Personalized Review-Oriented Explanations For Recommender Systems
WEB INFORMATION SYSTEMS AND TECHNOLOGIES (WEBIST 2018)(2019)
摘要
Explainable recommender systems aim to provide clear interpretations to a user regarding the recommended list of items. The explanations present different formats to justify the recommended list of items such as images, graphs or text. We propose to use review-oriented explanations to help users in their decision since we can find crucial detailed feature in the reviews given by users. The model uses advances of natural language processing and incorporates the helpfulness score given in previous reviews to explain the recommended list of items provided by a latent factor model prediction. We conducted empirical experiments in the Yelp and Amazon datasets, proving that our model improves the quality of the explanations. The model outperforms baselines models by 13% for NDCG@5, 83% for HitRatio@5, 13% for NDCG@10, and 55% for HitRatio@10 in the Yelp dataset. For the Amazon dataset, the observed improvement was 9% for NDCG@5, 83% for HitRatio@5, 9% for NDCG@10, and 22% for HitRatio@10.
更多查看译文
关键词
Explainability, Recommender systems, Matrix factorization
AI 理解论文
溯源树
样例
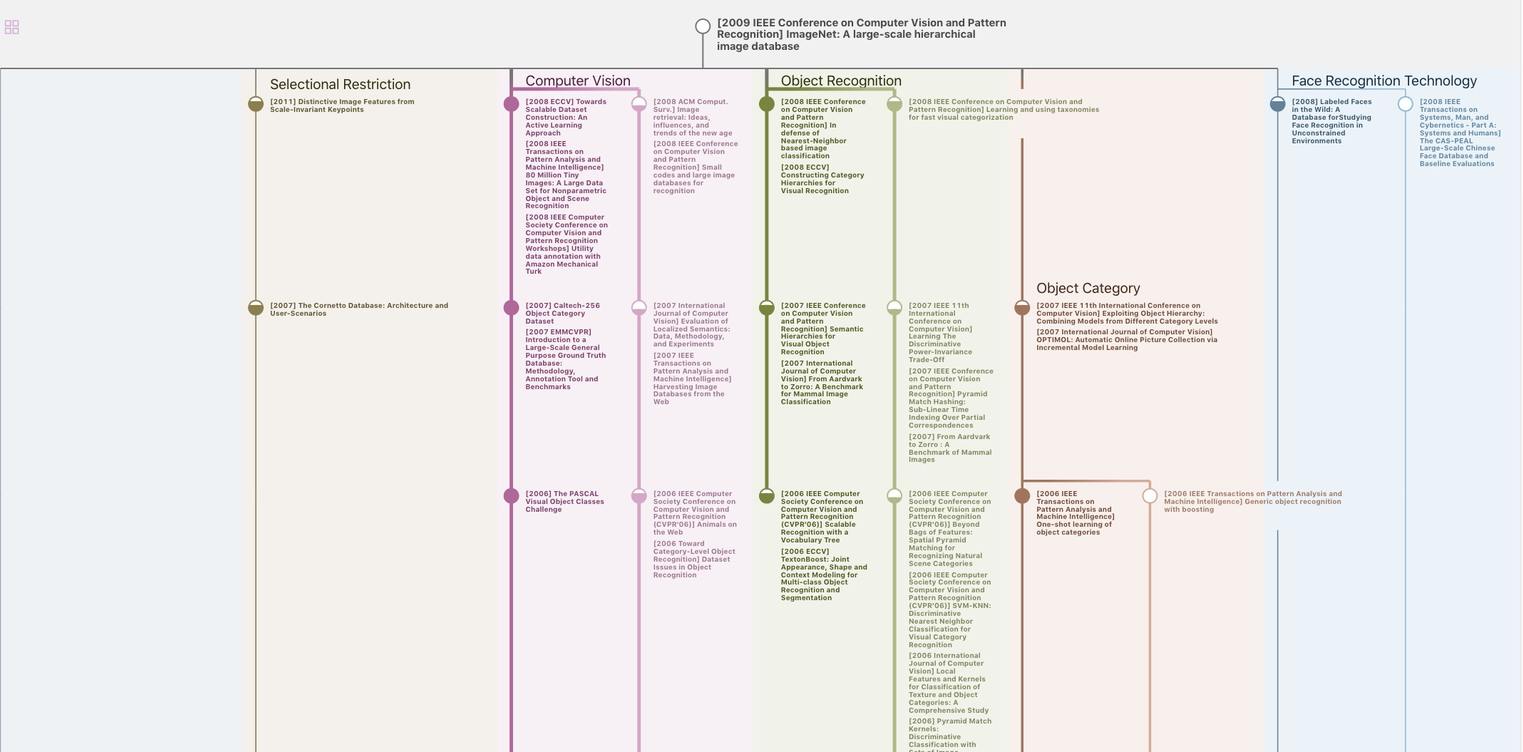
生成溯源树,研究论文发展脉络
Chat Paper
正在生成论文摘要