Multi-factor congressional vote prediction.
ASONAM '19: International Conference on Advances in Social Networks Analysis and Mining Vancouver British Columbia Canada August, 2019(2019)
摘要
In recent times we have seen a trend of having the ideologies of the two dominant political parties in the U.S. growing further and further apart. Simultaneously we have entered the age of big data raising enormous interest in computational approaches to solve problems in many domains such as political elections. However, an overlooked problem lies in predicting what happens once our elected officials take office, more specifically, predicting the congressional votes, which are perhaps the most influential decisions being made in the U.S. This, nevertheless, is far from a trivial task, since the congressional system is highly complex and heavily influenced by both ideological and social factors. Thus, dedicated efforts are required to first effectively identify and represent these factors, then furthermore capture the interactions between them. To this end, we proposed a robust end-to-end framework Multi-Factor Congressional Vote Prediction (MFCVP) that defines and encodes features from indicative ideological factors while also extracting novel social features. This allows for a principled expressive representation of the complex system, which ultimately leads to MFCVP making accurate vote predictions. Experimental results on a dataset from the U.S. House of Representatives shows the superiority of MFCVP to several representatives approaches when predicting votes for individual representatives and also the overall outcome of the bill voted on. Finally, we perform a factor analysis to understand the effectiveness and interplay between the different factors.
更多查看译文
关键词
congressional system,MFCVP,indicative ideological factors,social features,complex system,factor analysis,multifactor congressional vote prediction,dominant political parties,Big Data,political elections,elected officials,principled expressive representation
AI 理解论文
溯源树
样例
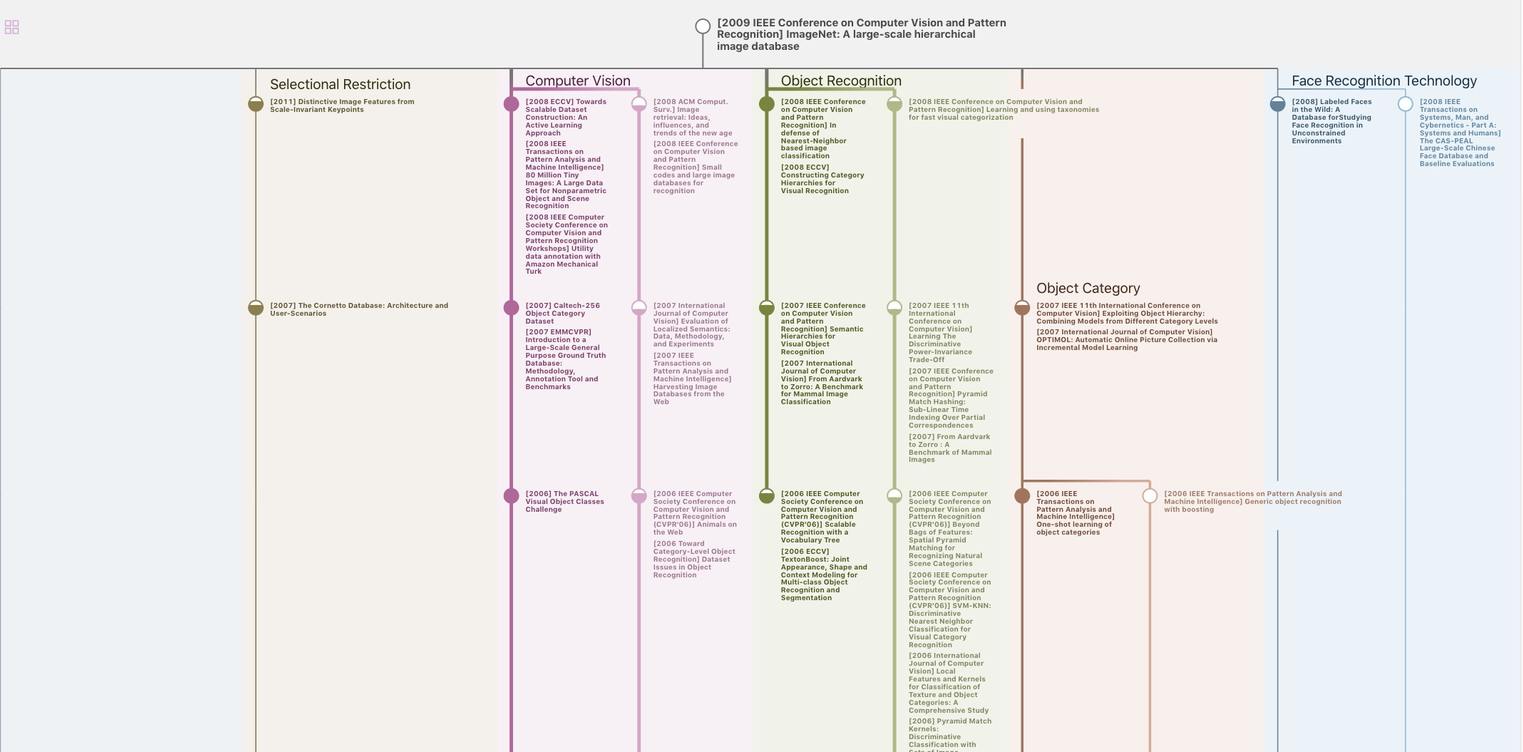
生成溯源树,研究论文发展脉络
Chat Paper
正在生成论文摘要