Sanity-Check: Boosting the Reliability of Safety-Critical Deep Neural Network Applications
2019 IEEE 28th Asian Test Symposium (ATS)(2019)
摘要
The widespread usage of deep neural networks in autonomous driving necessitates a consideration of the safety arguments against hardware-level faults. This study confirms the possible catastrophic impact of hardware-level faults on DNN accuracy; the consequent need for low-cost fault tolerance methods can be met through a rigorous exploration of the mathematical properties of the associated computations. We propose Sanity-Check, which makes use of the linearity property and employs spatial and temporal checksums to protect fully-connected and convolutional layers in deep neural networks. Sanity-Check can be purely implemented on software and deployed on different execution platforms with no additional modification. We also propose Sanity-Check hardware which integrates seamlessly with modern DNN accelerators and neutralizes the small performance overhead in pure software implementations. Sanity-Check delivers perfect error-caused misprediction coverage in our experiments, which makes it a promising candidate for boosting the reliability of safety-critical deep neural network applications.
更多查看译文
关键词
deep neural networks,fault tolerance,reliability,autonomous driving
AI 理解论文
溯源树
样例
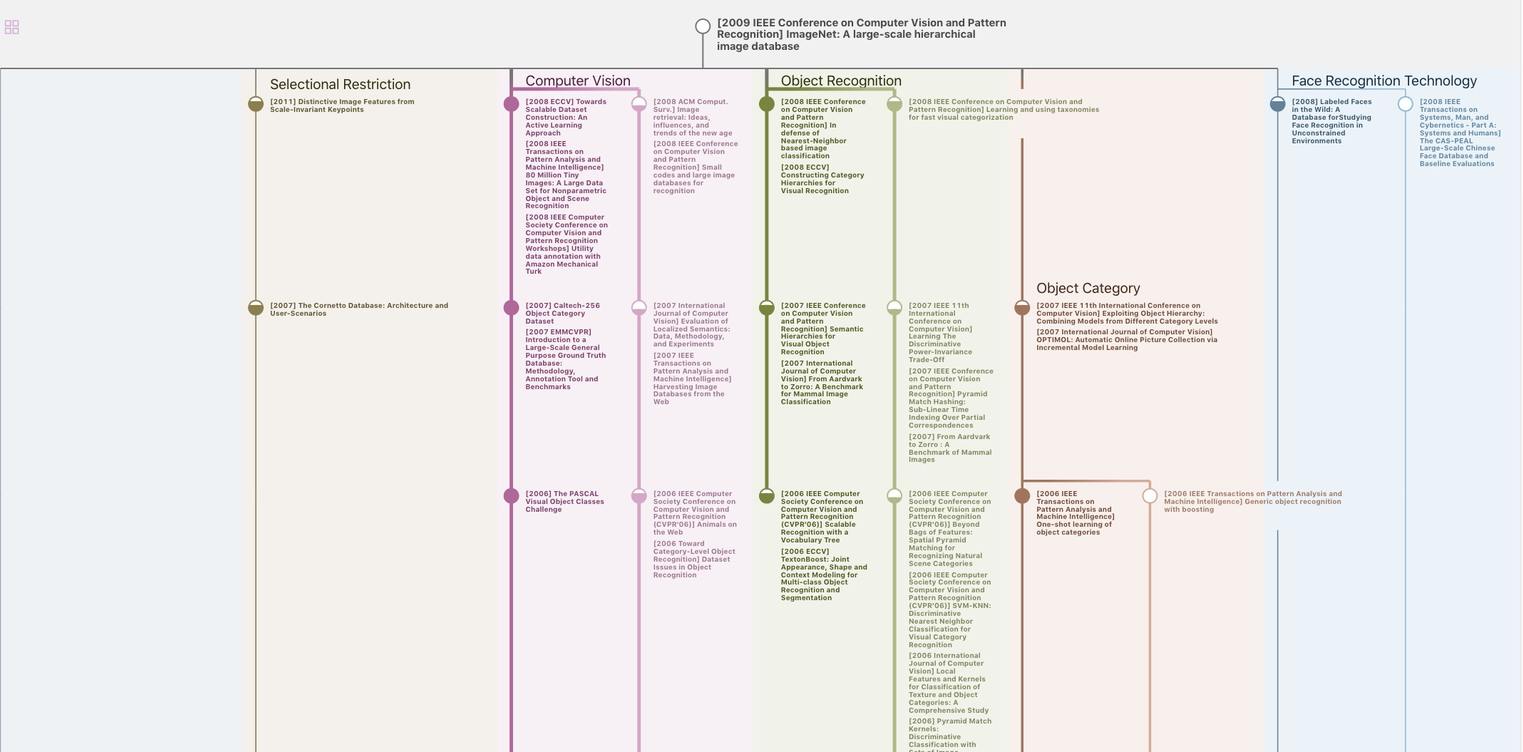
生成溯源树,研究论文发展脉络
Chat Paper
正在生成论文摘要