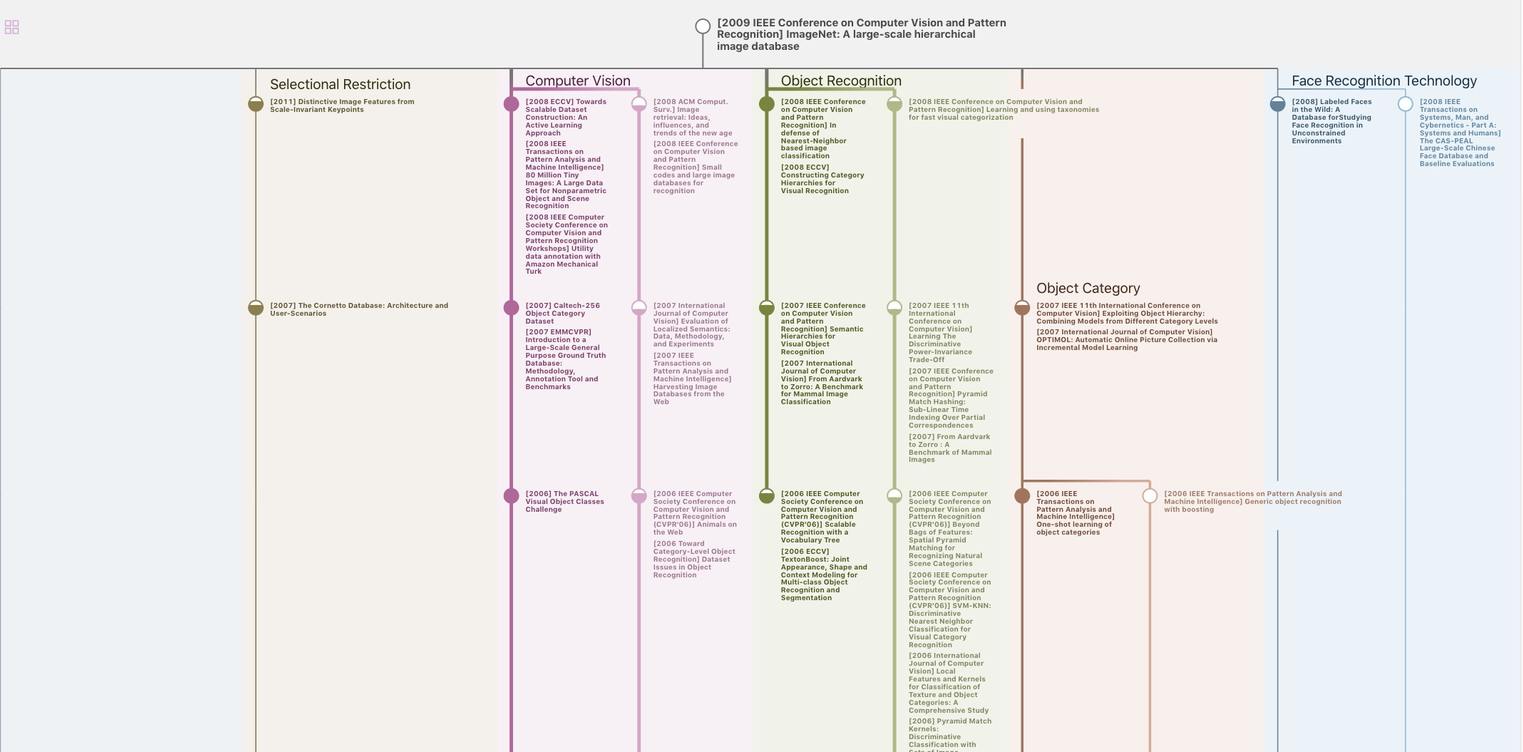
Generate MRT to find the research sequence of this paper
Chat Paper
Summary is being generated by the instructions you defined