Reweighted L(1) Algorithm For Robust Principal Component Analysis
ADVANCED COMPUTATIONAL METHODS FOR KNOWLEDGE ENGINEERING (ICCSAMA 2019)(2020)
摘要
In this work, we consider the Robust Principal Components Analysis, a popular method of dimensionality reduction. The corresponding optimization involves the minimization of l(0)-norm which is known to be NP-hard. To deal with this problem, we replace the l(0)-norm by a non-convex approximation, namely capped l(1)DD-norm. The resulting optimization problem is non-convex for which we develop a reweighted l(1) based algorithm. Numerical experiments on several synthetic datasets illustrate the efficiency of our algorithm and its superiority comparing to several state-of-the-art algorithms.
更多查看译文
关键词
Robust principal component analysis, Sparse optimization, Non-convex optimization, Reweighted-l(1)
AI 理解论文
溯源树
样例
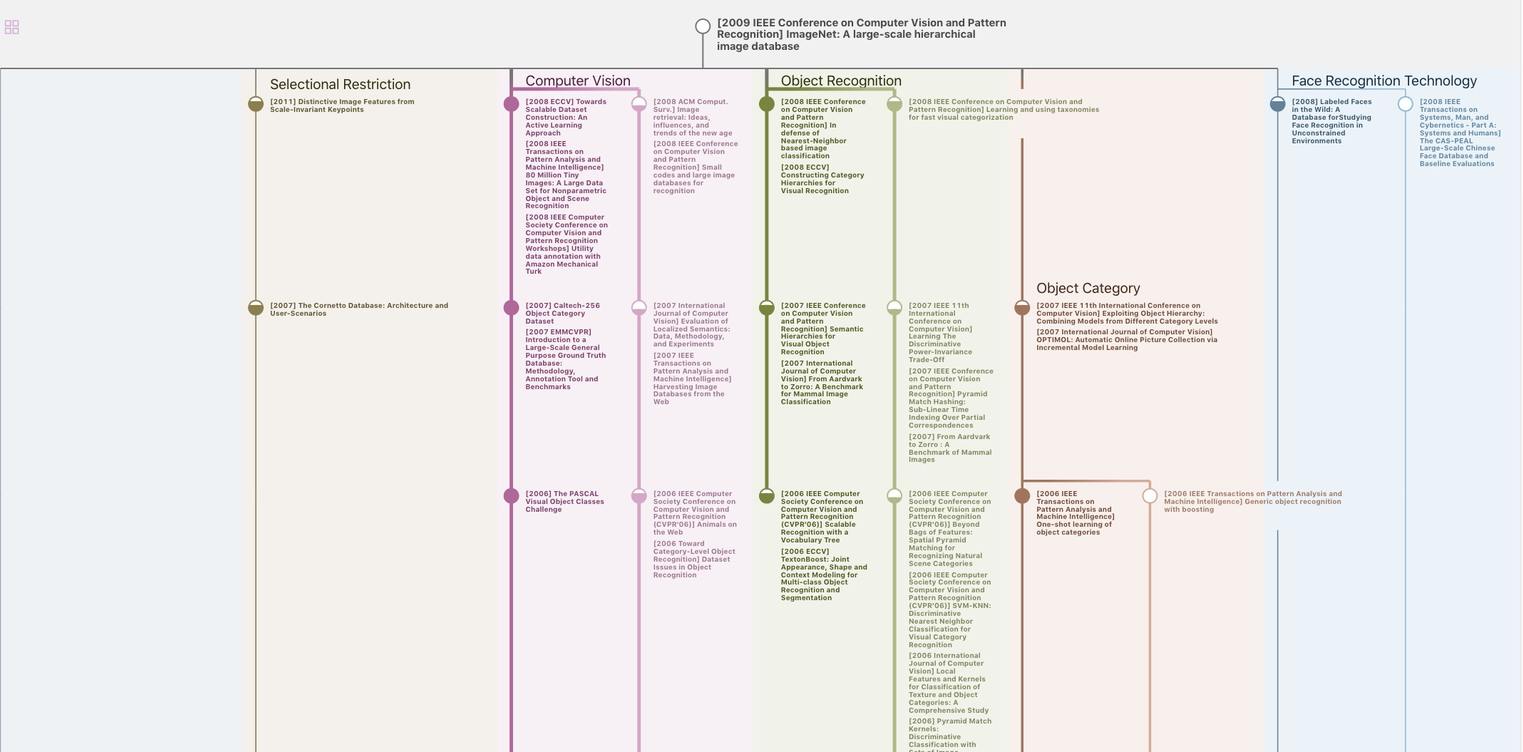
生成溯源树,研究论文发展脉络
Chat Paper
正在生成论文摘要