Flexible compression of big data.
ASONAM '19: International Conference on Advances in Social Networks Analysis and Mining Vancouver British Columbia Canada August, 2019(2019)
摘要
High volumes of valuable data and information can be easily collected in the current era of big data. As rich and constant sources of big data, an incredible amount of people from different social stratum take part in social networks. Hence, social networks are desired for many research topics. In social networks, users (or social entities) are often linked by some 'following' relationships. As the social networks growing, some famous users account (or social entities) might be followed by a large number of same other users. In this situation, we call those famous users as frequently followed groups, which some researchers (or businesses) may be interested in them for investigating. However, the discovery of those frequently followed groups might be difficult and challenging because the following data in social networks are usually very big but sparse (huge number of users lead to big 'following' data, but each user is likely only following a small number of other users). As a result, in this paper, we present a new compression model, which can be used during mining these very big but sparse social networks for discovering the frequently followed groups of users/social entities.
更多查看译文
关键词
big data, social networks, social network 'following' patterns mining
AI 理解论文
溯源树
样例
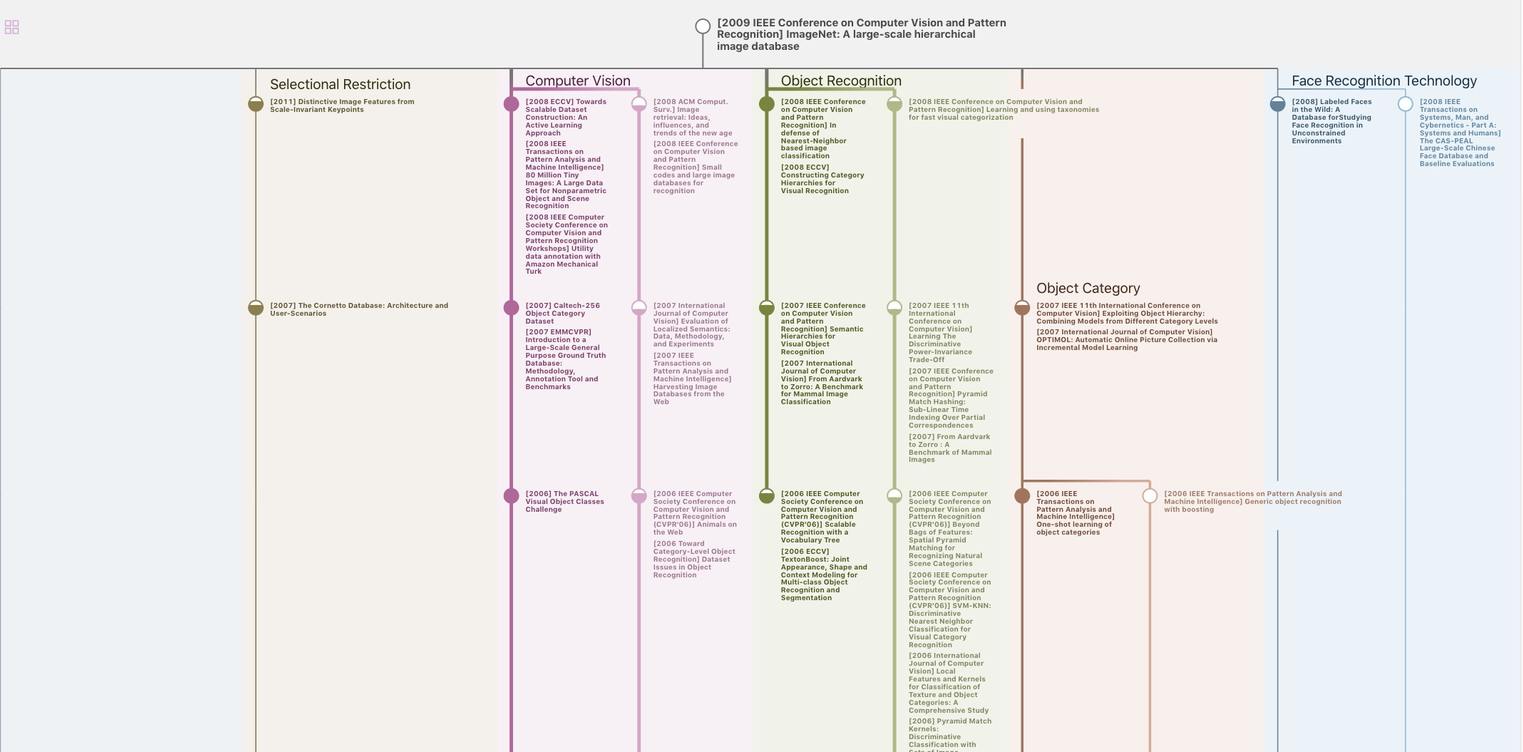
生成溯源树,研究论文发展脉络
Chat Paper
正在生成论文摘要