Motor Bearing Fault Diagnosis Based on Deep Learning
2019 20th IEEE/ACIS International Conference on Software Engineering, Artificial Intelligence, Networking and Parallel/Distributed Computing (SNPD)(2019)
摘要
The effective fault monitoring of the motor bearings not only can ensure the smooth and efficient operation of equipment, but also can detect and eliminate the running fault in time to prevent major accidents. Based on deep learning algorithm, this paper constructs a stacked auto-encoder (SAE) network. The input data are compressed by introduced sparsity constraint, so that the network can accurately extract the fault characteristics of the input data. And the fault recognition ability of the network can be improved by introducing random noise to reconstruct input data. The simulation result shows that the SAE network can not only overcome the shortcomings of traditional fault diagnosis methods that requires personnel to distinguish fault samples and needs a large number of prior knowledge; but also realize the self-learning of fault feature information. The accuracy rates of fault identification reach 98%, 94%, 96% and 95.5% in four different working conditions. What’s more, the network can adapt to the actual multi-load cases, demonstrate strong robustness under different working conditions.
更多查看译文
关键词
fault diagnosis,deep learning,stacked auto-encoder,sparsity constraint,data reconstruction
AI 理解论文
溯源树
样例
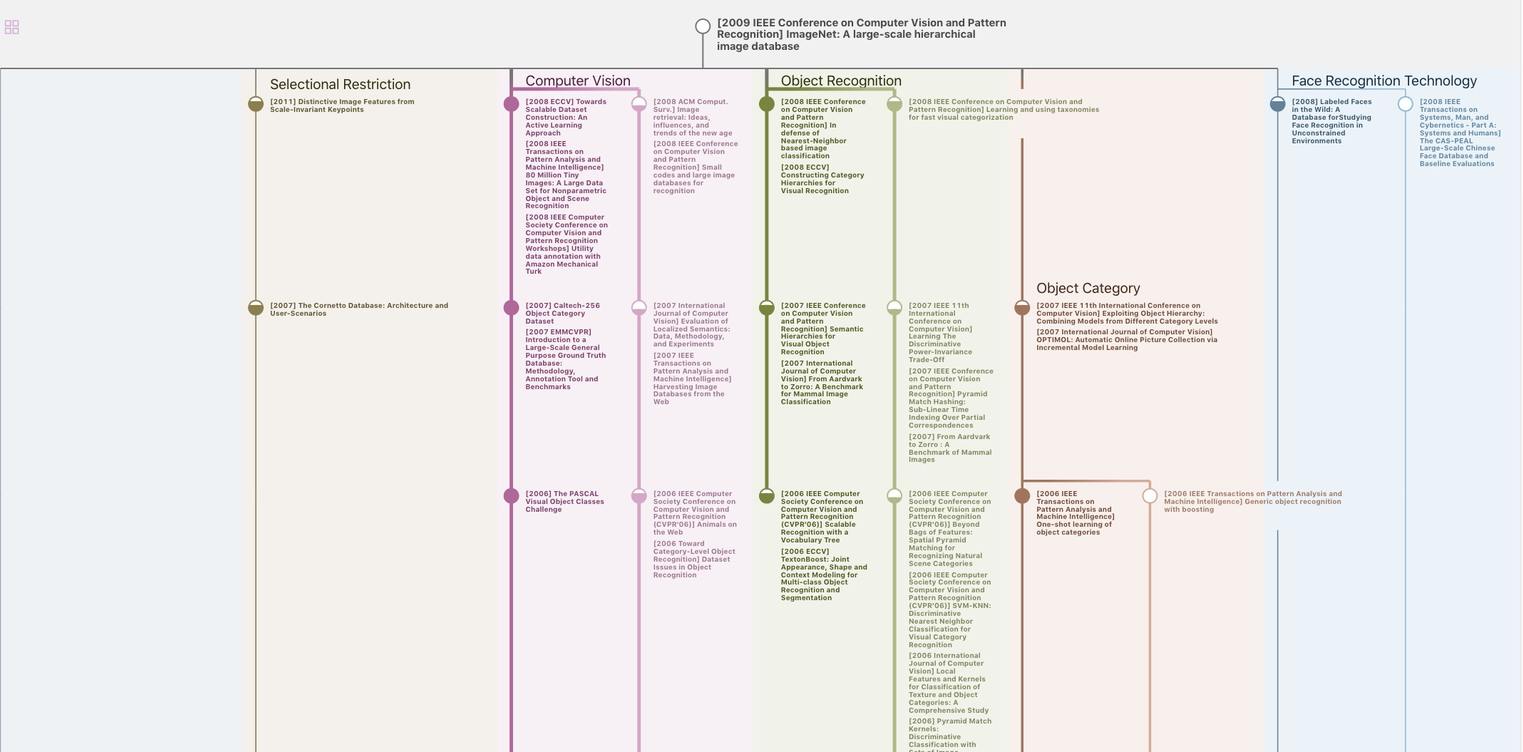
生成溯源树,研究论文发展脉络
Chat Paper
正在生成论文摘要