Fuzzy C-Means Based Clustering And Rule Formation Approach For Classification Of Bearing Faults Using Discrete Wavelet Transform
COMPUTATION(2019)
摘要
The rolling bearings are considered as the heart of rotating machinery and early fault diagnosis is one of the biggest challenges during operation. Due to complicated mechanical assemblies, detection of the advancing fault and faults at the incipient stage is very difficult and tedious. This work presents a fuzzy rule based classification of bearing faults using Fuzzy C-means clustering method using vibration measurements. Experiments were conducted to collect the vibration signals of a normal bearing and bearings with faults in the inner race, outer race and ball fault. Discrete Wavelet Transform (DWT) technique is used to decompose the vibration signals into different frequency bands. In order to detect the early faults in the bearings, various statistical features were extracted from this decomposed signal of each frequency band. Based on the extracted features, Fuzzy C-means clustering method (FCM) is developed to classify the faults using suitable membership functions and fuzzy rule base is developed for each class of the bearing fault using labeled data. The experimental results show that the proposed method is able to classify the condition of the bearing using the extracted features. The proposed FCM based clustering and classification model provides easier interpretation and implementation for monitoring the condition of the rolling bearings at an early stage and it will be helpful to take the preventive action before a large-scale failure.
更多查看译文
关键词
bearing fault diagnosis, discrete wavelet transform, clustering, feature extraction, Fuzzy C-means, fuzzy rule base
AI 理解论文
溯源树
样例
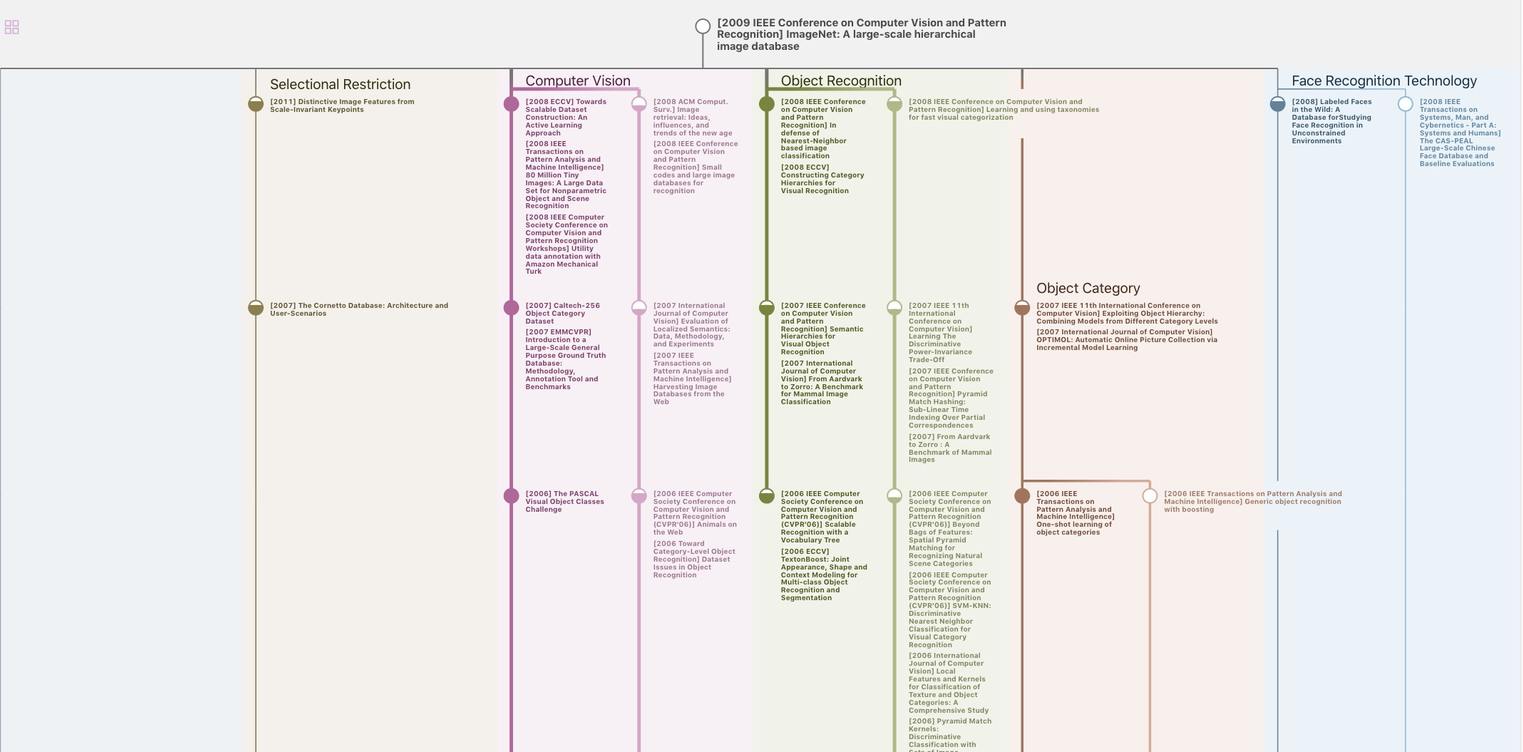
生成溯源树,研究论文发展脉络
Chat Paper
正在生成论文摘要